# Style loss class GramMatrix(nn.Module): def forward(self, input): a, b, c, d = input.size() # a=batch size(=1) # b=number of feature maps # (c,d)=dimensions of a f. map (N=c*d) features = input.view(a * b, c * d) # resise F_XL into \hat F_XL G = torch.mm(features, features.t()) # compute the gram product # we 'normalize' the values of the gram matrix # by dividing by the number of element in each feature maps. return G.div(a * b * c * d) class StyleLoss(nn.Module): def __init__(self, target, weight): super(StyleLoss, self).__init__() self.target = target.detach() * weight self.weight = weight self.gram = GramMatrix() self.criterion = nn.MSELoss() def forward(self, input): self.output = input.clone() self.G = self.gram(input) self.G.mul_(self.weight) self.loss = self.criterion(self.G, self.target) return self.output def backward(self, retain_graph=True): self.loss.backward(retain_graph=retain_graph) return self.loss
时间: 2023-12-09 16:06:11 浏览: 142
这段代码是用于计算风格损失的。其中,GramMatrix类用于计算输入的Gram矩阵,即特征图的协方差矩阵,以表达输入的风格信息;StyleLoss类则用于计算输入与目标风格之间的均方误差,作为风格损失。
在forward方法中,输入被克隆为输出,并使用GramMatrix计算出输入的Gram矩阵,再乘以权重,最后计算均方误差得到风格损失。在backward方法中,反向传播损失,并返回损失值。
相关问题
class GramMatrix(nn.Module): def forward(self, input): a, b, c, d = input.size() # a=batch size(=1) # b=number of feature maps # (c,d)=dimensions of a f. map (N=c*d) features = input.view(a * b, c * d) # resise F_XL into \hat F_XL G = torch.mm(features, features.t()) # compute the gram product # we 'normalize' the values of the gram matrix # by dividing by the number of element in each feature maps. return G.div(a * b * c * d) class StyleLoss(nn.Module): def __init__(self, target, weight): super(StyleLoss, self).__init__() self.target = target.detach() * weight self.weight = weight self.gram = GramMatrix() self.criterion = nn.MSELoss() def forward(self, input): self.output = input.clone() self.G = self.gram(input) self.G.mul_(self.weight) self.loss = self.criterion(self.G, self.target) return self.output def backward(self, retain_graph=True): self.loss.backward(retain_graph=retain_graph) return self.loss
这段代码实现了风格损失的计算。其中GramMatrix模块用来计算输入的特征图的Gram矩阵,StyleLoss模块则用来计算输入图像与目标图像在风格上的差异。具体实现中,输入图像通过GramMatrix模块计算出它的Gram矩阵,然后与目标图像的Gram矩阵计算出它们之间的MSE损失。这个MSE损失就是风格损失,用于衡量输入图像和目标图像在风格上的相似程度。在反向传播时,通过调用backward函数来计算梯度。
class ContentLoss(nn.Module): def __init__(self, target, weight): super(ContentLoss, self).__init__() # we 'detach' the target content from the tree used self.target = target.detach() * weight # to dynamically compute the gradient: this is a stated value, # not a variable. Otherwise the forward method of the criterion # will throw an error. self.weight = weight self.criterion = nn.MSELoss() def forward(self, input): self.loss = self.criterion(input * self.weight, self.target) self.output = input return self.output def backward(self, retain_graph=True): self.loss.backward(retain_graph=retain_graph) return self.loss
这是一个用于计算内容损失的 PyTorch 模块。它包括两个主要方法:forward 和 backward。forward 方法计算输入张量 input 与目标张量 target 之间的均方误差损失,并将其乘以权重 weight。backward 方法计算损失关于输入张量的梯度,并返回损失值。这个模块通常用于图像生成领域中的风格转移任务,其中需要最小化输入图像与目标图像之间的内容差异。
阅读全文
相关推荐
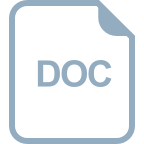
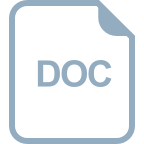
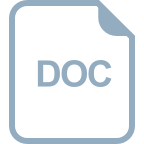













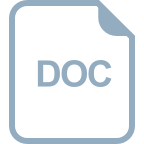