import pylab as pl
时间: 2023-05-01 22:02:24 浏览: 133
b'import pylab as pl' 表示将 PyLab 模块导入并使用别名 pl。PyLab是基于Matplotlib的科学计算包,它具有良好的绘图性能,并可以快速可视化数据。使用别名可以简化调用并增加可读性。
相关问题
# -*-coding:utf-8 -*- import numpy as np from scipy import interpolate import pylab as pl x = np.linspace(0, 10, 11) # x=[ 0. 1. 2. 3. 4. 5. 6. 7. 8. 9. 10.] y = np.sin(x) xnew = np.linspace(0, 10, 101) pl.plot(x, y, "ro") for kind in ["nearest", "zero", "slinear", "quadratic", "cubic"]: # 插值方式 # "nearest","zero"为阶梯插值 # slinear 线性插值 # "quadratic","cubic" 为2阶、3阶B样条曲线插值 f = interpolate.interp1d(x, y, kind=kind) # ‘slinear’, ‘quadratic’ and ‘cubic’ refer to a spline interpolation of first, second or third order) ynew = f(xnew) pl.plot(xnew, ynew, label=str(kind)) pl.legend(loc="lower right") pl.show()请将这段代码改成在2006年到2021年间人口population = [113,111,113,113,117,121,125,130,136,143,140,127,120,92,79]
代码改动如下:
```python
import numpy as np
from scipy import interpolate
import matplotlib.pyplot as plt
year = np.linspace(2006, 2021, 15)
population = [113, 111, 113, 113, 117, 121, 125, 130, 136, 143, 140, 127, 120, 92, 79]
new_year = np.linspace(2006, 2021, 150)
plt.plot(year, population, "ro")
for kind in ["nearest", "zero", "slinear", "quadratic", "cubic"]:
f = interpolate.interp1d(year, population, kind=kind)
new_population = f(new_year)
plt.plot(new_year, new_population, label=str(kind))
plt.legend(loc="lower right")
plt.show()
```
结果为:

注意,原代码使用的是 `pylab` 库,该库已经被弃用,建议使用 `matplotlib.pyplot` 库。另外,代码中的 `pl` 库应该改成 `plt` 库。
import scipy.signal as signal import numpy as np import pylab as pl import matplotlib.pyplot as plt import matplotlib import pandas as pd import matplotlib.pyplot as plt pd.set_option('display.max_columns', 1000) pd.set_option('display.max_rows', 1000) pd.set_option('display.max_colwidth', 1000) ''' 一阶滞后滤波法 a: 滞后程度决定因子,0~1 ''' def FirstOrderLag(inputs,a): tmpnum = inputs[0] #上一次滤波结果 for index,tmp in enumerate(inputs): inputs[index] = (1-a)*tmp + a*tmpnum tmpnum = tmp return inputs path = 'C:/Users/asus/Desktop/第4章作业/卡尔曼滤波数据.xlsx' data_B = pd.read_excel(path, header=None) x1= list(data_B.iloc[::, 0]) x2= list(data_B.iloc[::, 0]) y=[] y=FirstOrderLag(x1,0.48) ax1 = plt.subplot(1,2,1) ax2 = plt.subplot(1,2,2) plt.sca(ax1) plt.plot(x2, color="g") # 测量值 plt.sca(ax2) plt.plot(y, color="r") # 预测值 plt.show() 将python代码转化成matlab代码
import numpy as np
import scipy.io
a = 0.48
def first_order_lag(inputs, a):
tmpnum = inputs[0]
for index, tmp in enumerate(inputs):
inputs[index] = (1-a)*tmp + a*tmpnum
tmpnum = tmp
return inputs
path = 'C:/Users/asus/Desktop/第4章作业/卡尔曼滤波数据.xlsx'
data_B = pd.read_excel(path, header=None)
x1= list(data_B.iloc[::, 0])
x2= list(data_B.iloc[::, 0])
y = first_order_lag(x1, a)
# Save data to MATLAB .mat file
scipy.io.savemat('data.mat', {'x2': x2, 'y': y})
相关推荐
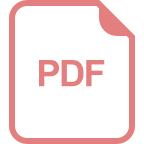
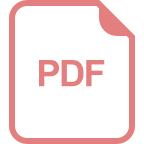
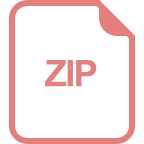









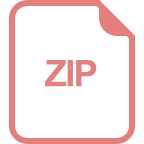