class SelfAttention(nn.Module): def __init__(self, in_channels, reduction=4): super(SelfAttention, self).__init__() self.avg_pool = nn.AdaptiveAvgPool1d(1) # print("in_channels", in_channels) print("in_channels:", in_channels) print("reduction:", reduction) self.fc1 = nn.Conv1d(in_channels, in_channels // reduction, 1, bias=False) self.relu = nn.ReLU(inplace=True) self.fc2 = nn.Conv1d(in_channels // reduction, in_channels, 1, bias=False) self.sigmoid = nn.Sigmoid() def forward(self, x): b, c, n = x.size() print("x.shape=", x.shape) y = self.avg_pool(x) print("y.shape=", y.shape) # print("channel_out", channel_out) y = self.fc1(y) y = self.relu(y) y = self.fc2(y) y = self.sigmoid(y) return x * y.expand_as(x)我如果已将以上的SelfAttention模块加入到了PointRCNN中,及构建self.SA_modules.append( nn.Sequential( PointnetSAModuleMSG( npoint=cfg.RPN.SA_CONFIG.NPOINTS[k], radii=cfg.RPN.SA_CONFIG.RADIUS[k], nsamples=cfg.RPN.SA_CONFIG.NSAMPLE[k], mlps=mlps[:2], use_xyz=use_xyz, bn=cfg.RPN.USE_BN, ), SelfAttention(channel_out) ) ),在每一个SA模块后增加一层注意力机制模块,我想问的是SelfAttention中的forward函数什么时候被执行,是不是只有要最后输出全局特征时才执行forward函数,在构建网络时自动执行init函数
时间: 2023-11-20 07:05:21 浏览: 97
是的,SelfAttention类中的forward函数只有在网络执行前向传播时才会被调用。在你的代码中,当你构建网络时,会自动执行每个模块的init函数来初始化模块的参数。但是只有在执行网络的前向传播时,模块的forward函数才会被调用,因为在前向传播时需要计算模块的输出。所以只有当你调用模型的forward函数时,模块的forward函数才会被执行。在你的模型中,当你调用self.SA_modules的forward函数时,SelfAttention模块的forward函数才会被执行。
相关问题
SelfAttention(channel_out)该语句的作用是什么,我知道这个类的定义是:class SelfAttention(nn.Module): def __init__(self, in_channels, reduction=4): super(SelfAttention, self).__init__() self.avg_pool = nn.AdaptiveAvgPool1d(1) self.fc1 = nn.Conv1d(in_channels, in_channels // reduction, 1, bias=False) self.relu = nn.ReLU(inplace=True) self.fc2 = nn.Conv1d(in_channels // reduction, in_channels, 1, bias=False) self.sigmoid = nn.Sigmoid() def forward(self, x): b, c, n = x.size() y = self.avg_pool(x) y = self.fc1(y) y = self.relu(y) y = self.fc2(y) y = self.sigmoid(y) return x * y.expand_as(x)
这行代码的作用是用一个全连接层(fc1)来对输入的特征进行降维,然后通过ReLU激活函数进行非线性变换,再用另一个全连接层(fc2)将特征恢复到原来的维度。最后,通过Sigmoid函数将这个特征变换应用到原始特征向量上,以产生表示哪些部分应该被突出的向量,这个过程就是所谓的"自注意力"机制。因此,类SelfAttention的作用是实现一个自注意力机制,用于对输入数据进行特征提取和加权。
这是类的定义:class SelfAttention(nn.Module): def __init__(self, in_channels, reduction=4): super(SelfAttention, self).__init__() self.avg_pool = nn.AdaptiveAvgPool1d(1) self.fc1 = nn.Conv1d(in_channels, in_channels // reduction, 1, bias=False) self.relu = nn.ReLU(inplace=True) self.fc2 = nn.Conv1d(in_channels // reduction, in_channels, 1, bias=False) self.sigmoid = nn.Sigmoid() def forward(self, x): b, c, n = x.size() y = self.avg_pool(x) y = self.fc1(y) y = self.relu(y) y = self.fc2(y) y = self.sigmoid(y) return x * y.expand_as(x);而这是使用该类时的语句SelfAttention(channel_out),所以该语句是将channel_out传递给类中的in_channels吗?
是的,你的理解是正确的。在实例化`SelfAttention`类时,你需要传递一个`in_channels`参数,它将被用作输入张量`x`的通道数。在你的代码中,你使用`channel_out`来实例化`SelfAttention`类,因此`channel_out`将被传递给`in_channels`。在`forward`函数中,`x`表示输入张量,其大小为(b, c, n),其中b是批次大小,c是通道数,n是序列长度。
阅读全文
相关推荐
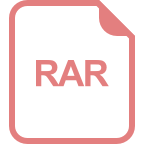
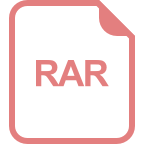
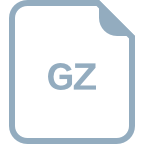









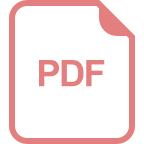
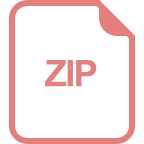
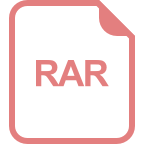
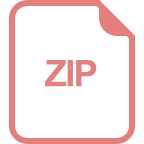
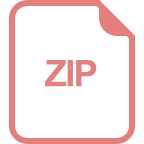