nthread = 0 nthreadpair = 0 nthreadtb = 0 ppenalty_ex = 0 stacksize: 8192 kb generating a scoring matrix for nucleotide (dist=200) ... done Gap Penalty = -1.53, +0.00, +0.00 Making a distance matrix .. There are 2627 ambiguous characters. 1501 / 1553 done. Constructing a UPGMA tree (efffree=0) ... 1550 / 1553 done. Progressive alignment 1/2... STEP 801 / 1552 f Reallocating..done. *alloclen = 32159 STEP 1001 / 1552 f
时间: 2024-04-03 18:32:11 浏览: 164
这段输出看起来是一个程序在运行时输出的信息。根据输出内容可以看出,程序正在进行一系列操作,包括生成核苷酸的评分矩阵(即计算不同核苷酸之间的匹配得分)、构建UPGMA(Unweighted Pair Group Method with Arithmetic Mean)树、以及进行渐进比对(Progressive Alignment)等步骤。
其中,生成评分矩阵是进行序列比对的基础,UPGMA树则是用来构建序列之间的进化关系,而渐进比对是一种常用的序列比对算法,可以有效地处理多个序列之间的差异。具体的参数和运行过程需要根据程序的具体实现来解释。
相关问题
nthread = 0 nthreadpair = 0 nthreadtb = 0 ppenalty_ex = 0 stacksize: 8192 kb generating a scoring matrix for nucleotide (dist=200) ... done Gap Penalty = -1.53, +0.00, +0.00 Making a distance matrix .. There are 2627 ambiguous characters. 101 / 1553
这是mafft运行时的一些输出信息,包括一些的默认值和程序运行的进度信息以下是各个参数的含义:
1. nthread: 指定使用的线程,默认值为0,表示使用单线程运。
2. nthreadpair: 指定使用的线程数,默认值为0,表示使用单线程运行。
3. nthreadtb: 指定使用的线程数,默认值为0,表示使用单线程运行。
4. ppenalty_ex: 插入空位(gap)的惩罚系数,默认值为0,表示不进行惩罚。
5. stacksize: 指定堆栈大小,默认值为8192 KB。
6. Generating a scoring matrix for nucleotide (dist=200): 生成用于核苷酸序列比对的得分矩阵,并指定距离参数为200。
7. Gap Penalty: 插入空位的惩罚系数,分别为-1.53、+0.00和+0.00。
8. Making a distance matrix: 生成序列之间的距离矩阵。
9. There are 2627 ambiguous characters: 检测到2627个模棱两可的字符,可能会影响比对结果的准确性。
10. 101 / 1553: 显示程序运行的进度信息,其中101表示已处理的序列数量,1553表示总共需要处理的序列数量。
这些输出信息可以帮助您了解mafft程序在运行时的一些基本参数和进度信息,有助于您诊断程序运行时的一些问题。
def cv_model(clf, train_x, train_y, test_x, clf_name='lgb'): folds = 5 seed = 2021 kf = KFold(n_splits=folds, shuffle=True, random_state=seed) train = np.zeros(train_x.shape[0]) test = np.zeros(test_x.shape[0]) cv_scores = [] for i, (train_index, valid_index) in enumerate(kf.split(train_x, train_y)): print('************ {} *************'.format(str(i+1))) trn_x, trn_y, val_x, val_y = train_x.iloc[train_index], train_y[train_index], train_x.iloc[valid_index], train_y[valid_index] train_matrix = clf.Dataset(trn_x, label=trn_y) valid_matrix = clf.Dataset(val_x, label=val_y) params = { 'boosting_type': 'gbdt', 'objective': 'binary', 'metric': 'auc', 'min_child_weight': 5, 'num_leaves': 2**6, 'lambda_l2': 10, 'feature_fraction': 0.9, 'bagging_fraction': 0.9, 'bagging_freq': 4, 'learning_rate': 0.01, 'seed': 2021, 'nthread': 28, 'n_jobs':-1, 'silent': True, 'verbose': -1, } model = clf.train(params, train_matrix, 50000, valid_sets=[train_matrix, valid_matrix], #categorical_feature = categorical_feature, verbose_eval=500,early_stopping_rounds=200) val_pred = model.predict(val_x, num_iteration=model.best_iteration) test_pred = model.predict(test_x, num_iteration=model.best_iteration) train[valid_index] = val_pred test += test_pred / kf.n_splits cv_scores.append(roc_auc_score(val_y, val_pred)) print(cv_scores) print("%s_scotrainre_list:" % clf_name, cv_scores) print("%s_score_mean:" % clf_name, np.mean(cv_scores)) print("%s_score_std:" % clf_name, np.std(cv_scores)) return train, test lgb_train, lgb_test = cv_model(lgb, x_train, y_train, x_test)这段代码什么意思,分类标签为0和1,属于二分类,预测结果点击率的数值是怎么来的
这段代码实现了一个基于 LightGBM 模型的交叉验证方法,其中:
- `clf` 表示 LightGBM 的分类器。
- `train_x` 和 `train_y` 分别表示训练集的特征和标签。
- `test_x` 表示测试集的特征。
- `clf_name` 表示分类器的名称,用于输出结果时的标识。
具体流程如下:
1. 将训练集分成五份,每份作为一次验证集,其余作为训练集,进行五次交叉验证。
2. 定义 LightGBM 模型的参数。
3. 对每一份验证集进行训练,得到模型。
4. 对验证集和测试集进行预测,得到预测结果。
5. 将五次交叉验证的预测结果进行平均,作为最终的预测结果。
6. 输出交叉验证的 AUC 分数,作为模型的评价指标。
在这个代码中,分类标签为 0 和 1,属于二分类问题。预测结果点击率的数值是通过模型预测得到的,其大小表示样本被预测为正例的概率,也就是点击率的估计值。
阅读全文
相关推荐
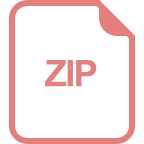
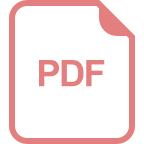
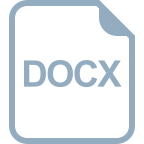
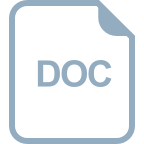
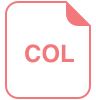
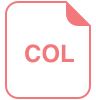










