def dice_coeff(pred, target): smooth = 1. num = pred.size(0) m1 = pred.view(num, -1) # Flatten m2 = target.view(num, -1) # Flatten intersection = (m1 * m2).sum() return (2. * intersection + smooth) / (m1.sum() + m2.sum() + smooth)
时间: 2024-04-13 09:25:57 浏览: 8
这是一个用于计算 Dice Coefficient 的函数。Dice Coefficient 是一种常用于衡量目标分割模型性能的指标,它衡量预测结果与真实标签的相似程度。
函数接受两个参数 `pred` 和 `target`,分别代表模型的预测结果和真实标签。在函数内部,首先定义了一个平滑因子 `smooth`,用于避免除零错误。
接下来,函数计算了 `pred` 和 `target` 的形状,并将其展平为二维矩阵。然后,计算了两者的交集,并将结果相加。
最后,函数将交集乘以 2 并加上平滑因子,再除以 `pred` 和 `target` 的总和与平滑因子之和。这样就得到了 Dice Coefficient 的值,作为函数的返回值。
请注意,这段代码是用 Python 编写的,其中使用了 PyTorch 的张量操作。如果你有任何关于该函数的问题,请继续提问。
相关问题
def dice_coef_fun(smooth=1): def dice_coef(y_true, y_pred): #求得每个sample的每个类的dice intersection = K.sum(y_true * y_pred, axis=(1,2,3)) union = K.sum(y_true, axis=(1,2,3)) + K.sum(y_pred, axis=(1,2,3)) sample_dices=(2. * intersection + smooth) / (union + smooth) #一维数组 为各个类别的dice #求得每个类的dice dices=K.mean(sample_dices,axis=0) return K.mean(dices) #所有类别dice求平均的dice return dice_coef def dice_coef_loss_fun(smooth=0): def dice_coef_loss(y_true,y_pred): return 1-1-dice_coef_fun(smooth=smooth)(y_true=y_true,y_pred=y_pred) return dice_coef_loss
这段代码定义了两个函数,`dice_coef_fun` 和 `dice_coef_loss_fun`,用于计算 Dice Coefficient 和 Dice Coefficient Loss。
首先是 `dice_coef_fun` 函数,它接受一个平滑因子 `smooth` 的默认值为 1。在函数内部,首先计算了预测值 `y_pred` 和真实标签 `y_true` 的交集,并对其进行求和操作,轴为 (1,2,3)。然后,计算了真实标签和预测值的总和,并同样进行求和操作。最后,根据 Dice Coefficient 的公式,将交集乘以 2 并加上平滑因子,再除以总和与平滑因子之和,得到每个类别的 Dice Coefficient。最终,将所有类别的 Dice Coefficient 求平均,作为函数的返回值。
接下来是 `dice_coef_loss_fun` 函数,它接受一个平滑因子 `smooth` 的默认值为 0。在函数内部,调用了 `dice_coef_fun` 函数,并将 `y_true` 和 `y_pred` 作为参数传入。然后,将 `dice_coef_fun` 的返回值与 1 相减,并再次减去 1,得到 Dice Coefficient Loss 的值,作为函数的返回值。
这段代码使用了 Keras(或者 TensorFlow)的张量操作。如果你有关于这些函数的任何问题,请继续提问。
if cfg.deepsupervision: masks_preds = net(imgs) loss = 0 for masks_pred in masks_preds: tot_cross_entropy = 0 for true_mask, pred in zip(true_masks, masks_pred): pred = (pred > cfg.out_threshold).float() #二值化处理 if cfg.n_classes > 1: sub_cross_entropy = F.cross_entropy(pred.unsqueeze(dim=0), true_mask.unsqueeze(dim=0).squeeze(1)).item()#计算损失 else: sub_cross_entropy = dice_coeff(pred, true_mask.squeeze(dim=1)).item()#预测分割掩码和真实标签相似度,将两个结果转化为二值化的掩码,然后计算交集并集 tot_cross_entropy += sub_cross_entropy #计算总损失 tot_cross_entropy = tot_cross_entropy / len(masks_preds) #计算平均损失 tot += tot_cross_entropy #计算总平均损失 else: masks_pred = net(imgs) for true_mask, pred in zip(true_masks, masks_pred): pred = (pred > cfg.out_threshold).float() if cfg.n_classes > 1: tot += F.cross_entropy(pred.unsqueeze(dim=0), true_mask.unsqueeze(dim=0).squeeze(1)).item() else: tot += dice_coeff(pred, true_mask.squeeze(dim=1)).item() pbar.update(imgs.shape[0])
这段代码是语义分割任务中的损失计算部分,其中使用了深监督(Deep Supervision)的方法。在深监督中,一个模型通常会产生多个输出,每个输出都对应着不同的层或分支,在训练过程中,每个输出都会计算一次损失,并反向传播更新梯度。这个方法可以有效地加快模型的收敛速度,并提高模型的鲁棒性。
如果cfg.deepsupervision为True,表示使用深监督方法。代码首先使用网络net对输入图像imgs进行预测,得到多个预测掩码masks_pred。接下来,对于每一个预测掩码masks_pred,计算它与真实掩码true_masks之间的交叉熵损失或Dice系数损失,并将它们累加得到总的损失tot_cross_entropy。最后,将总的损失除以预测掩码的数量,得到平均损失tot_cross_entropy,并将它加到总平均损失tot中。
如果cfg.deepsupervision为False,表示不使用深监督方法。代码中仍然使用网络net对输入图像imgs进行预测,得到一个预测掩码masks_pred。接下来,计算它与真实掩码true_masks之间的交叉熵损失或Dice系数损失,并将它们累加得到总的损失tot。
最后,代码使用进度条pbar来显示训练的进度。其中pbar.update(imgs.shape[0])表示当前已经处理了多少张图片。
相关推荐
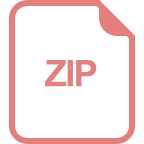
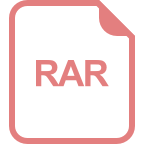
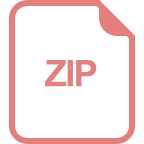












