def median_target(var): temp = data[data[var].notnull()] temp = temp[[var, 'Outcome']].groupby(['Outcome'])[[var]].median().reset_index() return temp data.loc[(data['Outcome'] == 0 ) & (data['Insulin'].isnull()), 'Insulin'] = 102.5 data.loc[(data['Outcome'] == 1 ) & (data['Insulin'].isnull()), 'Insulin'] = 169.5 data.loc[(data['Outcome'] == 0 ) & (data['Glucose'].isnull()), 'Glucose'] = 107 data.loc[(data['Outcome'] == 1 ) & (data['Glucose'].isnull()), 'Glucose'] = 1 data.loc[(data['Outcome'] == 0 ) & (data['SkinThickness'].isnull()), 'SkinThickness'] = 27 data.loc[(data['Outcome'] == 1 ) & (data['SkinThickness'].isnull()), 'SkinThickness'] = 32 data.loc[(data['Outcome'] == 0 ) & (data['BloodPressure'].isnull()), 'BloodPressure'] = 70 data.loc[(data['Outcome'] == 1 ) & (data['BloodPressure'].isnull()), 'BloodPressure'] = 74.5 data.loc[(data['Outcome'] == 0 ) & (data['BMI'].isnull()), 'BMI'] = 30.1 data.loc[(data['Outcome'] == 1 ) & (data['BMI'].isnull()), 'BMI'] = 34.3 target_col = ["Outcome"] cat_cols = data.nunique()[data.nunique() < 12].keys().tolist() cat_cols = [x for x in cat_cols ] #numerical columns num_cols = [x for x in data.columns if x not in cat_cols + target_col] #Binary columns with 2 values bin_cols = data.nunique()[data.nunique() == 2].keys().tolist() #Columns more than 2 values multi_cols = [i for i in cat_cols if i not in bin_cols] #Label encoding Binary columns le = LabelEncoder() for i in bin_cols : data[i] = le.fit_transform(data[i]) #Duplicating columns for multi value columns data = pd.get_dummies(data = data,columns = multi_cols ) #Scaling Numerical columns std = StandardScaler() scaled = std.fit_transform(data[num_cols]) scaled = pd.DataFrame(scaled,columns=num_cols) #dropping original values merging scaled values for numerical columns df_data_og = data.copy() data = data.drop(columns = num_cols,axis = 1) data = data.merge(scaled,left_index=True,right_index=True,how = "left") # Def X and Y X = data.drop('Outcome', axis=1) y = data['Outcome'] X_train, X_test, y_train, y_test = train_test_split(X, y, train_size=0.8, shuffle=True, random_state=1) y_train = to_categorical(y_train) y_test = to_categorical(y_test)
时间: 2024-01-14 07:02:33 浏览: 84
这段代码看起来是在进行数据预处理,首先定义了一个函数`median_target`,用于计算每个类别中某个特征的中位数。然后根据不同的`Outcome`值,对缺失值进行填充。接着将数据集中的分类特征进行编码,将二元特征进行二元编码,将多元特征进行独热编码。最后,对数值特征进行标准化处理,并将处理后的数据集进行拆分为训练集和测试集。
相关问题
def median_target(var): temp = data[data[var].notnull()] temp = temp[[var, 'Outcome']].groupby(['Outcome'])[[var]].median().reset_index() return temp
这是一个 Python 函数,用于计算指定变量在不同分类情况下的中位数。
函数名为 median_target,接收一个参数 var,表示要计算中位数的变量名。
函数中,首先使用 data[data[var].notnull()] 过滤掉 var 变量为空的行,然后使用 [[var, 'Outcome']] 选择 var 变量和分类变量 Outcome 两列。接着使用 groupby(['Outcome'])[[var]].median().reset_index() 对数据进行分组计算,分组依据为 Outcome 变量,计算的统计量为 var 变量的中位数。最后返回计算结果。
该函数可能是用于数据分析和特征工程中,用于计算不同分类情况下某个变量的中位数,以帮助挖掘变量与分类变量之间的关系。
function median_target(var) { temp = data[data[var].notnull()]; temp = temp[[var, 'Outcome']].groupby(['Outcome'])[[var]].median().reset_index(); return temp; } data.loc[(data['Outcome'] == 0) & (data['Insulin'].isnull()), 'Insulin'] = 102.5; data.loc[(data['Outcome'] == 1) & (data['Insulin'].isnull()), 'Insulin'] = 169.5; data.loc[(data['Outcome'] == 0) & (data['Glucose'].isnull()), 'Glucose'] = 107; data.loc[(data['Outcome'] == 1) & (data['Glucose'].isnull()), 'Glucose'] = 1; data.loc[(data['Outcome'] == 0) & (data['SkinThickness'].isnull()), 'SkinThickness'] = 27; data.loc[(data['Outcome'] == 1) & (data['SkinThickness'].isnull()), 'SkinThickness'] = 32; data.loc[(data['Outcome'] == 0) & (data['BloodPressure'].isnull()), 'BloodPressure'] = 70; data.loc[(data['Outcome'] == 1) & (data['BloodPressure'].isnull()), 'BloodPressure'] = 74.5; data.loc[(data['Outcome'] == 0) & (data['BMI'].isnull()), 'BMI'] = 30.1; data.loc[(data['Outcome'] == 1) & (data['BMI'].isnull()), 'BMI'] = 34.3; target_col = ["Outcome"]; cat_cols = data.nunique()[data.nunique() < 12].keys().tolist(); cat_cols = [x for x in cat_cols]; num_cols = [x for x in data.columns if x not in cat_cols + target_col]; bin_cols = data.nunique()[data.nunique() == 2].keys().tolist(); multi_cols = [i for i in cat_cols if i in bin_cols]; le = LabelEncoder(); for i in bin_cols: data[i] = le.fit_transform(data[i]); data = pd.get_dummies(data=data, columns=multi_cols); std = StandardScaler(); scaled = std.fit_transform(data[num_cols]); scaled = pd.DataFrame(scaled, columns=num_cols); df_data_og = data.copy(); data = data.drop(columns=num_cols, axis=1); data = data.merge(scaled, left_index=True, right_index=True, how='left'); X = data.drop('Outcome', axis=1); y = data['Outcome']; X_train, X_test, y_train, y_test = train_test_split(X, y, train_size=0.8, shuffle=True, random_state=1); y_train = to_categorical(y_train); y_test = to_categorical(y_test);将这段代码添加注释
# 导入必要的库
import pandas as pd
from sklearn.preprocessing import LabelEncoder, StandardScaler
from keras.utils import to_categorical
from sklearn.model_selection import train_test_split
# 定义函数,返回每个特征在不同结果下的中位数
def median_target(var):
temp = data[data[var].notnull()]
temp = temp[[var, 'Outcome']].groupby(['Outcome'])[[var]].median().reset_index()
return temp
# 将缺失值填充为中位数
data.loc[(data['Outcome'] == 0) & (data['Insulin'].isnull()), 'Insulin'] = 102.5
data.loc[(data['Outcome'] == 1) & (data['Insulin'].isnull()), 'Insulin'] = 169.5
data.loc[(data['Outcome'] == 0) & (data['Glucose'].isnull()), 'Glucose'] = 107
data.loc[(data['Outcome'] == 1) & (data['Glucose'].isnull()), 'Glucose'] = 1
data.loc[(data['Outcome'] == 0) & (data['SkinThickness'].isnull()), 'SkinThickness'] = 27
data.loc[(data['Outcome'] == 1) & (data['SkinThickness'].isnull()), 'SkinThickness'] = 32
data.loc[(data['Outcome'] == 0) & (data['BloodPressure'].isnull()), 'BloodPressure'] = 70
data.loc[(data['Outcome'] == 1) & (data['BloodPressure'].isnull()), 'BloodPressure'] = 74.5
data.loc[(data['Outcome'] == 0) & (data['BMI'].isnull()), 'BMI'] = 30.1
data.loc[(data['Outcome'] == 1) & (data['BMI'].isnull()), 'BMI'] = 34.3
# 将数据进行分类处理
target_col = ["Outcome"]
cat_cols = data.nunique()[data.nunique() < 12].keys().tolist()
cat_cols = [x for x in cat_cols]
num_cols = [x for x in data.columns if x not in cat_cols + target_col]
bin_cols = data.nunique()[data.nunique() == 2].keys().tolist()
multi_cols = [i for i in cat_cols if i in bin_cols]
# 对二分类特征进行编码
le = LabelEncoder()
for i in bin_cols:
data[i] = le.fit_transform(data[i])
# 将分类特征进行独热编码
data = pd.get_dummies(data=data, columns=multi_cols)
# 对数值特征进行标准化
std = StandardScaler()
scaled = std.fit_transform(data[num_cols])
scaled = pd.DataFrame(scaled, columns=num_cols)
# 将数据进行合并
df_data_og = data.copy()
data = data.drop(columns=num_cols, axis=1)
data = data.merge(scaled, left_index=True, right_index=True, how='left')
# 划分训练集和测试集,对标签进行独热编码
X = data.drop('Outcome', axis=1)
y = data['Outcome']
X_train, X_test, y_train, y_test = train_test_split(X, y, train_size=0.8, shuffle=True, random_state=1)
y_train = to_categorical(y_train)
y_test = to_categorical(y_test)
阅读全文
相关推荐
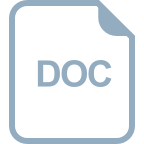
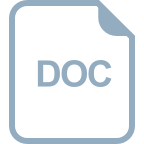
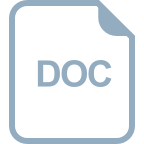

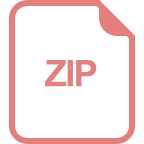


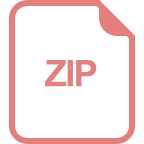
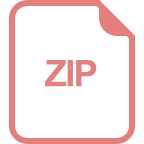
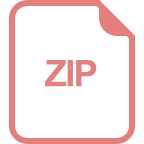
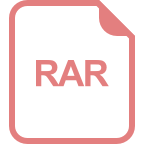
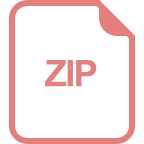
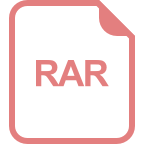
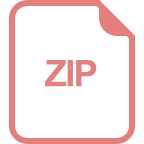
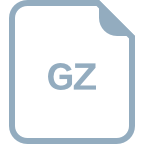
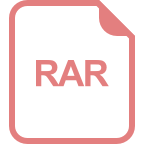
