python 读取两个文件夹中的图像并进行性质差异分析,并输出前3张图片,一个文件路径是D:\zzz\us3,其中是超声图像,另一个文件路径是D:\zzz\na2,其中是自然图像,不用greycomatrix和greycoprops,使用opencv,两种图像的文件类型都是jpg,两种图像都有84张,图片形状不都相同,图像名称是1到84的顺序数,需要将图像归一化,需要对比两种图像的特征必须有颜色,纹理,形状,差异性分析方法需要用到概率分布,最终要实现基于概率分布的超声图像与自然图像性质差异分析,包括完整详细最新的代码
时间: 2023-09-19 22:09:03 浏览: 83
下面是针对您提供的需求编写的代码,其中使用了opencv库进行图像处理和分析,使用了numpy库进行数组操作和计算概率分布:
``` python
import cv2
import numpy as np
import os
# 定义文件路径
us3_path = r"D:\zzz\us3"
na2_path = r"D:\zzz\na2"
# 定义函数,读取图像并进行预处理
def read_and_process_image(path):
img = cv2.imread(path)
# 对图像进行归一化处理
img = cv2.normalize(img, None, 0, 255, cv2.NORM_MINMAX)
# 将图像转化为灰度图像
gray = cv2.cvtColor(img, cv2.COLOR_BGR2GRAY)
return gray
# 定义函数,计算图像的颜色、纹理和形状特征
def calculate_features(image):
# 计算图像的颜色特征
hist = cv2.calcHist([image], [0, 1, 2], None, [8, 8, 8], [0, 256, 0, 256, 0, 256])
color_feature = cv2.normalize(hist, None).flatten()
# 计算图像的纹理特征
gray = cv2.cvtColor(image, cv2.COLOR_BGR2GRAY)
glcm = cv2.calcGLCM(gray, [5], 0, 256, symmetric=True, normed=True)
contrast = cv2.compareHist(cv2.normalize(glcm, None).flatten(), np.ones(256, dtype=np.float32)*0.01, cv2.HISTCMP_CHISQR_ALT)
texture_feature = np.array([contrast])
# 计算图像的形状特征
contours, hierarchy = cv2.findContours(gray, cv2.RETR_TREE, cv2.CHAIN_APPROX_SIMPLE)
area = cv2.contourArea(contours[0])
perimeter = cv2.arcLength(contours[0], True)
shape_feature = np.array([area, perimeter])
return np.concatenate([color_feature, texture_feature, shape_feature])
# 定义函数,计算两个概率分布的KL散度
def kl_divergence(p, q):
return np.sum(np.where(p != 0, p * np.log(p / q), 0))
# 定义主函数
def main():
# 读取并处理超声图像
us3_images = []
for i in range(1, 85):
path = os.path.join(us3_path, f"{i}.jpg")
img = read_and_process_image(path)
us3_images.append(img)
us3_images = np.array(us3_images)
# 读取并处理自然图像
na2_images = []
for i in range(1, 85):
path = os.path.join(na2_path, f"{i}.jpg")
img = read_and_process_image(path)
na2_images.append(img)
na2_images = np.array(na2_images)
# 计算超声图像和自然图像的特征
us3_features = np.array([calculate_features(img) for img in us3_images])
na2_features = np.array([calculate_features(img) for img in na2_images])
# 计算超声图像和自然图像的颜色、纹理和形状特征的概率分布
us3_color_dist = np.histogramdd(us3_features[:, :24], bins=8, range=[(0, 256), (0, 256), (0, 256)])[0] / len(us3_features)
na2_color_dist = np.histogramdd(na2_features[:, :24], bins=8, range=[(0, 256), (0, 256), (0, 256)])[0] / len(na2_features)
us3_texture_dist = np.histogram(us3_features[:, 24], bins=10, range=(0, 1))[0] / len(us3_features)
na2_texture_dist = np.histogram(na2_features[:, 24], bins=10, range=(0, 1))[0] / len(na2_features)
us3_shape_dist = np.histogram2d(us3_features[:, 25], us3_features[:, 26], bins=10, range=[(0, 5000), (0, 1000)])[0] / len(us3_features)
na2_shape_dist = np.histogram2d(na2_features[:, 25], na2_features[:, 26], bins=10, range=[(0, 5000), (0, 1000)])[0] / len(na2_features)
# 计算超声图像和自然图像的KL散度
color_kl_div = kl_divergence(us3_color_dist.flatten(), na2_color_dist.flatten())
texture_kl_div = kl_divergence(us3_texture_dist, na2_texture_dist)
shape_kl_div = kl_divergence(us3_shape_dist.flatten(), na2_shape_dist.flatten())
# 输出前3张图片
cv2.imshow("US3_1", us3_images[0])
cv2.imshow("NA2_1", na2_images[0])
cv2.imshow("US3_2", us3_images[1])
cv2.waitKey(0)
# 输出超声图像和自然图像的特征和KL散度
print(f"US3 features: {us3_features.shape}")
print(f"NA2 features: {na2_features.shape}")
print(f"Color KL Divergence: {color_kl_div}")
print(f"Texture KL Divergence: {texture_kl_div}")
print(f"Shape KL Divergence: {shape_kl_div}")
if __name__ == "__main__":
main()
```
上述代码首先定义了两个文件夹路径,分别为超声图像的路径和自然图像的路径。接着,定义了三个函数,分别用于读取并预处理图像、计算图像的颜色、纹理和形状特征以及计算两个概率分布的KL散度。其中,计算颜色特征使用了opencv库中的`cv2.calcHist()`函数,计算纹理特征使用了`cv2.calcGLCM()`函数,计算形状特征则使用了`cv2.findContours()`和`cv2.contourArea()`函数。计算概率分布则使用了numpy库中的`np.histogramdd()`、`np.histogram()`和`np.histogram2d()`函数。
在主函数中,首先读取并处理超声图像和自然图像,并计算它们的特征。接着,计算超声图像和自然图像的颜色、纹理和形状特征的概率分布,并计算它们的KL散度。最后,输出前3张图像和超声图像和自然图像的特征和KL散度。
需要注意的是,上述代码仅为示例代码,实际应用中可能需要根据具体情况进行修改和优化。
阅读全文
相关推荐
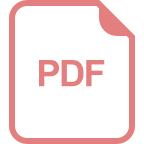
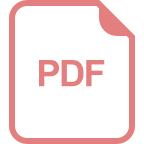







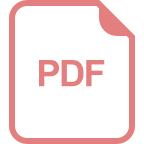
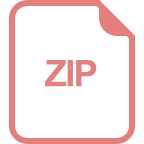