class ContentLoss(nn.Module): def __init__(self, target, weight): super(ContentLoss, self).__init__() # we 'detach' the target content from the tree used self.target = target.detach() * weight # to dynamically compute the gradient: this is a stated value, # not a variable. Otherwise the forward method of the criterion # will throw an error. self.weight = weight self.criterion = nn.MSELoss() def forward(self, input): self.loss = self.criterion(input * self.weight, self.target) self.output = input return self.output def backward(self, retain_graph=True): self.loss.backward(retain_graph=retain_graph) return self.loss
时间: 2023-12-25 22:05:26 浏览: 35
这段代码是PyTorch中一个自定义的损失函数模块。它继承了nn.Module类,因此可以像其他标准的神经网络层一样在模型中使用。
该损失函数的作用是衡量输入图片与目标图片之间的内容差异,即MSE(均方误差)。在初始化时,它会将目标图片与权重值相乘,用于动态计算梯度。在前向传播时,它计算输入图片与目标图片之间的MSE损失,并将输入图片作为输出返回。在反向传播时,它通过调用backward()方法来计算梯度并返回损失。retain_graph参数表示是否在计算梯度之后保留计算图,以便进行多次反向传播。
这个模块通常用于风格迁移的损失函数中,其中目标图片是所需的风格图片,而输入图片是待转换的内容图片。
相关问题
Focal loss代码如下:class FocalLoss(nn.Module): def __init__(self, alpha=1, gamma=2, weight=None): super(FocalLoss, self).__init__() self.alpha = alpha self.gamma = gamma def forward(self, x: torch.Tensor, target: torch.Tensor) -> torch.Tensor: log_probs = F.log_softmax(x, dim=-1) probs = torch.exp(log_probs) focal_loss = -self.alpha * (torch.pow((1 - probs), self.gamma)) * log_probs loss = torch.sum(target * focal_loss, dim=-1) return loss.mean() LDAM loss 代码如下:class LDAMLoss(nn.Module): def __init__(self, cls_num_list, max_m=0.5, weight=None, s=30): super(LDAMLoss, self).__init__() m_list = 1.0 / np.sqrt(np.sqrt(cls_num_list)) m_list = m_list * (max_m / np.max(m_list)) m_list = torch.cuda.FloatTensor(m_list) self.m_list = m_list assert s > 0 self.s = s self.weight = weight def forward(self, x, target): index = torch.zeros_like(x, dtype=torch.uint8) index.scatter_(1, target.data.view(-1, 1), 1) index_float = index.type(torch.cuda.FloatTensor) batch_m = torch.matmul(self.m_list[None, :], index_float.transpose(0,1)) batch_m = batch_m.view((-1, 1)) x_m = x - batch_m output = torch.where(index, x_m, x) return F.cross_entropy(self.s*output, target, weight=self.weight) LMF loss 是Focal loss 和LDAM loss两个损失函数的加权求和,请用pytorch代码实现LMF损失函数
下面是LMF损失函数的PyTorch代码实现,其中alpha和beta是Focal loss和LDAM loss的权重,需要根据具体情况进行调整:
```
class LMF(nn.Module):
def __init__(self, cls_num_list, max_m=0.5, weight=None, s=30, alpha=0.25, gamma=2, beta=0.5):
super(LMF, self).__init__()
self.focal_loss = FocalLoss(alpha=alpha, gamma=gamma, weight=weight)
self.ldam_loss = LDAMLoss(cls_num_list, max_m=max_m, weight=weight, s=s)
self.alpha = alpha
self.beta = beta
def forward(self, x, target):
focal_loss = self.focal_loss(x, target)
ldam_loss = self.ldam_loss(x, target)
loss = self.alpha * focal_loss + self.beta * ldam_loss
return loss
```
其中,输入参数x是模型的输出,target是真实标签。cls_num_list是每个类别的样本数列表,max_m是LDAM loss中的一个参数,weight是交叉熵损失函数中的权重,s是LDAM loss中的一个参数,alpha和beta是Focal loss和LDAM loss的权重。最终返回LMF损失函数的值。
Focal 损失函数代码如下:def focal_loss(input_values, gamma): """Computes the focal loss""" p = torch.exp(-input_values) loss = (1 - p) ** gamma * input_values return loss.mean() class FocalLoss(nn.Module): def __init__(self, weight=None, gamma=0.): super(FocalLoss, self).__init__() assert gamma >= 0 self.gamma = gamma self.weight = weight def forward(self, input, target): return focal_loss(F.cross_entropy(input, target, reduction='none', weight=self.weight), self.gamma) LDAM损失函数代码如下:class LDAMLoss(nn.Module): def __init__(self, cls_num_list, max_m=0.5, weight=None, s=30): super(LDAMLoss, self).__init__() m_list = 1.0 / np.sqrt(np.sqrt(cls_num_list)) m_list = m_list * (max_m / np.max(m_list)) m_list = torch.cuda.FloatTensor(m_list) self.m_list = m_list assert s > 0 self.s = s self.weight = weight def forward(self, x, target): index = torch.zeros_like(x, dtype=torch.uint8) index.scatter_(1, target.data.view(-1, 1), 1) index_float = index.type(torch.cuda.FloatTensor) batch_m = torch.matmul(self.m_list[None, :], index_float.transpose(0,1)) batch_m = batch_m.view((-1, 1)) x_m = x - batch_m output = torch.where(index, x_m, x) return F.cross_entropy(self.s*output, target, weight=self.weight) Large Margin aware Focal (LMF) 损失函数是 Focal 损失函数和 LDAM损失函数加权的线性组合,帮我用pytorch代码实现LMF损失函数
以下是LMF损失函数的PyTorch实现:
```
class LMF_Loss(nn.Module):
def __init__(self, cls_num_list, max_m=0.5, weight=None, s=30, gamma=2.0, alpha=0.25):
super(LMF_Loss, self).__init__()
self.gamma = gamma
self.alpha = alpha
self.focal_loss = FocalLoss(weight=weight, gamma=gamma)
self.ldam_loss = LDAMLoss(cls_num_list, max_m=max_m, weight=weight, s=s)
def forward(self, x, target):
focal_loss = self.focal_loss(x, target)
ldam_loss = self.ldam_loss(x, target)
lmf_loss = self.alpha * focal_loss + (1 - self.alpha) * ldam_loss.pow(self.gamma)
return lmf_loss.mean()
```
其中,`gamma`为Focal Loss的超参数,`alpha`为LMF Loss中Focal Loss的权重。其他参数含义与原始的Focal Loss和LDAM Loss相同。
相关推荐
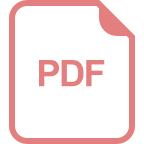
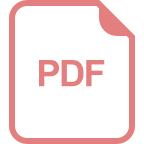
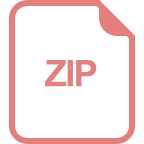











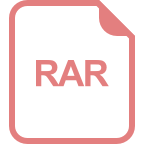
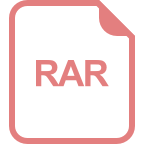