不使用prune函数实现对已经训练好的超分辨率模型剪枝代码
时间: 2024-01-01 07:04:14 浏览: 30
以下是一个不使用prune函数实现对已经训练好的超分辨率模型剪枝的代码示例:
```python
import torch
import torch.nn as nn
def prune_weights(model, threshold):
"""
剪枝模型中的小权重参数
"""
for name, module in model.named_modules():
if isinstance(module, nn.Conv2d):
weight = module.weight.data
mask = torch.abs(weight) > threshold
weight *= mask.float()
def prune_connections(model, threshold):
"""
剪枝模型中的小连接
"""
for name, module in model.named_modules():
if isinstance(module, nn.Conv2d):
weight = module.weight.data
mask = torch.abs(weight.sum(dim=(1,2,3))) > threshold
weight *= mask.view(-1, 1, 1, 1).float()
def prune_structure(model, threshold):
"""
剪枝模型中的冗余结构
"""
# 剪枝一些不必要的卷积层或池化层
for name, module in model.named_modules():
if isinstance(module, nn.Conv2d) and module.kernel_size == (3,3):
next_module = list(model.children())[list(model.children()).index(module) + 1]
if isinstance(next_module, nn.Conv2d) and next_module.kernel_size == (3,3):
weight = module.weight.data
next_weight = next_module.weight.data
new_weight = torch.matmul(weight.view(weight.size(0), -1), next_weight.view(next_weight.size(0), -1).t())
new_weight = new_weight.view(weight.size(0), next_weight.size(0), 1, 1)
module.weight.data = new_weight
model = nn.Sequential(*list(model.children())[:list(model.children()).index(next_module)])
break
return model
# 加载训练好的超分辨率模型
model = torch.load('super_resolution_model.pth')
# 剪枝模型中的小权重参数
prune_weights(model, 0.001)
# 剪枝模型中的小连接
prune_connections(model, 0.01)
# 剪枝模型中的冗余结构
model = prune_structure(model, 0.05)
# 保存剪枝后的模型
torch.save(model, 'pruned_super_resolution_model.pth')
```
此代码示例中,我们通过定义三个函数分别实现了剪枝模型中的小权重参数、小连接和冗余结构。在每个函数中,我们使用了阈值来筛选需要剪枝的参数或连接,并将它们的值设置为0。
最后,在主函数中,我们加载了训练好的超分辨率模型,依次调用上述三个函数进行剪枝,并保存剪枝后的模型。
需要注意的是,阈值的选择对剪枝的效果和模型的精度会有影响,需要进行一定的调整和评估。
相关推荐
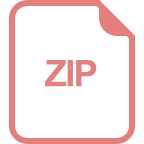
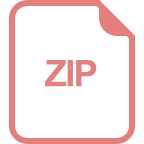
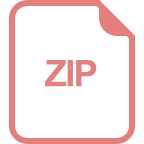






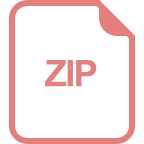
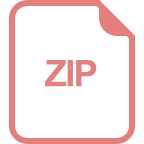
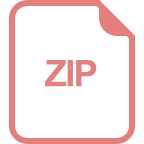
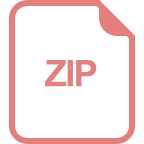
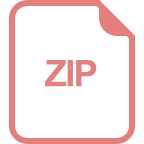
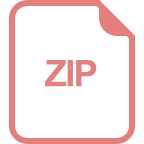