#分句分词 import pandas as pd import nltk import re import jieba hu = pd.read_csv('D:\文本挖掘\douban_data.csv',error_bad_lines=False #加入参数 ,encoding = 'gb18030') def cut_sentence(text): # 使用jieba库进行分词 seg_list = jieba.cut(text, cut_all=False) # 根据标点符号进行分句 sentence_list = [] sentence = '' for word in seg_list: sentence += word if word in ['。', '!', '?']: sentence_list.append(sentence) sentence = '' if sentence != '': sentence_list.append(sentence) return sentence_list # 获取需要分词的列 content_series =hu['comment'] # 对某一列进行分句 # sentences = [] # for text in content_series: # sentences.extend(nltk.sent_tokenize(text)) # 对每个元素进行分句 # cut_series = content_series.apply(lambda x: nltk.sent_tokenize(x)) cut_series = content_series.apply(lambda x: cut_sentence(x)) # # 对每个元素进行分词 # cut_series = content_series.apply(lambda x: nltk.word_tokenize(x)) # 将分词后的结果添加到原始的DataFrame中 xxy = pd.concat([comments, cut_series.rename('cut_sentences')], axis=1)
时间: 2024-03-04 11:54:01 浏览: 71
这段代码的作用是将一个包含评论的数据集进行分句和分词处理,并将处理后的结果添加到原始的DataFrame中。具体来说,它首先使用pandas库读取一个csv文件,然后定义了一个cut_sentence函数,使用jieba库进行分词,并根据标点符号进行分句。接着,它将需要分词的列取出,使用apply函数对每个元素进行分句和分词处理,得到一个Series对象。最后,它使用concat函数将原始DataFrame和分词后的Series对象按列合并,并将列名分别命名为comments和cut_sentences。
相关问题
分句解释这段代码 #select samples which are common to clinical file 选择临床文件中常见的样本 for (i in cancer_types){ colname_slice(str_c(dir,'\tcga_data',i,'\normalized_rna.csv'),1,12) #cut colnames colname_slice(str_c(dir,'\tcga_data',i,'\normalized_mi.csv'),1,12) } pb <- progress_bar$new(total = length(cancer_types)) for (i in cancer_types){ #intersect rna and clinical df<-read.csv(str_c(dir,'\tcga_data',i,'\normalized_rna.csv'),row.names = 1, check.names = F) df<-df[,!duplicated(colnames(df))] clinic<-read.csv(str_c(dir,'\tcga_data',i,'\TCGA-',i,'-clinical.csv'),row.names = 1, check.names = F) inter<-intersect(colnames(df),clinic[,1]) df<-df[,colnames(df)%in%inter] write.csv(df,str_c(dir,'\tcga_data',i,'\normalized_rna.csv'),quote = F) #intersect miRNA and clinical df<-read.csv(str_c(dir,'\tcga_data',i,'\normalized_mi.csv'),row.names = 1, check.names = F) df<-df[,!duplicated(colnames(df))] inter<-intersect(colnames(df),clinic[,1]) df<-df[,colnames(df)%in%inter] write.csv(df,str_c(dir,'\tcga_data',i,'\normalized_mi.csv'),quote = F) clinic<-clinic[clinic[,1]%in%inter,] write.csv(clinic,str_c(dir,'\tcga_data',i,'\TCGA-',i,'-clinical.csv'),quote = F) pb$tick() } rm(df,clinic,inter,dt)
这段代码的作用是从临床文件中选择常见的样本。首先,使用一个循环遍历变量 `cancer_types` 中的每个元素。在循环内部,通过 `colname_slice()` 函数提取文件路径,并使用 `read.csv()` 函数读取两个不同的文件(normalized_rna.csv 和 normalized_mi.csv),其中 `row.names = 1` 表示将第一列作为行名,`check.names = F` 表示不检查列名。
然后,通过交集操作找到两个数据集中共同存在的列名,并将其保留在数据框 `df` 中。接着,使用 `write.csv()` 函数将 `df` 数据框写入相应的文件路径中。
最后,通过一个进度条对象 `pb` 跟踪循环的进度,并在每次循环迭代中更新进度。循环结束后,使用 `rm()` 函数删除不再需要的变量。
总结起来,这段代码的目标是选择临床文件和两个数据集中共同存在的样本,并将处理后的数据保存到对应的文件中。
def extract_sentence(content): """第一步: 分句+分词+基础数据预处理""" sentences = split_document(content) tmp_all_sentences_words = [_seg_sent(sen) for sen in sentences] all_sentences_words = [words for words in tmp_all_sentences_words if len(words)] all_sentences = [''.join(words) for words in all_sentences_words]
这段代码是一个函数,接受一个参数 content,代表要处理的文本内容。函数的作用是将文本内容分成句子,并对每个句子进行分词和基础数据预处理。
具体来说,函数首先调用一个名为 split_document 的函数,将文本内容分成若干个句子。然后对于每个句子,调用名为 _seg_sent 的函数,将其分词并去除一些无用的词语。最后将所有句子的分词结果保存在 all_sentences_words 列表中,并将每个句子的分词结果拼接起来,保存在 all_sentences 列表中。
需要注意的是,这里的分词和基础数据预处理是通过调用外部函数实现的,代码中并没有给出这些函数的具体实现。
阅读全文
相关推荐
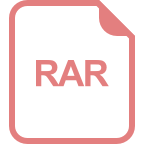
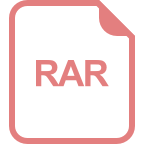
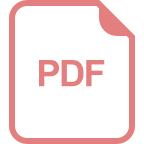
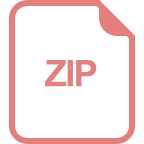
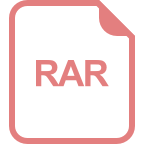
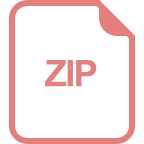
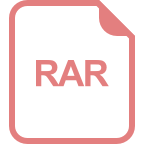





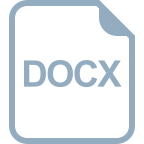
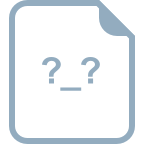