x = torch.cat([x1, x0], dim=1) x = self.concat_conv(x)
时间: 2023-11-16 11:06:52 浏览: 191
这是一个 PyTorch 的代码片段,该片段包含两行代码。第一行使用 `torch.cat()` 函数将两个张量 `x1` 和 `x0` 沿着第一维度进行拼接,生成一个新的张量 `x`。第二行代码将张量 `x` 作为输入传递给一个卷积层 `self.concat_conv` 进行卷积操作。具体来说,`torch.cat()` 函数用于在指定的维度上拼接张量,而卷积层是神经网络的一种常用层,用于提取输入张量的特征。
相关问题
def forward(self, x): x = self.downsample_conv(x) x0 = self.split_conv0(x) x1 = self.split_conv1(x) x1 = self.blocks_conv(x1) x = torch.cat([x1, x0], dim=1) x = self.concat_conv(x) return x这段代码的含义
这段代码定义了一个前向传播的函数,其中输入的参数 `x` 是一个张量,代表着网络模型的输入。在函数中,首先通过一个下采样卷积层 `downsample_conv` 对输入 `x` 进行下采样,将其尺寸减小。然后,将下采样后的特征张量 `x` 分别输入到两个卷积层 `split_conv0` 和 `split_conv1` 中,得到两个特征张量 `x0` 和 `x1`。接下来,对 `x1` 进行多次残差块计算,通过 `blocks_conv` 中的 Residual Block 层来提取和增强特征。然后,将 `x0` 和 `x1` 进行拼接,得到一个新的特征张量 `x`。最后,将 `x` 输入到合并卷积层 `concat_conv` 中,对特征进行融合和加工,最终得到网络模型的输出结果。整个函数的作用就是将输入张量通过网络模型的各个层进行前向传播计算,并返回最终的输出结果。
def forward(self, x1, x2): x1 = x1.to(torch.float32) x2 = x2.to(torch.float32) channel1_conv1 = self.channel1_conv1(x1).squeeze(dim=2) channel1_conv1 = torch.max(channel1_conv1, dim=1)[0].unsqueeze(dim=1) channel1_conv2 = self.channel1_conv2(x1).squeeze(dim=2) channel1_conv2 = torch.max(channel1_conv2, dim=1)[0].unsqueeze(dim=1) channel1_conv3 = self.channel1_conv3(x1).squeeze(dim=2) channel1_conv3 = torch.max(channel1_conv3, dim=1)[0].unsqueeze(dim=1) channel1_conv4 = self.channel1_conv4(x1).squeeze(dim=2) channel1_conv4 = torch.max(channel1_conv4, dim=1)[0].unsqueeze(dim=1) X1 = torch.cat([channel1_conv1, channel1_conv2, channel1_conv3, channel1_conv4], dim=1) channel2_conv1 = self.channel2_conv1(x2).squeeze(dim=2) channel2_conv1 = torch.max(channel2_conv1, dim=1)[0].unsqueeze(dim=1) channel2_conv2 = self.channel2_conv2(x2).squeeze(dim=2) channel2_conv2 = torch.max(channel2_conv2, dim=1)[0].unsqueeze(dim=1) channel2_conv3 = self.channel2_conv3(x2).squeeze(dim=2) channel2_conv3 = torch.max(channel2_conv3, dim=1)[0].unsqueeze(dim=1) channel2_conv4 = self.channel2_conv4(x2).squeeze(dim=2) channel2_conv4 = torch.max(channel2_conv4, dim=1)[0].unsqueeze(dim=1) X2 = torch.cat([channel2_conv1, channel2_conv2, channel2_conv3, channel2_conv4], dim=1) X2 = torch.cat([X1, X2], dim=1) output = self.layer3(X2)
这段代码是一个神经网络的前向传播函数,输入是两个张量 x1 和 x2,经过一系列卷积和池化操作后,最终输出一个张量 output。这个神经网络的具体结构是由一些卷积层、池化层和全连接层构成的,其中 channel1_conv1-4 和 channel2_conv1-4 是卷积层,layer3 是全连接层。这个神经网络的输入是两个通道的数据,通过卷积和池化操作后,将两个通道的结果拼接在一起,最后通过全连接层生成输出。
阅读全文
相关推荐
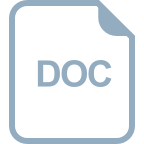
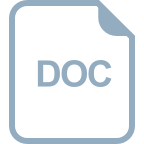
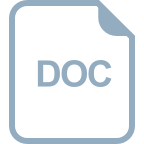













