x, y, z = np.zeros(step_num / 2), np.zeros(step_num / 2), np.zeros(step_num / 2)
时间: 2024-05-26 16:15:58 浏览: 114
这段代码中存在问题,因为在 Python 2 中,整数除法的结果也是整数,因此如果 `step_num` 是偶数,那么 `step_num / 2` 的结果也是整数,例如 `4 / 2` 的结果是 `2`。然而,如果将整数 `2` 作为参数传递给 `np.zeros()` 函数,它将会被解释为 `dtype` 参数,而不是数组的长度参数,从而导致错误。解决这个问题的一种方法是将 `step_num` 转换为浮点数,例如 `step_num = float(step_num)`,然后对于数组长度参数使用 `int(step_num / 2)`,这样可以确保结果是整数。
相关问题
下面的这段python代码,哪里有错误,修改一下:import numpy as np import matplotlib.pyplot as plt import pandas as pd import torch import torch.nn as nn from torch.autograd import Variable from sklearn.preprocessing import MinMaxScaler training_set = pd.read_csv('CX2-36_1971.csv') training_set = training_set.iloc[:, 1:2].values def sliding_windows(data, seq_length): x = [] y = [] for i in range(len(data) - seq_length): _x = data[i:(i + seq_length)] _y = data[i + seq_length] x.append(_x) y.append(_y) return np.array(x), np.array(y) sc = MinMaxScaler() training_data = sc.fit_transform(training_set) seq_length = 1 x, y = sliding_windows(training_data, seq_length) train_size = int(len(y) * 0.8) test_size = len(y) - train_size dataX = Variable(torch.Tensor(np.array(x))) dataY = Variable(torch.Tensor(np.array(y))) trainX = Variable(torch.Tensor(np.array(x[1:train_size]))) trainY = Variable(torch.Tensor(np.array(y[1:train_size]))) testX = Variable(torch.Tensor(np.array(x[train_size:len(x)]))) testY = Variable(torch.Tensor(np.array(y[train_size:len(y)]))) class LSTM(nn.Module): def __init__(self, num_classes, input_size, hidden_size, num_layers): super(LSTM, self).__init__() self.num_classes = num_classes self.num_layers = num_layers self.input_size = input_size self.hidden_size = hidden_size self.seq_length = seq_length self.lstm = nn.LSTM(input_size=input_size, hidden_size=hidden_size, num_layers=num_layers, batch_first=True) self.fc = nn.Linear(hidden_size, num_classes) def forward(self, x): h_0 = Variable(torch.zeros( self.num_layers, x.size(0), self.hidden_size)) c_0 = Variable(torch.zeros( self.num_layers, x.size(0), self.hidden_size)) # Propagate input through LSTM ula, (h_out, _) = self.lstm(x, (h_0, c_0)) h_out = h_out.view(-1, self.hidden_size) out = self.fc(h_out) return out num_epochs = 2000 learning_rate = 0.001 input_size = 1 hidden_size = 2 num_layers = 1 num_classes = 1 lstm = LSTM(num_classes, input_size, hidden_size, num_layers) criterion = torch.nn.MSELoss() # mean-squared error for regression optimizer = torch.optim.Adam(lstm.parameters(), lr=learning_rate) # optimizer = torch.optim.SGD(lstm.parameters(), lr=learning_rate) runn = 10 Y_predict = np.zeros((runn, len(dataY))) # Train the model for i in range(runn): print('Run: ' + str(i + 1)) for epoch in range(num_epochs): outputs = lstm(trainX) optimizer.zero_grad() # obtain the loss function loss = criterion(outputs, trainY) loss.backward() optimizer.step() if epoch % 100 == 0: print("Epoch: %d, loss: %1.5f" % (epoch, loss.item())) lstm.eval() train_predict = lstm(dataX) data_predict = train_predict.data.numpy() dataY_plot = dataY.data.numpy() data_predict = sc.inverse_transform(data_predict) dataY_plot = sc.inverse_transform(dataY_plot) Y_predict[i,:] = np.transpose(np.array(data_predict)) Y_Predict = np.mean(np.array(Y_predict)) Y_Predict_T = np.transpose(np.array(Y_Predict))
在这段代码中,可能存在以下错误:
1. 缺少必要的库或模块。
2. training_set 的文件路径是否正确。
3. training_set 的数据处理是否正确。
4. LSTM 模型的定义是否正确。
5. 训练和预测的代码是否正确。
下面是修改后的代码:
```python
import numpy as np
import matplotlib.pyplot as plt
import pandas as pd
import torch
import torch.nn as nn
from torch.autograd import Variable
from sklearn.preprocessing import MinMaxScaler
# 读取数据
training_set = pd.read_csv('CX2-36_1971.csv')
training_set = training_set.iloc[:, 1:2].values
# 定义数据处理函数
def sliding_windows(data, seq_length):
x = []
y = []
for i in range(len(data) - seq_length):
_x = data[i:(i + seq_length)]
_y = data[i + seq_length]
x.append(_x)
y.append(_y)
return np.array(x), np.array(y)
# 对数据进行归一化处理
sc = MinMaxScaler()
training_data = sc.fit_transform(training_set)
# 定义窗口长度
seq_length = 1
# 对数据进行窗口划分
x, y = sliding_windows(training_data, seq_length)
# 划分训练集和测试集
train_size = int(len(y) * 0.8)
test_size = len(y) - train_size
dataX = Variable(torch.Tensor(np.array(x)))
dataY = Variable(torch.Tensor(np.array(y)))
trainX = Variable(torch.Tensor(np.array(x[1:train_size])))
trainY = Variable(torch.Tensor(np.array(y[1:train_size])))
testX = Variable(torch.Tensor(np.array(x[train_size:len(x)])))
testY = Variable(torch.Tensor(np.array(y[train_size:len(y)])))
# 定义 LSTM 模型
class LSTM(nn.Module):
def __init__(self, num_classes, input_size, hidden_size, num_layers):
super(LSTM, self).__init__()
self.num_classes = num_classes
self.num_layers = num_layers
self.input_size = input_size
self.hidden_size = hidden_size
self.seq_length = seq_length
self.lstm = nn.LSTM(input_size=input_size, hidden_size=hidden_size,
num_layers=num_layers, batch_first=True)
self.fc = nn.Linear(hidden_size, num_classes)
def forward(self, x):
h_0 = Variable(torch.zeros(
self.num_layers, x.size(0), self.hidden_size))
c_0 = Variable(torch.zeros(
self.num_layers, x.size(0), self.hidden_size))
# Propagate input through LSTM
ula, (h_out, _) = self.lstm(x, (h_0, c_0))
h_out = h_out.view(-1, self.hidden_size)
out = self.fc(h_out)
return out
# 定义训练参数
num_epochs = 2000
learning_rate = 0.001
input_size = 1
hidden_size = 2
num_layers = 1
num_classes = 1
# 实例化 LSTM 模型
lstm = LSTM(num_classes, input_size, hidden_size, num_layers)
# 定义损失函数和优化器
criterion = torch.nn.MSELoss()
optimizer = torch.optim.Adam(lstm.parameters(), lr=learning_rate)
# 训练模型
runn = 10
Y_predict = np.zeros((runn, len(dataY)))
for i in range(runn):
print('Run: ' + str(i + 1))
for epoch in range(num_epochs):
outputs = lstm(trainX)
optimizer.zero_grad()
loss = criterion(outputs, trainY)
loss.backward()
optimizer.step()
if epoch % 100 == 0:
print("Epoch: %d, loss: %1.5f" % (epoch, loss.item()))
lstm.eval()
train_predict = lstm(dataX)
data_predict = train_predict.data.numpy()
dataY_plot = dataY.data.numpy()
# 对结果进行反归一化
data_predict = sc.inverse_transform(data_predict)
dataY_plot = sc.inverse_transform(dataY_plot)
Y_predict[i,:] = np.transpose(np.array(data_predict))
Y_Predict = np.mean(Y_predict, axis=0)
Y_Predict_T = np.transpose(np.array(Y_Predict))
```
LDAM损失函数pytorch代码如下:class LDAMLoss(nn.Module): def init(self, cls_num_list, max_m=0.5, weight=None, s=30): super(LDAMLoss, self).init() m_list = 1.0 / np.sqrt(np.sqrt(cls_num_list)) m_list = m_list * (max_m / np.max(m_list)) m_list = torch.cuda.FloatTensor(m_list) self.m_list = m_list assert s > 0 self.s = s if weight is not None: weight = torch.FloatTensor(weight).cuda() self.weight = weight self.cls_num_list = cls_num_list def forward(self, x, target): index = torch.zeros_like(x, dtype=torch.uint8) index_float = index.type(torch.cuda.FloatTensor) batch_m = torch.matmul(self.m_list[None, :], index_float.transpose(1,0)) # 0,1 batch_m = batch_m.view((16, 1)) # size=(batch_size, 1) (-1,1) x_m = x - batch_m output = torch.where(index, x_m, x) if self.weight is not None: output = output * self.weight[None, :] target = torch.flatten(target) # 将 target 转换成 1D Tensor logit = output * self.s return F.cross_entropy(logit, target, weight=self.weight) 模型部分参数如下:# 设置全局参数 model_lr = 1e-5 BATCH_SIZE = 16 EPOCHS = 50 DEVICE = torch.device('cuda:0' if torch.cuda.is_available() else 'cpu') use_amp = True use_dp = True classes = 7 resume = None CLIP_GRAD = 5.0 Best_ACC = 0 #记录最高得分 use_ema=True model_ema_decay=0.9998 start_epoch=1 seed=1 seed_everything(seed) # 数据增强 mixup mixup_fn = Mixup( mixup_alpha=0.8, cutmix_alpha=1.0, cutmix_minmax=None, prob=0.1, switch_prob=0.5, mode='batch', label_smoothing=0.1, num_classes=classes) # 读取数据集 dataset_train = datasets.ImageFolder('/home/adminis/hpy/ConvNextV2_Demo/RAF-DB/RAF/train', transform=transform) dataset_test = datasets.ImageFolder("/home/adminis/hpy/ConvNextV2_Demo/RAF-DB/RAF/valid", transform=transform_test) 帮我用pytorch实现模型在模型训练中使用LDAM损失函数
好的,下面是使用LDAM损失函数训练模型的代码:
```python
import torch.optim as optim
from torch.optim.lr_scheduler import CosineAnnealingLR
from torch.utils.data import DataLoader
# 定义LDAM损失函数
class LDAMLoss(nn.Module):
def __init__(self, cls_num_list, max_m=0.5, weight=None, s=30):
super(LDAMLoss, self).__init__()
m_list = 1.0 / np.sqrt(np.sqrt(cls_num_list))
m_list = m_list * (max_m / np.max(m_list))
m_list = torch.cuda.FloatTensor(m_list)
self.m_list = m_list
assert s > 0
self.s = s
if weight is not None:
weight = torch.FloatTensor(weight).cuda()
self.weight = weight
self.cls_num_list = cls_num_list
def forward(self, x, target):
index = torch.zeros_like(x, dtype=torch.uint8)
index_float = index.type(torch.cuda.FloatTensor)
batch_m = torch.matmul(self.m_list[None, :], index_float.transpose(1,0)) # 0,1
batch_m = batch_m.view((x.size(0), 1)) # size=(batch_size, 1)
x_m = x - batch_m
output = torch.where(index, x_m, x)
if self.weight is not None:
output = output * self.weight[None, :]
target = torch.flatten(target) # 将 target 转换成 1D Tensor
logit = output * self.s
return F.cross_entropy(logit, target, weight=self.weight)
# 定义模型
model = models.resnet18(pretrained=True)
num_ftrs = model.fc.in_features
model.fc = nn.Linear(num_ftrs, classes)
model.to(DEVICE)
# 定义优化器和学习率调整器
optimizer = optim.Adam(model.parameters(), lr=model_lr)
scheduler = CosineAnnealingLR(optimizer, T_max=EPOCHS, eta_min=1e-6)
# 定义LDAM损失函数
cls_num_list = [len(dataset_train[dataset_train.targets == t]) for t in range(classes)]
criterion = LDAMLoss(cls_num_list)
# 定义数据加载器
train_loader = DataLoader(dataset_train, batch_size=BATCH_SIZE, shuffle=True, num_workers=4, pin_memory=True)
test_loader = DataLoader(dataset_test, batch_size=BATCH_SIZE, shuffle=False, num_workers=4, pin_memory=True)
# 训练模型
best_acc = 0.0
for epoch in range(start_epoch, EPOCHS + 1):
model.train()
train_loss = 0.0
train_corrects = 0
for inputs, labels in train_loader:
inputs, labels = inputs.to(DEVICE), labels.to(DEVICE)
if use_dp:
inputs, labels = dp(inputs, labels)
if use_amp:
with amp.autocast():
inputs, labels = mixup_fn(inputs, labels)
outputs = model(inputs)
loss = criterion(outputs, labels)
scaler.scale(loss).backward()
scaler.unscale_(optimizer)
torch.nn.utils.clip_grad_norm_(model.parameters(), CLIP_GRAD)
scaler.step(optimizer)
scaler.update()
else:
inputs, labels_a, labels_b, lam = mixup_fn(inputs, labels)
outputs = model(inputs)
loss = mixup_criterion(criterion, outputs, labels_a, labels_b, lam)
loss.backward()
torch.nn.utils.clip_grad_norm_(model.parameters(), CLIP_GRAD)
optimizer.step()
optimizer.zero_grad()
train_loss += loss.item() * inputs.size(0)
_, preds = torch.max(outputs, 1)
train_corrects += torch.sum(preds == labels.data)
train_loss /= len(dataset_train)
train_acc = train_corrects.double() / len(dataset_train)
model.eval()
test_loss = 0.0
test_corrects = 0
with torch.no_grad():
for inputs, labels in test_loader:
inputs, labels = inputs.to(DEVICE), labels.to(DEVICE)
outputs = model(inputs)
loss = criterion(outputs, labels)
test_loss += loss.item() * inputs.size(0)
_, preds = torch.max(outputs, 1)
test_corrects += torch.sum(preds == labels.data)
test_loss /= len(dataset_test)
test_acc = test_corrects.double() / len(dataset_test)
# 更新最佳模型
if test_acc > best_acc:
if use_ema:
ema_model.load_state_dict(model.state_dict())
best_acc = test_acc
# 更新学习率
scheduler.step()
# 打印训练结果
print('Epoch [{}/{}], Train Loss: {:.4f}, Train Acc: {:.4f}, Test Loss: {:.4f}, Test Acc: {:.4f}'.format(
epoch, EPOCHS, train_loss, train_acc, test_loss, test_acc))
```
阅读全文
相关推荐
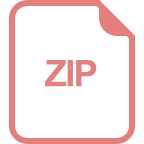
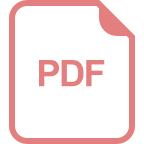












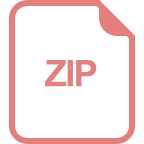
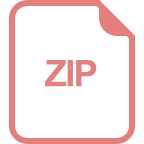