class SelfAttention(nn.Module): def init(self, input_size=1, num_heads=1): super(SelfAttention, self).init() self.num_heads = 1 self.head_size = 1 self.query = nn.Linear(1, 1) self.key = nn.Linear(1, 1) self.value = nn.Linear(1, 1) self.out = nn.Linear(1, 1) def forward(self, inputs): batch_size, seq_len, input_size = inputs.size() # 128 706 1 # Split inputs into num_heads inputs = inputs.view(batch_size, seq_len, self.num_heads, self.head_size) inputs = inputs.permute(0, 2, 1, 3).contiguous() queries = self.query(inputs).view(batch_size, self.num_heads, seq_len, self.head_size) keys = self.key(inputs).view(batch_size, self.num_heads, seq_len, self.head_size) values = self.value(inputs).view(batch_size, self.num_heads, seq_len, self.head_size) # Compute attention scores scores = torch.matmul(queries, keys.permute(0, 1, 3, 2)) scores = scores / (self.head_size ** 0.5) attention = F.softmax(scores, dim=-1) # Apply attention weights to values attention_output = torch.matmul(attention, values) attention_output = attention_output.view(batch_size, seq_len, input_size) # Apply output linear layer output = self.out(attention_output) return output class DenseAttentionLayer(nn.Module): def init(self, input_size, return_alphas=True, name=None, num_heads=1): super(DenseAttentionLayer, self).init() self.return_alphas = return_alphas self.name = name self.num_heads = num_heads # If input comes with a hidden dimension (e.g. 5 features per gene) # print("len(input_size): ",len(input_size)) # 2 if len(input_size) == 3: self.feature_collapse = nn.Linear(input_size[-1], 1) input_size = (input_size[0], input_size[1]) self.attention = SelfAttention(input_size=1, num_heads=1) def forward(self, inputs): print("inputs.shape: ",inputs.shape) # torch.Size([128, 706]) output = self.attention(inputs) if self.return_alphas: alphas = F.softmax(output, dim=1) return torch.mul(inputs, alphas), alphas else: return output 对于上述代码其中numheads=1 headsize=1
时间: 2023-11-16 11:03:48 浏览: 60
这段代码实现了一个自注意力层(Self-Attention Layer)和一个稠密注意力层(Dense Attention Layer)。
在自注意力层中,输入被划分为多个头(num_heads),每个头的大小为head_size。然后,通过三个线性层(query、key、value)将输入映射到查询(queries)、键(keys)和值(values)空间,并计算注意力分数(scores),再通过softmax函数计算权重(attention),最后将权重与值相乘得到输出(attention_output)。
在稠密注意力层中,首先将输入进行特征折叠(feature collapse),将第三个维度(如果存在)折叠为1。然后,将折叠后的输入送入自注意力层,得到输出。如果return_alphas为True,则也返回注意力权重。
需要注意的是,这里的num_heads和head_size都被设置为1,因此实际上并没有使用多头自注意力机制。
相关问题
class SelfAttention(nn.Module): def __init__(self, input_size=1, num_heads=1): super(SelfAttention, self).__init__() self.num_heads = 1 self.head_size = 1 self.query = nn.Linear(1, 1) self.key = nn.Linear(1, 1) self.value = nn.Linear(1, 1) self.out = nn.Linear(1, 1) def forward(self, inputs): batch_size, seq_len, input_size = inputs.size() # 128 706 1 # Split inputs into num_heads inputs = inputs.view(batch_size, seq_len, self.num_heads, self.head_size) inputs = inputs.permute(0, 2, 1, 3).contiguous() queries = self.query(inputs).view(batch_size, self.num_heads, seq_len, self.head_size) keys = self.key(inputs).view(batch_size, self.num_heads, seq_len, self.head_size) values = self.value(inputs).view(batch_size, self.num_heads, seq_len, self.head_size) # Compute attention scores scores = torch.matmul(queries, keys.permute(0, 1, 3, 2)) scores = scores / (self.head_size ** 0.5) attention = F.softmax(scores, dim=-1) # Apply attention weights to values attention_output = torch.matmul(attention, values) attention_output = attention_output.view(batch_size, seq_len, input_size) # Apply output linear layer output = self.out(attention_output) return output 解释一下代码 其中num_heads=1
这段代码定义了一个自注意力模块(Self-Attention),用于在神经网络中实现自注意力机制。自注意力机制在自然语言处理领域非常常见,它可以根据当前输入中的关键词来动态地调整权重,使得模型可以更好地捕捉句子中的重要信息。
该模块的输入是一个形状为 (batch_size, seq_len, input_size) 的张量,其中 batch_size 表示批次大小,seq_len 表示序列长度,input_size 表示每个位置的向量维度。模块会将输入张量分成 num_heads 份,每份的大小为 head_size = input_size / num_heads。这里 num_heads=1,因此每个位置向量的维度大小为1。
接着,模块会通过三个线性变换(query、key、value)将每个位置的向量映射到一个新的维度上,以便计算注意力权重。将 query、key、value 映射后的结果分别表示为 queries、keys、values 张量。
然后,模块会计算得到注意力权重,具体方法是通过 queries 和 keys 的点积得到一个分数矩阵,然后对分数矩阵进行 softmax 操作得到注意力权重。最后,将注意力权重乘以 values 张量,并将结果进行加权和得到 attention_output 张量。
最后,将 attention_output 张量通过一个线性变换 out,得到最终的输出张量 output。注意,这里的 num_heads=1 表示只有一份输入,因此在计算注意力权重时并没有进行多头注意力的操作。
这是一个完整的transformer模型吗:class transformerModel(nn.Module): def __init__(self, input_size, hidden_size, num_heads, num_encoder_layers, num_decoder_layers, dropout_rate): super(transformerModel, self).__init__() self.hidden_size = hidden_size self.embedding = nn.Linear(input_size, hidden_size) encoder_layer = TransformerEncoderLayer(d_model=input_size, nhead=num_heads) self.encoder = TransformerEncoder(encoder_layer, num_encoder_layers) decoder_layer = TransformerDecoderLayer(d_model=input_size, nhead=num_heads) self.decoder = TransformerDecoder(decoder_layer, num_decoder_layers) self.fc = nn.Linear(input_size, 1) def forward(self, x): x = self.embedding(x) x = x.permute(1, 0, 2) # 调整输入维度顺序 encoding = self.encoder(x) decoding = self.decoder(encoding,encoding) out = self.fc(decoding[-1]) # 只使用最后一个时间步的输出 return out # 创建模型实例 input_size = X_train.shape[1] print(input_size) hidden_size = 6 num_heads = 1 num_encoder_layers = 2 num_decoder_layers = 2 dropout_rate = 0.2 model = transformerModel(input_size, hidden_size, num_heads, num_encoder_layers, num_decoder_layers, dropout_rate)
是的,这是一个完整的 Transformer 模型。它包括一个编码器(`self.encoder`)和一个解码器(`self.decoder`),并在 `forward()` 方法中进行了前向传播操作。模型的输入尺寸由 `input_size` 决定,隐藏层大小为 `hidden_size`,使用 `num_heads` 个头注意力机制,编码器和解码器层数由 `num_encoder_layers` 和 `num_decoder_layers` 决定。最后的输出通过线性层 `self.fc` 进行映射。你已经正确地创建了一个模型实例并进行了初始化。
相关推荐
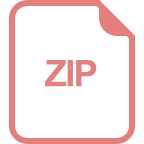
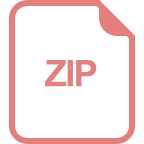
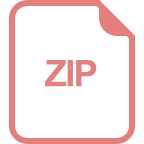













