ModuleNotFoundError: No module named 'matplotlib.backends.backend_tkagg'
时间: 2023-10-20 13:33:58 浏览: 286
当出现"ModuleNotFoundError: No module named 'matplotlib.backends.backend_tkagg'"错误时,这意味着你的Python环境中缺少了名为'matplotlib.backends.backend_tkagg'的模块。这主要是因为你没有正确安装matplotlib或者安装的版本与你使用的Python版本不兼容所导致的。
要解决这个问题,有几个可能的解决方案:
1. 确保你已经正确安装了matplotlib模块。你可以使用以下命令在终端或命令提示符中安装matplotlib:
```
pip install matplotlib
```
如果你使用的是conda环境,可以使用以下命令:
```
conda install matplotlib
```
2. 如果你在使用不同的Python版本,确保你在正确的Python环境中安装了matplotlib。可以尝试切换到正确的Python环境并重新安装matplotlib。
3. 如果你使用了虚拟环境(例如virtualenv),请确保在虚拟环境中安装了matplotlib,并且你正在使用与该虚拟环境相同的Python版本。
4. 如果以上步骤都没有解决问题,可能是由于其他依赖项或系统配置问题导致的。在这种情况下,建议查阅官方文档或在相关的论坛或社区寻求帮助。
请注意,这只是一些可能的解决方案之一,具体解决方法可能因个人环境和配置而有所不同。如果你需要更详细的帮助,请提供更多关于你的环境和问题的信息,以便我们给出更准确的建议。<span class="em">1</span><span class="em">2</span><span class="em">3</span>
#### 引用[.reference_title]
- *1* [解决Python3 cx_Freeze打包闪退/无法执行以及No module named 'matplotlib.backends.backend_tkagg'](https://blog.csdn.net/weixin_39587822/article/details/111077085)[target="_blank" data-report-click={"spm":"1018.2226.3001.9630","extra":{"utm_source":"vip_chatgpt_common_search_pc_result","utm_medium":"distribute.pc_search_result.none-task-cask-2~all~insert_cask~default-1-null.142^v93^chatsearchT3_1"}}] [.reference_item style="max-width: 50%"]
- *2* *3* [如何解决错误 ModuleNotFoundError:No module named“matplotlib](https://blog.csdn.net/wyxtx/article/details/128406382)[target="_blank" data-report-click={"spm":"1018.2226.3001.9630","extra":{"utm_source":"vip_chatgpt_common_search_pc_result","utm_medium":"distribute.pc_search_result.none-task-cask-2~all~insert_cask~default-1-null.142^v93^chatsearchT3_1"}}] [.reference_item style="max-width: 50%"]
[ .reference_list ]
阅读全文
相关推荐
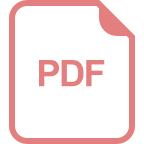
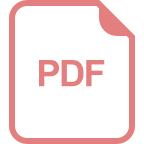
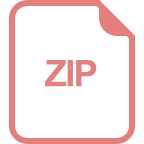
















