如果想自定义U-net网络的损失函数为IoU函数,应该如何修改代码
时间: 2024-03-21 15:41:18 浏览: 29
U-Net网络通常使用交叉熵损失函数进行训练,但是如果你想将损失函数改为IoU函数,可以按照以下步骤进行修改:
1. 定义IoU函数
首先,需要定义IoU函数。IoU函数可以定义为真实标签和预测标签之间的交集与并集之比。可以使用PyTorch中的函数来计算交集和并集。
```python
def iou(output, target, smooth=1):
intersection = (output & target).sum(dim=(2, 3))
union = (output | target).sum(dim=(2, 3))
iou = (intersection + smooth) / (union - intersection + smooth)
return iou.mean()
```
2. 修改损失函数
将交叉熵损失函数替换为IoU损失函数。在PyTorch中,损失函数通常是一个可调用对象,输入为模型输出和真实标签,输出为损失值。因此,我们可以定义一个IoULoss类,并实现__call__方法来计算IoU损失。
```python
class IoULoss(nn.Module):
def __init__(self, smooth=1):
super(IoULoss, self).__init__()
self.smooth = smooth
def forward(self, output, target):
intersection = (output & target).sum(dim=(2, 3))
union = (output | target).sum(dim=(2, 3))
iou = (intersection + self.smooth) / (union - intersection + self.smooth)
loss = 1 - iou.mean()
return loss
```
3. 修改训练代码
将使用交叉熵损失函数的部分替换为IoU损失函数。在U-Net网络中,输出是一个二进制掩码,因此我们需要使用sigmoid函数将输出转换为概率值。然后,将概率值与阈值进行比较,生成二进制掩码。最后,使用IoU损失函数计算损失值。
```python
criterion = IoULoss()
optimizer = torch.optim.Adam(model.parameters(), lr=learning_rate)
for epoch in range(num_epochs):
for i, (images, labels) in enumerate(train_loader):
images = images.to(device)
labels = labels.to(device)
# 前向传播
outputs = model(images)
probs = torch.sigmoid(outputs)
preds = (probs > 0.5).float()
# 计算损失函数
loss = criterion(preds, labels)
# 反向传播和优化
optimizer.zero_grad()
loss.backward()
optimizer.step()
# 打印损失函数值
if (i + 1) % 10 == 0:
print('Epoch [{}/{}], Step [{}/{}], Loss: {:.4f}'
.format(epoch+1, num_epochs, i+1, total_step, loss.item()))
```
这样就可以使用IoU损失函数训练U-Net网络了。需要注意的是,IoU函数是一个非凸函数,可能会导致训练过程中的局部最优解。因此,你可能需要尝试不同的超参数组合来找到较好的结果。
相关推荐
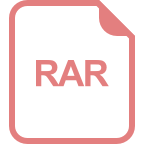
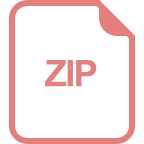
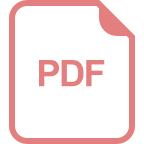














