self.avg_pool = nn.AdaptiveAvgPool2d(1) 解释该段代码
时间: 2024-05-28 19:15:08 浏览: 9
这段代码使用PyTorch中的nn库实例化了一个名为"avg_pool"的AdaptiveAvgPool2d层,该层对输入的二维张量(即图像)进行自适应平均池化操作,将每个通道内的值取平均,最后输出的是形状为[N,C,1,1]的张量,其中N是批大小,C是通道数,1表示高度和宽度均为1。这种自适应池化可以处理不同尺寸的输入数据。
相关问题
self.avg_pool = nn.AdaptiveAvgPool2d((1, 1))
This code snippet creates an instance of the nn.AdaptiveAvgPool2d module with a target output size of (1, 1).
The AdaptiveAvgPool2d module is used for global average pooling, which is a technique commonly used in convolutional neural networks for image classification. It takes as input a tensor of shape (batch_size, channels, height, width), and computes the average value of each channel across all spatial dimensions (height and width) to produce a tensor of shape (batch_size, channels, 1, 1).
By setting the output size to (1, 1), the module ensures that the resulting tensor has a fixed size regardless of the input size, which can be useful for downstream layers that require a fixed input size.
class ChannelAttention(nn.Module): def __init__(self, in_planes, ratio = 4): super(ChannelAttention, self).__init__() self.avg_pool = nn.AdaptiveAvgPool2d(1) self.max_pool = nn.AdaptiveMaxPool2d(1) self.fc1 = nn.Conv2d(in_planes, in_planes // ratio, 1, bias=False) self.relu1 = nn.ReLU() self.fc2 = nn.Conv2d(in_planes // ratio, in_planes, 1, bias=False) self.sigmoid = nn.Sigmoid() def forward(self, x): avg_out = self.fc2(self.relu1(self.fc1(self.avg_pool(x)))) max_out = self.fc2(self.relu1(self.fc1(self.max_pool(x)))) out = avg_out + max_out return self.sigmoid(out) 改为tensorflow形式
在 TensorFlow 中,可以使用以下代码来实现相同的通道注意力模块:
```python
import tensorflow as tf
class ChannelAttention(tf.keras.layers.Layer):
def __init__(self, in_planes, ratio=4):
super(ChannelAttention, self).__init__()
self.avg_pool = tf.keras.layers.GlobalAvgPool2D()
self.max_pool = tf.keras.layers.GlobalMaxPool2D()
self.fc1 = tf.keras.layers.Conv2D(in_planes // ratio, kernel_size=1, strides=1, use_bias=False)
self.relu1 = tf.keras.layers.ReLU()
self.fc2 = tf.keras.layers.Conv2D(in_planes, kernel_size=1, strides=1, use_bias=False)
self.sigmoid = tf.keras.layers.Activation('sigmoid')
def call(self, x):
avg_out = self.fc2(self.relu1(self.fc1(self.avg_pool(x))))
max_out = self.fc2(self.relu1(self.fc1(self.max_pool(x))))
out = avg_out + max_out
return self.sigmoid(out)
```
在 TensorFlow 中,我们使用 `tf.keras.layers` 模块来定义网络层,使用 `call()` 方法来实现前向传播。这里的 `GlobalAvgPool2D` 和 `GlobalMaxPool2D` 分别代替了 PyTorch 中的 `AdaptiveAvgPool2d` 和 `AdaptiveMaxPool2d`,它们也可以用来对不同尺寸的输入进行平均池化和最大池化。另外,TensorFlow 中没有 `nn.ReLU` 模块,我们需要使用 `tf.keras.layers.ReLU()` 来替代。其他的代码实现和 PyTorch 版本相同。
相关推荐
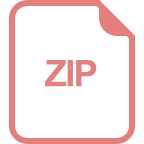













