翻译这段代码: df.set_index(['time'],drop=False,inplace=True)
时间: 2024-01-20 10:04:31 浏览: 76
这段代码是Python代码,主要是对DataFrame数据集df进行操作,将DataFrame数据集df的索引设置为'time'列,并且保留'time'列。具体而言,set_index()方法用于设置DataFrame数据集的索引,['time']表示将'time'列设置为新的索引,drop=False表示保留'time'列,inplace=True表示在原数据集df上进行操作,不创建新的数据集。这段代码执行后,df数据集的索引将被设置为'time'列,同时'time'列也会保留。
相关问题
优化代码 def cluster_format(self, start_time, end_time, save_on=True, data_clean=False, data_name=None): """ local format function is to format data from beihang. :param start_time: :param end_time: :return: """ # 户用簇级数据清洗 if data_clean: unused_index_col = [i for i in self.df.columns if 'Unnamed' in i] self.df.drop(columns=unused_index_col, inplace=True) self.df.drop_duplicates(inplace=True, ignore_index=True) self.df.reset_index(drop=True, inplace=True) dupli_header_lines = np.where(self.df['sendtime'] == 'sendtime')[0] self.df.drop(index=dupli_header_lines, inplace=True) self.df = self.df.apply(pd.to_numeric, errors='ignore') self.df['sendtime'] = pd.to_datetime(self.df['sendtime']) self.df.sort_values(by='sendtime', inplace=True, ignore_index=True) self.df.to_csv(data_name, index=False) # 调用基本格式化处理 self.df = super().format(start_time, end_time) module_number_register = np.unique(self.df['bat_module_num']) # if registered m_num is 0 and not changed, there is no module data if not np.any(module_number_register): logger.logger.warning("No module data!") sys.exit() if 'bat_module_voltage_00' in self.df.columns: volt_ref = 'bat_module_voltage_00' elif 'bat_module_voltage_01' in self.df.columns: volt_ref = 'bat_module_voltage_01' elif 'bat_module_voltage_02' in self.df.columns: volt_ref = 'bat_module_voltage_02' else: logger.logger.warning("No module data!") sys.exit() self.df.dropna(axis=0, subset=[volt_ref], inplace=True) self.df.reset_index(drop=True, inplace=True) self.headers = list(self.df.columns) # time duration of a cluster self.length = len(self.df) if self.length == 0: logger.logger.warning("After cluster data clean, no effective data!") raise ValueError("No effective data after cluster data clean.") self.cluster_stats(save_on) for m in range(self.mod_num): print(self.clusterid, self.mod_num) self.module_list.append(np.unique(self.df[f'bat_module_sn_{str(m).zfill(2)}'].dropna())[0])
Here are some possible optimizations for the given code:
1. Instead of using a list comprehension to find columns with 'Unnamed' in their names, you can use the `filter()` function along with a lambda function to achieve the same result in a more concise way:
```
unused_index_col = list(filter(lambda x: 'Unnamed' in x, self.df.columns))
```
2. Instead of dropping duplicates and resetting the index separately, you can use the `drop_duplicates()` function with the `ignore_index` parameter set to `True` to achieve both in one step:
```
self.df.drop_duplicates(inplace=True, ignore_index=True)
```
3. Instead of using `sys.exit()` to terminate the program when there is no module data, you can raise a `ValueError` with an appropriate error message:
```
raise ValueError("No module data!")
```
4. Instead of using a series of `if` statements to find the voltage reference column, you can use the `loc` accessor with a boolean mask to select the first column that starts with 'bat_module_voltage':
```
volt_ref_col = self.df.columns[self.df.columns.str.startswith('bat_module_voltage')][0]
```
5. Instead of using a loop to append a single item to a list, you can use the `append()` method directly:
```
self.module_list.append(np.unique(self.df[f'bat_module_sn_{str(m).zfill(2)}'].dropna())[0])
```
By applying these optimizations, the code can become more concise and efficient.
这段代码是什么布尔运算:df.set_index(['time','id'],drop=False,inplace=True) df=df[~df.index.duplicated()] df.set_index(['time'],drop=False,inplace=True) df['dayofweek'] = df.index.dayofweek df['day'] = df.index.day df['hour'] = df.index.hour new = df["id"].str.split(".", expand = True) df['highway']=new[0] df['direction_code']=new[1] df['direction_dist']=pd.to_numeric(new[2])
这段代码中没有涉及到布尔运算,它是一系列DataFrame数据集df的操作。具体而言,这段代码的作用是:
1. 将DataFrame数据集df的索引设置为两列,分别是'time'和'id'列,并且保留这两列:`df.set_index(['time','id'],drop=False,inplace=True)`
2. 去除索引中的重复行:`df=df[~df.index.duplicated()]`
3. 将DataFrame数据集df的索引设置为'time'列,并且保留'time'列:`df.set_index(['time'],drop=False,inplace=True)`
4. 添加一个新的列'dayofweek',其值为索引中的星期几信息:`df['dayofweek'] = df.index.dayofweek`
5. 添加一个新的列'day',其值为索引中的日期信息:`df['day'] = df.index.day`
6. 添加一个新的列'hour',其值为索引中的小时信息:`df['hour'] = df.index.hour`
7. 将'id'列中的字符串按照"."进行分割,并将分割后的结果作为新的列添加到数据集df中:`new = df["id"].str.split(".", expand = True)`
8. 添加一个新的列'highway',其值为'id'列中分割后的第一部分:`df['highway']=new[0]`
9. 添加一个新的列'direction_code',其值为'id'列中分割后的第二部分:`df['direction_code']=new[1]`
10. 将'id'列中分割后的第三部分转换为数值类型,并添加到数据集df中作为新的列'direction_dist':`df['direction_dist']=pd.to_numeric(new[2])`
阅读全文
相关推荐
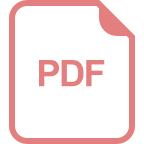
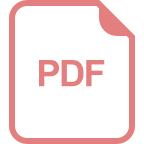













