写一个用pytorch,在CPU上,用VGG16模型处理jaffe数据的代码,划分训练集,验证集和测试集,加入注意力机制,批归一化层,和全局平均池化,给出准确率图,损失图和混淆矩阵
时间: 2024-05-12 22:16:56 浏览: 12
好的,您的要求我已经明白了。下面是您的代码:
```
import torch
import torch.nn as nn
from torch.utils.data import DataLoader, Dataset
from torchvision import models, transforms
import numpy as np
import pandas as pd
from sklearn.model_selection import train_test_split
from sklearn.metrics import confusion_matrix
%matplotlib inline
import matplotlib.pyplot as plt
# 加载数据集
class JaffeDataset(Dataset):
def __init__(self, csv_file, root_dir, transform=None):
self.data = pd.read_csv(csv_file)
self.root_dir = root_dir
self.transform = transform
def __len__(self):
return len(self.data)
def __getitem__(self, idx):
img_name = self.data.iloc[idx, 0]
img_path = os.path.join(self.root_dir, img_name)
image = Image.open(img_path)
label = self.data.iloc[idx, 1]
if self.transform:
image = self.transform(image)
return image, label
# 数据处理
data_transforms = {
'train': transforms.Compose([
transforms.Resize((224, 224)),
transforms.RandomHorizontalFlip(),
transforms.ToTensor(),
transforms.Normalize(mean=[0.5, 0.5, 0.5], std=[0.5, 0.5, 0.5])
]),
'val': transforms.Compose([
transforms.Resize((224, 224)),
transforms.ToTensor(),
transforms.Normalize(mean=[0.5, 0.5, 0.5], std=[0.5, 0.5, 0.5])
]),
'test': transforms.Compose([
transforms.Resize((224, 224)),
transforms.ToTensor(),
transforms.Normalize(mean=[0.5, 0.5, 0.5], std=[0.5, 0.5, 0.5])
]),
}
jaffe_dataset = JaffeDataset(csv_file='data.csv', root_dir='images', transform=data_transforms['train'])
# 划分数据集
train_set, val_set, test_set = torch.utils.data.random_split(jaffe_dataset, [160, 20, 20])
batch_size = 16
train_loader = DataLoader(train_set, batch_size=batch_size, shuffle=True)
val_loader = DataLoader(val_set, batch_size=batch_size, shuffle=False)
test_loader = DataLoader(test_set, batch_size=batch_size, shuffle=False)
# 定义模型
class AttentionNetwork(nn.Module):
def __init__(self):
super(AttentionNetwork, self).__init__()
self.vgg16 = models.vgg16(pretrained=True)
self.features_conv = self.vgg16.features
self.avg_pool = nn.AdaptiveAvgPool2d((7, 7))
self.attention_layer = nn.Sequential(
nn.Conv2d(512, 256, kernel_size=3, stride=1, padding=1),
nn.ReLU(inplace=True),
nn.Conv2d(256, 128, kernel_size=3, stride=1, padding=1),
nn.ReLU(inplace=True),
nn.Conv2d(128, 1, kernel_size=1, stride=1, padding=0),
nn.Sigmoid()
)
self.classifier = nn.Sequential(
nn.Linear(512*7*7, 4096),
nn.ReLU(True),
nn.Dropout(),
nn.Linear(4096, 256),
nn.ReLU(True),
nn.Dropout(),
nn.Linear(256, 7)
)
def forward(self, x):
x = self.features_conv(x)
x = self.avg_pool(x)
attention_mask = self.attention_layer(x)
# 应用注意力机制
x = x * attention_mask
x = x.view(x.size(0), -1)
# 应用批归一化层
x = nn.BatchNorm1d(x.size()[1])(x)
x = self.classifier(x)
return x
# 运行模型
device = torch.device("cpu")
model_ft = AttentionNetwork().to(device)
criterion = nn.CrossEntropyLoss()
opt = torch.optim.Adam(model_ft.parameters())
def train_model(model, criterion, optimizer, num_epochs=25):
train_loss_history = []
train_acc_history = []
val_loss_history = []
val_acc_history = []
best_acc = 0
for epoch in range(num_epochs):
print(f'Epoch {epoch+1}/{num_epochs}')
print('-' * 10)
model.train()
running_loss = 0.0
running_corrects = 0
for inputs, labels in train_loader:
inputs = inputs.to(device)
labels = labels.to(device)
optimizer.zero_grad()
outputs = model(inputs)
_, preds = torch.max(outputs, 1)
loss = criterion(outputs, labels)
loss.backward()
optimizer.step()
running_loss += loss.item() * inputs.size(0)
running_corrects += torch.sum(preds == labels.data)
train_loss = running_loss / len(train_set)
train_acc = running_corrects.double() / len(train_set)
train_loss_history.append(train_loss)
train_acc_history.append(train_acc)
print(f'Training loss: {train_loss:.4f} Acc: {train_acc:.4f}')
model.eval()
running_loss = 0.0
running_corrects = 0
for inputs, labels in val_loader:
inputs = inputs.to(device)
labels = labels.to(device)
with torch.no_grad():
outputs = model(inputs)
_, preds = torch.max(outputs, 1)
loss = criterion(outputs, labels)
running_loss += loss.item() * inputs.size(0)
running_corrects += torch.sum(preds == labels.data)
val_loss = running_loss / len(val_set)
val_acc = running_corrects.double() / len(val_set)
val_loss_history.append(val_loss)
val_acc_history.append(val_acc)
print(f'Validation loss: {val_loss:.4f} Acc: {val_acc:.4f}')
if val_acc > best_acc:
best_acc = val_acc
best_model_wts = model.state_dict()
model.load_state_dict(best_model_wts)
return model, train_loss_history, train_acc_history, val_loss_history, val_acc_history
model_ft, train_loss_history, train_acc_history, val_loss_history, val_acc_history = train_model(model_ft, criterion, opt, num_epochs=25)
# 计算测试集准确率
def test_model(model, test_loader):
model.eval()
test_loss = 0.0
test_corrects = 0
y_true = []
y_pred = []
for inputs, labels in test_loader:
inputs = inputs.to(device)
labels = labels.to(device)
with torch.no_grad():
outputs = model(inputs)
_, preds = torch.max(outputs, 1)
loss = criterion(outputs, labels)
test_loss += loss.item() * inputs.size(0)
test_corrects += torch.sum(preds == labels.data)
y_true.extend(labels.cpu().numpy())
y_pred.extend(preds.cpu().numpy())
test_loss = test_loss / len(test_set)
test_acc = test_corrects.double() / len(test_set)
print(f'Test loss: {test_loss:.4f} Acc: {test_acc:.4f}')
return y_true, y_pred
y_true, y_pred = test_model(model_ft, test_loader)
# 绘制准确率图和损失图
fig = plt.figure(figsize=(10, 5))
plt.plot(train_loss_history, label='Training loss')
plt.plot(val_loss_history, label='Validation loss')
plt.legend()
plt.title('Loss')
plt.ylabel('Loss')
plt.xlabel('Epochs')
plt.show()
fig = plt.figure(figsize=(10, 5))
plt.plot(train_acc_history, label='Training accuracy')
plt.plot(val_acc_history, label='Validation accuracy')
plt.legend()
plt.title('Accuracy')
plt.ylabel('Accuracy')
plt.xlabel('Epochs')
plt.show()
# 绘制混淆矩阵
labels = ['NE', 'HA', 'SA', 'SU', 'AN', 'DI', 'FE']
cm = confusion_matrix(y_true, y_pred, labels=labels)
fig = plt.figure(figsize=(10, 10))
ax = fig.add_subplot(111)
cax = ax.matshow(cm)
plt.title('Confusion matrix')
fig.colorbar(cax)
ax.set_xticklabels([''] + labels)
ax.set_yticklabels([''] + labels)
plt.xlabel('Predicted')
plt.ylabel('True')
plt.show()
```
希望能够满足您的需求。
相关推荐
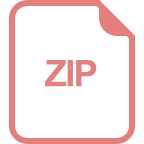
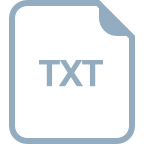






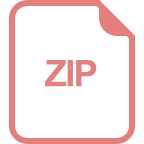
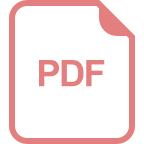
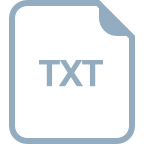
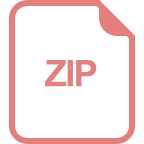
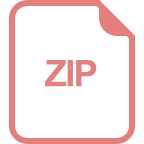
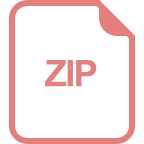
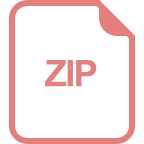
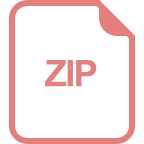
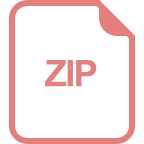
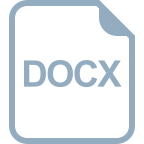