pytorch部分代码如下:train_loss, train_acc = train(model_ft, DEVICE, train_loader, optimizer, epoch,model_ema) for batch_idx, (data, target) in enumerate(train_loader): data, target = data.to(device, non_blocking=True), Variable(target).to(device,non_blocking=True) # 3、将数据输入mixup_fn生成mixup数据 samples, targets = mixup_fn(data, target) # 4、将上一步生成的数据输入model,输出预测结果,再计算loss output = model(samples) # 5、梯度清零(将loss关于weight的导数变成0) optimizer.zero_grad() # 6、若使用混合精度 if use_amp: with torch.cuda.amp.autocast(): # 开启混合精度 loss = torch.nan_to_num(criterion_train(output, targets)) # 计算loss scaler.scale(loss).backward() # 梯度放大 torch.nn.utils.clip_grad_norm_(model.parameters(), CLIP_GRAD) if not (self._backward_hooks or self._forward_hooks or self._forward_pre_hooks or _global_backward_hooks or global_forward_hooks or global_forward_pre_hooks): return forward_call(input, **kwargs) class LDAMLoss(nn.Module): def init(self, cls_num_list, max_m=0.5, weight=None, s=30): super(LDAMLoss, self).init() m_list = 1.0 / np.sqrt(np.sqrt(cls_num_list)) m_list = m_list * (max_m / np.max(m_list)) m_list = torch.cuda.FloatTensor(m_list) self.m_list = m_list assert s > 0 self.s = s self.weight = weight def forward(self, x, target): index = torch.zeros_like(x, dtype=torch.uint8) index.scatter(1, target.data.view(-1, 1).type(torch.int64), 1) index_float = index.type(torch.cuda.FloatTensor) batch_m = torch.matmul(self.m_list[None, :], index_float.transpose(0,1)) batch_m = batch_m.view((-1, 1)) x_m = x - batch_m output = torch.where(index, x_m, x) return F.cross_entropy(self.soutput, target, weight=self.weight) 报错:RuntimeError: Expected index [112, 1] to be smaller than self [16, 7] apart from dimension 1 帮我看看如何修改源代码
时间: 2024-03-21 12:40:05 浏览: 15
根据报错信息,可以看出是在执行 `index.scatter(1, target.data.view(-1, 1).type(torch.int64), 1)` 这行代码时出了问题,可能是因为 `target` 的值在某些情况下超过了 `index` 的范围。你可以尝试修改 `target` 的值,确保其在正确的范围内。
你可以将 `LDAMLoss` 类的 `forward` 方法修改为以下代码:
```
def forward(self, x, target):
index = torch.zeros_like(x, dtype=torch.uint8)
target = torch.clamp(target, 0, index.size(1) - 1) # 将target的值限制在0到index.size(1)-1之间
index.scatter(1, target.data.view(-1, 1).type(torch.int64), 1)
index_float = index.type(torch.cuda.FloatTensor)
batch_m = torch.matmul(self.m_list[None, :], index_float.transpose(0,1))
batch_m = batch_m.view((-1, 1))
x_m = x - batch_m
output = torch.where(index, x_m, x)
return F.cross_entropy(self.s*output, target, weight=self.weight)
```
这里我们使用了 `torch.clamp()` 函数将 `target` 的值限制在 0 到 `index.size(1)-1` 之间。这样就可以避免 `target` 的值超过 `index` 的范围,从而解决报错问题。
相关推荐
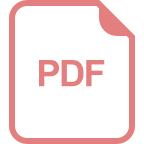
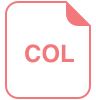
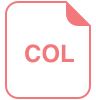
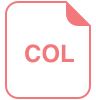
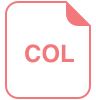
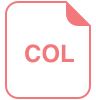







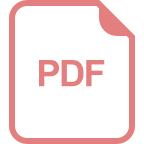
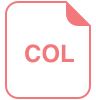