pytorch embedding
时间: 2023-06-15 12:04:55 浏览: 56
在PyTorch中,嵌入(embedding)层是将离散特征转换为密集向量的常见方式。它通常用于自然语言处理(NLP)中,其中单词或字符被编码为离散的整数,然后通过嵌入层将其转换为连续的向量表示。
嵌入层是一个简单的矩阵乘法,其中输入是一个整数张量,每个整数都对应于一个嵌入向量。嵌入层的输出是一个张量,其中每个整数都被替换为相应的嵌入向量。嵌入层的权重矩阵是一个大小为(输入大小,输出大小)的矩阵,其中输入大小是嵌入层的输入维度,而输出大小是嵌入向量的维度。
在PyTorch中,可以使用torch.nn.Embedding类来创建嵌入层。以下是一个简单的例子,展示如何创建和使用嵌入层:
``` python
import torch.nn as nn
# 创建一个嵌入层,输入大小为1000,输出大小为50
embedding = nn.Embedding(1000, 50)
# 定义一个输入张量
input_tensor = torch.LongTensor([1, 2, 3, 4, 5])
# 将输入张量传递给嵌入层
embedded = embedding(input_tensor)
# 输出嵌入向量的形状
print(embedded.shape) # 输出: torch.Size([5, 50])
```
在上面的例子中,我们首先创建了一个大小为(1000,50)的嵌入层,然后创建了一个包含5个整数的输入张量。我们将输入张量传递给嵌入层,得到一个大小为(5,50)的嵌入向量张量。
相关问题
pytorch embedding demo code
Here is a simple PyTorch embedding demo code:
```
import torch
import torch.nn as nn
# Define some input data
input_data = torch.LongTensor([[1, 2, 3], [4, 5, 6]])
# Define the embedding layer
embedding_layer = nn.Embedding(num_embeddings=10, embedding_dim=4)
# Pass the input data through the embedding layer
embedded_data = embedding_layer(input_data)
# Print the output
print(embedded_data)
```
In this code, we first define some input data as a LongTensor with shape (2, 3). We then define an embedding layer using nn.Embedding, specifying the number of embeddings (num_embeddings) and the dimension of each embedding (embedding_dim). We then pass the input_data through the embedding layer using embedding_layer(input_data), which returns the embedded_data with shape (2, 3, 4), where the last dimension corresponds to the embedding dimension. Finally, we print the output.
pytorch embedding层
PyTorch中的embedding层是一种用于将离散变量映射到连续向量空间的神经网络层。它将输入的整数序列转换为对应的向量序列,这些向量可以用于后续的神经网络模型中。embedding层的参数是一个矩阵,其中每一行对应一个离散变量的向量表示。在训练过程中,这些向量会被学习,以最小化模型的损失函数。embedding层在自然语言处理等领域中广泛应用。
相关推荐
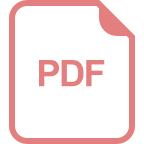












