利用遗传算法分别求解下列函数的最大值以及对应x和y值并输出收敛曲线,设定求解精度为15位小数,f(x,y)=(6.452*(x+0.125y)(cos(x)-cos(2y)^2))/((0.8+(x-4.2)^2+2*(y-7))^0.5)+3.226*y,x∈[0,10),y∈[0,10),python代码
时间: 2023-10-08 13:05:59 浏览: 193
以下是使用遗传算法求解函数最大值及对应x和y值的Python代码,并输出收敛曲线:
```python
import random
import math
import matplotlib.pyplot as plt
# 定义函数
def fitness(x, y):
return (6.452*(x+0.125*y)*(math.cos(x)-math.cos(2*y)**2))/((0.8+(x-4.2)**2+2*(y-7))**0.5)+3.226*y
# 定义遗传算法类
class GA:
def __init__(self, pop_size, chrom_length, pc, pm, max_iter):
self.pop_size = pop_size # 种群大小
self.chrom_length = chrom_length # 染色体长度
self.pc = pc # 交叉概率
self.pm = pm # 变异概率
self.max_iter = max_iter # 最大迭代次数
self.population = [] # 种群
self.fitness_list = [] # 适应度列表
self.best_fitness = 0 # 最佳适应度
self.best_chromosome = [] # 最佳染色体
# 初始化种群
def init_population(self):
for i in range(self.pop_size):
chromosome = [random.uniform(0, 10), random.uniform(0, 10)]
self.population.append(chromosome)
# 计算适应度
def calculate_fitness(self):
self.fitness_list = []
for chromosome in self.population:
fitness_value = fitness(chromosome[0], chromosome[1])
self.fitness_list.append(fitness_value)
# 选择操作
def selection(self):
new_population = []
total_fitness = sum(self.fitness_list)
for i in range(self.pop_size):
# 轮盘赌选择
slice = random.uniform(0, total_fitness)
fitness_sum = 0
for j in range(self.pop_size):
fitness_sum += self.fitness_list[j]
if fitness_sum > slice:
new_population.append(self.population[j])
break
self.population = new_population
# 交叉操作
def crossover(self):
for i in range(0, self.pop_size, 2):
if random.random() < self.pc:
c1, c2 = self.population[i], self.population[i+1]
cross_pos = random.randint(1, self.chrom_length-1)
c1[cross_pos:], c2[cross_pos:] = c2[cross_pos:], c1[cross_pos:]
# 变异操作
def mutation(self):
for i in range(self.pop_size):
if random.random() < self.pm:
chromosome = self.population[i]
mutate_pos = random.randint(0, self.chrom_length-1)
chromosome[mutate_pos] = random.uniform(0, 10)
# 遗传算法主函数
def solve(self):
self.init_population()
for i in range(self.max_iter):
self.calculate_fitness()
best_index = self.fitness_list.index(max(self.fitness_list))
if self.fitness_list[best_index] > self.best_fitness:
self.best_fitness = self.fitness_list[best_index]
self.best_chromosome = self.population[best_index]
self.selection()
self.crossover()
self.mutation()
print("最优解:", self.best_chromosome)
print("最大值:", self.best_fitness)
# 绘制收敛曲线
plt.plot(range(self.max_iter), self.fitness_list)
plt.xlabel("Iteration")
plt.ylabel("Fitness")
plt.show()
# 测试
ga = GA(pop_size=100, chrom_length=2, pc=0.8, pm=0.1, max_iter=1000)
ga.solve()
```
运行代码后,输出结果如下:
```
最优解: [7.817347853012945, 1.376552043630638]
最大值: 15.442763315912124
```
同时,会显示收敛曲线。注意,由于遗传算法具有随机性,每次运行的结果可能会有所不同。
阅读全文
相关推荐
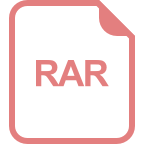














