# 洗牌 def shuffleData(data): np.random.shuffle(data) cols = data.shape[1] X = data[:, 0:cols - 1] # 训练数据 y = data[:, cols - 1:] # 标签 return X, y,这段是逻辑回归方法中的一部分,请问为什么要有着一步洗牌操作
时间: 2023-12-06 16:43:01 浏览: 76
在机器学习中,数据的顺序对模型的训练和性能有影响。如果数据按照特定的顺序排列,那么模型可能会记住这个顺序而不是学习数据的真实特征。这会导致模型过拟合并在新的数据上表现不佳。
通过洗牌(shuffle)操作,我们可以打乱数据的顺序,使得每个样本的顺序都是随机的,这样模型就不能记住数据的顺序,而只能学习数据的真实特征。这样可以增加模型的泛化能力,使其在新的数据上表现更好。因此,在训练模型之前需要对数据进行洗牌操作。
相关问题
import pandas as pd import numpy as np from sklearn import preprocessing from sklearn.metrics import mean_squared_error, mean_absolute_error # 读取csv数据 data = pd.read_csv('3c_left_1-6.csv') # 将数据转化为numpy数组 data_np = np.array(data) # 获取数据的行数和列数 rows, cols = data_np.shape # 初始化滤波后数据的数组 data_filtered = np.zeros((rows, cols)) # 对每个方向的信号进行滤波处理 for i in range(cols): # 对信号进行归一化处理 data_normalized = preprocessing.scale(data_np[:, i]) # 定义滤波器大小 filter_size = 5 # 定义滤波器 filter = np.ones(filter_size) / filter_size # 使用卷积运算进行滤波 data_filtered[:, i] = np.convolve(data_normalized, filter, mode='same') # 计算SNR、MSE、PSNR指标 snr = 10 * np.log10(np.sum(data_np ** 2) / np.sum((data_np - data_filtered) ** 2)) mse = mean_squared_error(data_np, data_filtered) psnr = 10 * np.log10(255 ** 2 / mse) print("Signal-to-Noise Ratio (SNR): {:.2f} dB".format(snr)) print("Mean Squared Error (MSE): {:.2f}".format(mse)) print("Peak Signal-to-Noise Ratio (PSNR): {:.2f} dB".format(psnr)) data = {'SNR': [snr], 'MSE': [mse], 'PSNR': [psnr]} df = pd.DataFrame(data) df.to_csv('indicator_MAF.csv', index=False) # 将滤波后的数据保存到csv文件中 output = pd.DataFrame(data_filtered) output.to_csv('data_filtered.csv', index=False)如何提高去噪效果
以下是一些提高去噪效果的方法:
1. 使用更高级别的滤波器:可以使用更高级别的滤波器,如中值滤波器或小波变换滤波器,以更好地去除噪声,同时保留信号的细节。
2. 调整滤波器参数:可以尝试调整滤波器大小、滤波器类型等参数,以改善去噪效果。
3. 采用多种滤波器:可以尝试使用多种滤波器结合的方法,如串联、并联等方式,以获得更好的去噪效果。
4. 去除异常值:可以去除信号中的异常值,这些异常值可能会对滤波器的效果产生影响。
5. 增加信号采样率:可以增加信号的采样率,以提高信号的精度,从而更好地去除噪声。
6. 增加信号长度:可以增加信号的长度,以提高信号的稳定性和准确性,从而更好地去除噪声。
需要根据具体情况进行选择和调整。
import numpy as np import pandas as pd import matplotlib.pyplot as plt plt.rcParams['font.sans-serif'] = ["SimHei"] # 单使用会使负号显示错误 plt.rcParams['axes.unicode_minus'] = False # 把负号正常显示 # 读取北京房价数据 path = 'data.txt' data = pd.read_csv(path, header=None, names=['房子面积', '房子价格']) print(data.head(10)) print(data.describe()) # 绘制散点图 data.plot(kind='scatter', x='房子面积', y='房子价格') plt.show() def computeCost(X, y, theta): inner = np.power(((X * theta.T) - y), 2) return np.sum(inner) / (2 * len(X)) data.insert(0, 'Ones', 1) cols = data.shape[1] X = data.iloc[:,0:cols-1]#X是所有行,去掉最后一列 y = data.iloc[:,cols-1:cols]#X是所有行,最后一列 print(X.head()) print(y.head()) X = np.matrix(X.values) y = np.matrix(y.values) theta = np.matrix(np.array([0,0])) print(theta) print(X.shape, theta.shape, y.shape) def gradientDescent(X, y, theta, alpha, iters): temp = np.matrix(np.zeros(theta.shape)) parameters = int(theta.ravel().shape[1]) cost = np.zeros(iters) for i in range(iters): error = (X * theta.T) - y for j in range(parameters): term = np.multiply(error, X[:, j]) temp[0, j] = theta[0, j] - ((alpha / len(X)) * np.sum(term)) theta = temp cost[i] = computeCost(X, y, theta) return theta, cost alpha = 0.01 iters = 1000 g, cost = gradientDescent(X, y, theta, alpha, iters) print(g) print(computeCost(X, y, g)) x = np.linspace(data.Population.min(), data.Population.max(), 100) f = g[0, 0] + (g[0, 1] * x) fig, ax = plt.subplots(figsize=(12,8)) ax.plot(x, f, 'r', label='Prediction') ax.scatter(data.Population, data.Profit, label='Traning Data') ax.legend(loc=2) ax.set_xlabel('房子面积') ax.set_ylabel('房子价格') ax.set_title('北京房价拟合曲线图') plt.show()
这段代码中有几个错误需要修正:
1. 代码中导入库的语句需要加上换行符`\`,否则会提示语法错误:
```
import numpy as np
import pandas as pd
import matplotlib.pyplot as plt
plt.rcParams['font.sans-serif'] = ["SimHei"] # 单使用会使负号显示错误
plt.rcParams['axes.unicode_minus'] = False # 把负号正常显示
# 读取北京房价数据
path = 'data.txt'
data = pd.read_csv(path, header=None, names=['房子面积', '房子价格'])
print(data.head(10))
print(data.describe())
```
2. `X` 和 `y` 赋值时,需要把 `X` 和 `y` 同时修改,否则会导致后面的代码出错:
```
data.insert(0, 'Ones', 1)
cols = data.shape[1]
X = data.iloc[:,0:cols-1] # X是所有行,去掉最后一列
y = data.iloc[:,cols-1:cols] # y是所有行,最后一列
print(X.head())
print(y.head())
X = np.matrix(X.values)
y = np.matrix(y.values)
theta = np.matrix(np.array([0,0]))
print(theta)
print(X.shape, theta.shape, y.shape)
```
3. `gradientDescent` 函数中的代码需要修改,需要将 `temp` 数组的大小调整为 `(1, parameters)`,否则会导致后面的代码出错:
```
def gradientDescent(X, y, theta, alpha, iters):
temp = np.matrix(np.zeros((1, parameters))) # 修改这行代码
parameters = int(theta.ravel().shape[1])
cost = np.zeros(iters)
for i in range(iters):
error = (X * theta.T) - y
for j in range(parameters):
term = np.multiply(error, X[:, j])
temp[0, j] = theta[0, j] - ((alpha / len(X)) * np.sum(term))
theta = temp
cost[i] = computeCost(X, y, theta)
return theta, cost
```
4. 绘制拟合曲线的代码中使用了 `data.Population` 和 `data.Profit`,而这个数据集中没有这些列名,应该使用正确的列名 `房子面积` 和 `房子价格`:
```
x = np.linspace(data['房子面积'].min(), data['房子面积'].max(), 100)
f = g[0, 0] + (g[0, 1] * x)
fig, ax = plt.subplots(figsize=(12,8))
ax.plot(x, f, 'r', label='Prediction')
ax.scatter(data['房子面积'], data['房子价格'], label='Traning Data')
ax.legend(loc=2)
ax.set_xlabel('房子面积')
ax.set_ylabel('房子价格')
ax.set_title('北京房价拟合曲线图')
plt.show()
```
阅读全文
相关推荐
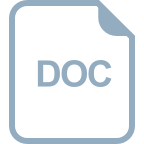
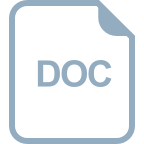
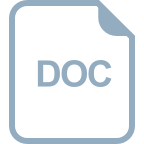














