self.c_fCASOTSUC = self.Cfg.getfloat('Calibration','fCASOTSUC')
时间: 2023-09-29 17:10:13 浏览: 61
这段代码是从配置文件中读取了一个浮点类型的参数,它属于一个名为"Calibration"的配置段(section),参数名为"fCASOTSUC"。通过调用`Cfg.getfloat`方法,可以将该参数的值读取到一个名为`c_fCASOTSUC`的实例变量中。假设配置文件中的内容如下:
```
[Calibration]
fCASOTSUC = 3.14
```
那么执行上述代码后,`c_fCASOTSUC`的值将为3.14。
相关问题
这是类的定义,class SelfAttention(nn.Module): def __init__(self, in_channels, reduction=4): super(SelfAttention, self).__init__() self.avg_pool = nn.AdaptiveAvgPool1d(1) self.fc1 = nn.Conv1d(in_channels, in_channels // reduction, 1, bias=False) self.relu = nn.ReLU(inplace=True) self.fc2 = nn.Conv1d(in_channels // reduction, in_channels, 1, bias=False) self.sigmoid = nn.Sigmoid() def forward(self, x): b, c, n = x.size() y = self.avg_pool(x) y = self.fc1(y) y = self.relu(y) y = self.fc2(y) y = self.sigmoid(y) return x * y.expand_as(x),而这是使用该类的位置:self.SA_modules.append( nn.Sequential( PointnetSAModuleMSG( npoint=cfg.RPN.SA_CONFIG.NPOINTS[k], radii=cfg.RPN.SA_CONFIG.RADIUS[k], nsamples=cfg.RPN.SA_CONFIG.NSAMPLE[k], mlps=mlps, use_xyz=use_xyz, bn=cfg.RPN.USE_BN, channel_out=channel_out ), SelfAttention(channel_out=channel_out) ) ),但是运行时报错:File "/root/autodl-tmp/project/tools/../lib/net/pointnet2_msg.py", line 125, in __init__ SelfAttention(channel_out=channel_out) TypeError: __init__() got an unexpected keyword argument 'channel_out',为什么呢?如何解决
在你的代码中,SelfAttention类的初始化方法中只接收两个参数in_channels和reduction,而你在使用该类时传递了一个名为channel_out的参数,导致了TypeError。
解决方法是修改SelfAttention类的定义,为其增加一个名为channel_out的参数,并在初始化方法中接收和使用该参数。修改后的类定义如下:
```
class SelfAttention(nn.Module):
def __init__(self, in_channels, channel_out, reduction=4):
super(SelfAttention, self).__init__()
self.avg_pool = nn.AdaptiveAvgPool1d(1)
self.fc1 = nn.Conv1d(in_channels, in_channels // reduction, 1, bias=False)
self.relu = nn.ReLU(inplace=True)
self.fc2 = nn.Conv1d(in_channels // reduction, channel_out, 1, bias=False)
self.sigmoid = nn.Sigmoid()
def forward(self, x):
b, c, n = x.size()
y = self.avg_pool(x)
y = self.fc1(y)
y = self.relu(y)
y = self.fc2(y)
y = self.sigmoid(y)
return x * y.expand_as(x)
```
然后在使用SelfAttention类时,需要传递in_channels和channel_out参数,例如:
```
self.SA_modules.append(
nn.Sequential(
PointnetSAModuleMSG(
npoint=cfg.RPN.SA_CONFIG.NPOINTS[k],
radii=cfg.RPN.SA_CONFIG.RADIUS[k],
nsamples=cfg.RPN.SA_CONFIG.NSAMPLE[k],
mlps=mlps,
use_xyz=use_xyz,
bn=cfg.RPN.USE_BN,
channel_out=channel_out
),
SelfAttention(in_channels=channel_out, channel_out=channel_out)
)
)
```
class SelfAttention(nn.Module): def __init__(self, in_channels, reduction=4): super(SelfAttention, self).__init__() self.avg_pool = nn.AdaptiveAvgPool1d(1) self.fc1 = nn.Conv1d(in_channels, in_channels // reduction, 1, bias=False) self.relu = nn.ReLU(inplace=True) self.fc2 = nn.Conv1d(in_channels // reduction, in_channels, 1, bias=False) self.sigmoid = nn.Sigmoid() def forward(self, x): b, c, n = x.size() y = self.avg_pool(x) y = self.fc1(y) y = self.relu(y) y = self.fc2(y) y = self.sigmoid(y) return x * y.expand_as(x) def get_model(input_channels=6, use_xyz=True): return Pointnet2MSG(input_channels=input_channels, use_xyz=use_xyz) class Pointnet2MSG(nn.Module): def __init__(self, input_channels=6, use_xyz=True): super().__init__() self.SA_modules = nn.ModuleList() channel_in = input_channels skip_channel_list = [input_channels] for k in range(cfg.RPN.SA_CONFIG.NPOINTS.len()): mlps = cfg.RPN.SA_CONFIG.MLPS[k].copy() channel_out = 0 for idx in range(mlps.len()): mlps[idx] = [channel_in] + mlps[idx] channel_out += mlps[idx][-1] mlps.append(channel_out) self.SA_modules.append( nn.Sequential( PointnetSAModuleMSG( npoint=cfg.RPN.SA_CONFIG.NPOINTS[k], radii=cfg.RPN.SA_CONFIG.RADIUS[k], nsamples=cfg.RPN.SA_CONFIG.NSAMPLE[k], mlps=mlps, use_xyz=use_xyz, bn=cfg.RPN.USE_BN ), SelfAttention(channel_out) ) ) skip_channel_list.append(channel_out) channel_in = channel_out self.FP_modules = nn.ModuleList() for k in range(cfg.RPN.FP_MLPS.len()): pre_channel = cfg.RPN.FP_MLPS[k + 1][-1] if k + 1 < len(cfg.RPN.FP_MLPS) else channel_out self.FP_modules.append( PointnetFPModule( mlp=[pre_channel + skip_channel_list[k]] + cfg.RPN.FP_MLPS[k] ) ) def _break_up_pc(self, pc): xyz = pc[..., 0:3].contiguous() features = ( pc[..., 3:].transpose(1, 2).contiguous() if pc.size(-1) > 3 else None ) return xyz, features def forward(self, pointcloud: torch.cuda.FloatTensor): xyz, features = self._break_up_pc(pointcloud) l_xyz, l_features = [xyz], [features] for i in range(len(self.SA_modules)): li_xyz, li_features = self.SA_modules[i](l_xyz[i], l_features[i]) l_xyz.append(li_xyz) l_features.append(li_features) for i in range(-1, -(len(self.FP_modules) + 1), -1): l_features[i - 1] = self.FP_modules[i]( l_xyz[i - 1], l_xyz[i], l_features[i - 1], l_features[i] ) return l_xyz[0], l_features[0]在forward函数中,如果我要使用channel_out变量传入SA_modules中,我该如何在forward函数中计算并得到它,再传入SA_modules中,你可以给我详细的代码吗?
在 `Pointnet2MSG` 的构造函数中,我们可以看到 SA_modules 是一个 `nn.ModuleList()`,其中每个元素都是一个 `nn.Sequential()`,包含了一个 `PointnetSAModuleMSG` 和一个 `SelfAttention`。
在 `PointnetSAModuleMSG` 中,我们可以看到最后一个 MLP 输出的通道数即为 `channel_out`。因此,我们可以在每个 `PointnetSAModuleMSG` 的定义中记录 `channel_out`,并将其传递给下一个 `PointnetSAModuleMSG` 的输入。
以下是修改后的代码:
```python
class Pointnet2MSG(nn.Module):
def __init__(self, input_channels=6, use_xyz=True):
super().__init__()
self.SA_modules = nn.ModuleList()
channel_in = input_channels
skip_channel_list = [input_channels]
for k in range(cfg.RPN.SA_CONFIG.NPOINTS.len()):
mlps = cfg.RPN.SA_CONFIG.MLPS[k].copy()
channel_out = 0
for idx in range(mlps.len()):
mlps[idx] = [channel_in] + mlps[idx]
channel_out += mlps[idx][-1]
mlps.append(channel_out)
sa_module = nn.Sequential(
PointnetSAModuleMSG(
npoint=cfg.RPN.SA_CONFIG.NPOINTS[k],
radii=cfg.RPN.SA_CONFIG.RADIUS[k],
nsamples=cfg.RPN.SA_CONFIG.NSAMPLE[k],
mlps=mlps,
use_xyz=use_xyz,
bn=cfg.RPN.USE_BN
)
)
self.SA_modules.append(sa_module)
skip_channel_list.append(channel_out)
channel_in = channel_out
self.FP_modules = nn.ModuleList()
for k in range(cfg.RPN.FP_MLPS.len()):
pre_channel = cfg.RPN.FP_MLPS[k + 1][-1] if k + 1 < len(cfg.RPN.FP_MLPS) else channel_out
self.FP_modules.append(
PointnetFPModule(
mlp=[pre_channel + skip_channel_list[k]] + cfg.RPN.FP_MLPS[k]
)
)
def _break_up_pc(self, pc):
xyz = pc[..., 0:3].contiguous()
features = (
pc[..., 3:].transpose(1, 2).contiguous()
if pc.size(-1) > 3 else None
)
return xyz, features
def forward(self, pointcloud: torch.cuda.FloatTensor):
xyz, features = self._break_up_pc(pointcloud)
l_xyz, l_features = [xyz], [features]
for i in range(len(self.SA_modules)):
sa_module = self.SA_modules[i]
li_xyz, li_features = sa_module(l_xyz[i], l_features[i])
l_xyz.append(li_xyz)
l_features.append(li_features)
channel_out = sa_module[-1].fc2.out_channels
for i in range(-1, -(len(self.FP_modules) + 1), -1):
l_features[i - 1] = self.FP_modules[i](
l_xyz[i - 1], l_xyz[i], l_features[i - 1], l_features[i]
)
return l_xyz[0], l_features[0], channel_out
```
使用上述代码,我们可以在 forward 函数中得到 `channel_out`,并将其传递给下一个 `PointnetSAModuleMSG` 的输入。
相关推荐
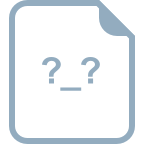
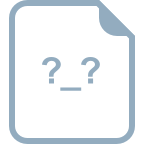








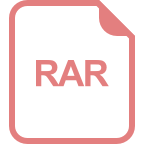