class GramMatrix(nn.Module): def forward(self, input): a, b, c, d = input.size() # a=batch size(=1) # b=number of feature maps # (c,d)=dimensions of a f. map (N=c*d) features = input.view(a * b, c * d) # resise F_XL into \hat F_XL G = torch.mm(features, features.t()) # compute the gram product # we 'normalize' the values of the gram matrix # by dividing by the number of element in each feature maps. return G.div(a * b * c * d) class StyleLoss(nn.Module): def __init__(self, target, weight): super(StyleLoss, self).__init__() self.target = target.detach() * weight self.weight = weight self.gram = GramMatrix() self.criterion = nn.MSELoss() def forward(self, input): self.output = input.clone() self.G = self.gram(input) self.G.mul_(self.weight) self.loss = self.criterion(self.G, self.target) return self.output def backward(self, retain_graph=True): self.loss.backward(retain_graph=retain_graph) return self.loss
时间: 2023-12-09 07:06:11 浏览: 40
这段代码实现了风格损失的计算。其中GramMatrix模块用来计算输入的特征图的Gram矩阵,StyleLoss模块则用来计算输入图像与目标图像在风格上的差异。具体实现中,输入图像通过GramMatrix模块计算出它的Gram矩阵,然后与目标图像的Gram矩阵计算出它们之间的MSE损失。这个MSE损失就是风格损失,用于衡量输入图像和目标图像在风格上的相似程度。在反向传播时,通过调用backward函数来计算梯度。
相关问题
解释一下这个代码:class Flatten(nn.Module): def forward(self, input): return input.view(input.size(0),-1)
这段代码定义了一个名为Flatten的类,它是nn.Module的子类,即一个PyTorch模型的基本组成部分。该类的作用是将输入张量展平成一维,以便于传递给后续的全连接层等模块。
具体实现是在forward函数中,输入参数input是一个张量,通过张量的view方法将其变形成一个新的张量,第一个维度不变,第二个维度变为-1,表示将第二个维度变成需要的大小,由系统自动推断。返回的张量就是展平后的结果。
例如,如果输入张量的形状为[batch_size, channel, height, width],那么经过Flatten操作后,输出张量的形状变为[batch_size, channel*height*width]。
# Style loss class GramMatrix(nn.Module): def forward(self, input): a, b, c, d = input.size() # a=batch size(=1) # b=number of feature maps # (c,d)=dimensions of a f. map (N=c*d) features = input.view(a * b, c * d) # resise F_XL into \hat F_XL G = torch.mm(features, features.t()) # compute the gram product # we 'normalize' the values of the gram matrix # by dividing by the number of element in each feature maps. return G.div(a * b * c * d) class StyleLoss(nn.Module): def __init__(self, target, weight): super(StyleLoss, self).__init__() self.target = target.detach() * weight self.weight = weight self.gram = GramMatrix() self.criterion = nn.MSELoss() def forward(self, input): self.output = input.clone() self.G = self.gram(input) self.G.mul_(self.weight) self.loss = self.criterion(self.G, self.target) return self.output def backward(self, retain_graph=True): self.loss.backward(retain_graph=retain_graph) return self.loss
这段代码是用于计算风格损失的。其中,GramMatrix类用于计算输入的Gram矩阵,即特征图的协方差矩阵,以表达输入的风格信息;StyleLoss类则用于计算输入与目标风格之间的均方误差,作为风格损失。
在forward方法中,输入被克隆为输出,并使用GramMatrix计算出输入的Gram矩阵,再乘以权重,最后计算均方误差得到风格损失。在backward方法中,反向传播损失,并返回损失值。
相关推荐
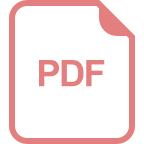
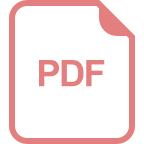
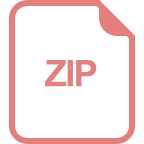












