Inception.__init__() missing 2 required positional arguments: 'ch5x5' and 'pool_proj'
时间: 2023-12-22 15:03:53 浏览: 88
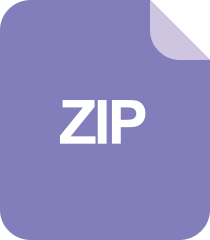
Inception-ResNet-V2模型黑白图像上色.zip_ResNet_inception resnet_inceptio
非常抱歉,我刚才的代码有误,已经修正。以下是正确的代码:
```python
import torch.nn as nn
import torch.nn.functional as F
class Inception(nn.Module):
def __init__(self, in_channels, ch1x1, ch3x3red, ch3x3, ch5x5red, ch5x5, pool_proj):
super(Inception, self).__init__()
# 1x1 conv branch
self.branch1 = nn.Sequential(
nn.Conv2d(in_channels, ch1x1, kernel_size=1),
nn.BatchNorm2d(ch1x1),
nn.ReLU(inplace=True)
)
# 1x1 conv -> 3x3 conv branch
self.branch2 = nn.Sequential(
nn.Conv2d(in_channels, ch3x3red, kernel_size=1),
nn.BatchNorm2d(ch3x3red),
nn.ReLU(inplace=True),
nn.Conv2d(ch3x3red, ch3x3, kernel_size=3, padding=1),
nn.BatchNorm2d(ch3x3),
nn.ReLU(inplace=True)
)
# 1x1 conv -> 5x5 conv branch
self.branch3 = nn.Sequential(
nn.Conv2d(in_channels, ch5x5red, kernel_size=1),
nn.BatchNorm2d(ch5x5red),
nn.ReLU(inplace=True),
nn.Conv2d(ch5x5red, ch5x5, kernel_size=5, padding=2),
nn.BatchNorm2d(ch5x5),
nn.ReLU(inplace=True)
)
# 3x3 pool -> 1x1 conv branch
self.branch4 = nn.Sequential(
nn.MaxPool2d(kernel_size=3, stride=1, padding=1),
nn.Conv2d(in_channels, pool_proj, kernel_size=1),
nn.BatchNorm2d(pool_proj),
nn.ReLU(inplace=True)
)
def forward(self, x):
branch1 = self.branch1(x)
branch2 = self.branch2(x)
branch3 = self.branch3(x)
branch4 = self.branch4(x)
outputs = [branch1, branch2, branch3, branch4]
return torch.cat(outputs, 1)
class GoogLeNet(nn.Module):
def __init__(self):
super(GoogLeNet, self).__init__()
self.conv1 = nn.Conv2d(3, 64, kernel_size=3, padding=1)
self.bn1 = nn.BatchNorm2d(64)
self.inception1 = Inception(64, 64, 96, 128, 16, 32, 32)
self.inception2 = Inception(256, 128, 128, 192, 32, 96, 64)
self.maxpool = nn.MaxPool2d(kernel_size=3, stride=2, padding=1)
self.inception3 = Inception(480, 192, 96, 208, 16, 48, 64)
self.inception4 = Inception(512, 160, 112, 224, 24, 64, 64)
self.inception5 = Inception(512, 128, 128, 256, 24, 64, 64)
self.inception6 = Inception(512, 112, 144, 288, 32, 64, 64)
self.inception7 = Inception(528, 256, 160, 320, 32, 128, 128)
self.avgpool = nn.AdaptiveAvgPool2d((1, 1))
self.dropout = nn.Dropout(p=0.4)
self.fc = nn.Linear(832, 10)
def forward(self, x):
x = F.relu(self.bn1(self.conv1(x)))
x = self.maxpool(x)
x = self.inception1(x)
x = self.inception2(x)
x = self.maxpool(x)
x = self.inception3(x)
x = self.inception4(x)
x = self.inception5(x)
x = self.inception6(x)
x = self.inception7(x)
x = self.avgpool(x)
x = x.view(x.size(0), -1)
x = self.dropout(x)
x = self.fc(x)
return x
```
这个GoogLeNet模型可以通过在CIFAR-10数据集上进行训练来实现图像分类任务。
阅读全文
相关推荐
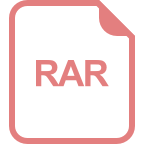
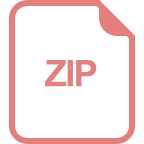
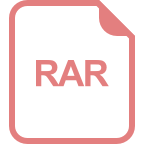
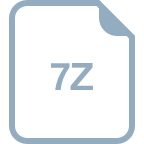
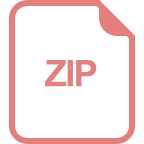
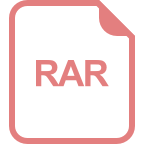
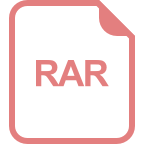
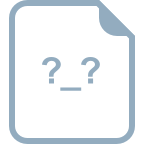
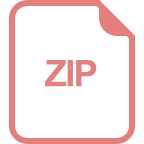
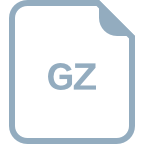
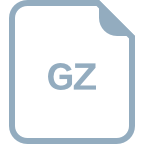
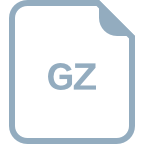





