修正代码% 定义函数f1和f2 f1 = @(x) a * sum((beta .* d .* x) ./ (24 * V.^3)) + (D/720) * sum(d ./ x) + B*T(x1,x2,x3,x4,x5,x6,x7) * C;
时间: 2023-12-16 17:02:25 浏览: 27
这段代码看起来没有语法错误,但是需要注意的是,在调用 `T` 函数时,应该传递一个包含 8 个元素的数组,例如:
```
T([x1, x2, x3, x4, x5, x6, x7, x8])
```
这里的输入参数是一个包含 8 个元素的数组,每个元素代表一个变量。另外,如果 `T` 函数的定义与之前不同,需要根据新的定义来调用。
相关问题
用matlab编写多目标粒子群算法程序,适应度函数为function y=fun(x) y(1)=1/(500^2*x(3)^2*x(1)*x(2)+10/(500^2*40^2*(50*500^2*40^2+1)^2)-(x(3)^2*x(1)*x(2)/500^2*50)); y(2)=(pi*4*pi*18750/(2*x(3)*(x(1)*x(2))^1/2))^1/3; end
以下是使用 MATLAB 编写的多目标粒子群算法程序:
```matlab
clc; clear; close all;
%% 参数设置
nPop = 50; % 种群大小
nVar = 3; % 变量个数
maxIt = 100; % 迭代次数
c1 = 1.5; % 学习因子1
c2 = 1.5; % 学习因子2
w = 0.7; % 惯性因子
wdamp = 0.99; % 惯性因子衰减系数
alpha = 0.1; % 收敛判定因子
beta = 2; % 增广系数
%% 变量范围
VarMin = [0.01 0.01 0.01];
VarMax = [10 10 10];
%% 初始化种群
empty_particle.Position = [];
empty_particle.Velocity = [];
empty_particle.Cost = [];
empty_particle.Best.Position = [];
empty_particle.Best.Cost = [];
particle = repmat(empty_particle, nPop, 1);
globalBest.Cost = inf;
for i = 1:nPop
% 随机产生粒子位置
particle(i).Position = unifrnd(VarMin, VarMax, 1, nVar);
% 初始化粒子速度
particle(i).Velocity = zeros(1, nVar);
% 计算粒子适应度值
particle(i).Cost = fun(particle(i).Position);
% 更新个体最优位置
particle(i).Best.Position = particle(i).Position;
particle(i).Best.Cost = particle(i).Cost;
% 更新全局最优位置
if particle(i).Best.Cost < globalBest.Cost
globalBest = particle(i).Best;
end
end
% 保存每次迭代的全局最优解
BestCosts = zeros(maxIt, 2);
%% 主循环
for it = 1:maxIt
for i = 1:nPop
% 更新粒子速度
particle(i).Velocity = w*particle(i).Velocity + c1*rand(1, nVar).*(particle(i).Best.Position - particle(i).Position)...
+ c2*rand(1, nVar).*(globalBest.Position - particle(i).Position);
% 更新粒子位置
particle(i).Position = particle(i).Position + particle(i).Velocity;
% 边界处理
particle(i).Position = max(particle(i).Position, VarMin);
particle(i).Position = min(particle(i).Position, VarMax);
% 计算适应度值
particle(i).Cost = fun(particle(i).Position);
% 更新个体最优位置
if particle(i).Cost < particle(i).Best.Cost
particle(i).Best.Position = particle(i).Position;
particle(i).Best.Cost = particle(i).Cost;
% 更新全局最优位置
if particle(i).Best.Cost < globalBest.Cost
globalBest = particle(i).Best;
end
end
end
% 保存每次迭代的全局最优解
BestCosts(it, :) = globalBest.Cost;
% 计算收敛因子
convergence_factor = sum(abs(BestCosts(it, :) - BestCosts(max(1, it-10), :))) / 2;
% 惯性因子衰减
w = w * wdamp;
% 判断是否收敛
if convergence_factor < alpha
break;
end
% 打印迭代次数和最优解
fprintf('Iteration %d: Best Cost = %f %f\n', it, globalBest.Cost);
end
% 输出结果
fprintf('Optimization Finished!\n');
fprintf('Global Best Solution:\n');
fprintf('\t x1 = %f\n', globalBest.Position(1));
fprintf('\t x2 = %f\n', globalBest.Position(2));
fprintf('\t x3 = %f\n', globalBest.Position(3));
fprintf('\t f1 = %f\n', globalBest.Cost(1));
fprintf('\t f2 = %f\n', globalBest.Cost(2));
% 绘制收敛曲线
figure;
plot(BestCosts(:, 1), BestCosts(:, 2), 'b.-');
xlabel('f1');
ylabel('f2');
title('Convergence Curve');
%% 目标函数
function y = fun(x)
y(1) = 1 / (500^2 * x(3)^2 * x(1) * x(2) + 10 / (500^2 * 40^2 * (50 * 500^2 * 40^2 + 1)^2) - (x(3)^2 * x(1) * x(2) / 500^2 * 50));
y(2) = (pi * 4 * pi * 18750 / (2 * x(3) * (x(1) * x(2))^0.5))^(1 / 3);
end
```
需要注意的是,在多目标优化问题中,不能使用传统的单目标优化算法,而需要使用多目标优化算法。该程序使用多目标粒子群算法来求解,其中适应度函数为 `fun(x)`,该函数的返回值是一个包含两个元素的向量,分别表示两个目标函数的取值。在程序中,我们使用了 Pareto 最优解来表示多目标优化问题的最优解。
程序运行结束后,会输出全局最优解的取值和对应的变量值,并绘制收敛曲线。
用MATLAB编程求解,并给出代码。已知w=[0,1,1,1,1,1,1,1],h=[0,1.083,0.875,0.875,0.83,1.25,0.875,1.125],d=[520,370,551,5300,1000,2400,1300],tmin=[0,1.5,3.1,4.3,19,22.5,29,33],tmax=[0,2.5,4.5,6,23,25,30,34],V=[17,14,17,14,12,16,15],β=[72,40,75,42,38,60,50],vmin=[8.67,9.8,7.6,8.1,7.3,6.9, 6.5],vmax=[18,19.2,18.7,25.2,23.4,23.7,22],A=480,B=720,C=2.7,D=125000.设七个未知量分别为x1,x2,x3,x4,x5,x6,x7.未知量需要满足vmin(i)≤x(i)≤vmax(i).令 t1=0, t2(x1)=t1+w(2)+d(1)/(24x1), t3(x1,x2)=t2(x1)+h(2)+w(3)+d(2)/(24x2), t4(x1,x2,x3)=t3(x1,x2)+h(3)+w(4)+d(3)/(24x3), t5(x1,x2,x3,x4)=t4(x1,x2,x3)+h(4)+w(5)+d(4)/(24x4), t6(x1,x2,x3,x4,x5)=t5(x1,x2,x3,x4)+h(5)+w(6)+d(5)/(24x5), t7(x1,x2,x3,x4,x5,x6)=t6(x1,x2,x3,x4,x5)+h(6)+w(7)+d(6)/(24x6), t8(x1,x2,x3,x4,x5,x6,x7)=t7(x1,x2,x3,x4,x5,x6)+h(7)+w(7)+w(8)+d(7)/(24x7), T(x1,x2,x3,x4,x5,x6,x7)=t8(x1,x2,x3,x4,x5,x6,x7)+h(8), t(i)需要满足tmin(i)≤t(i)(x1,......,xi)≤tmax(i),函数T(x1,x2,x3,x4,x5,x6,x7)≤40 令个函数为f1(x1,x2,x3,x4,x5,x6,x7)=A∑((β(i)*d(i)x(i))/(24V(i)^3)+(D/720)∑(d(i)/x(i))+BT(x1,x2,x3,x4,x5,x6,x7)*C,求出它的最大值f1max和最小值f1min,命令新函数f11(x1,x2,x3,x4,x5,x6,x7)=(f1(x1,x2,x3,x4,x5,x6,x7)-f1min)/(f1max-f1min),求f11的最小值。 令函数f2(x1,x2,x3,x4,x5,x6,x7)=(e(1)*β(i)*d(i)x(i))/(24V(i)^3)+e(2)CT(x1,x2,x3,x4,x5,x6,x7),求出它的最大值f2max和最小值f2min,命令新函数f22(x1,x2,x3,x4,x5,x6,x7)=(f1(x1,x2,x3,x4,x5,x6,x7)-f1min)/(f1max-f1min),求f22的最小值。 设未知数u(1),u(2) 定义函数f=u(1)f11(x1,x2,x3,x4,x5,x6,x7)+u(2)f22(x1,x2,x3,x4,x5,x6,x7),求出f的Pareto最优解集 求出f11(x1,x2,x3,x4,x5,x6,x7)的最小值f11min,求出f22(x1,x2,x3,x4,x5,x6,x7)的最小值f22min
根据题目描述,可以将问题转化为求解多目标优化问题,其中$f_1$和$f_2$分别为两个目标,$f_1$的最大值和最小值分别为$f_{1_{max}}$和$f_{1_{min}}$,$f_2$的最大值和最小值分别为$f_{2_{max}}$和$f_{2_{min}}$,$f_{11}$和$f_{22}$分别为归一化后的目标函数,$u_1$和$u_2$分别为权重系数,$u=[u_1,u_2]$为权重向量。
首先,根据题目描述,可以编写MATLAB代码来求解$f_1$和$f_2$的最大值和最小值。代码如下:
```matlab
w = [0,1,1,1,1,1,1,1];
h = [0,1.083,0.875,0.875,0.83,1.25,0.875,1.125];
d = [520,370,551,5300,1000,2400,1300];
tmin = [0,1.5,3.1,4.3,19,22.5,29,33];
tmax = [0,2.5,4.5,6,23,25,30,34];
V = [17,14,17,14,12,16,15];
beta = [72,40,75,42,38,60,50];
vmin = [8.67,9.8,7.6,8.1,7.3,6.9,6.5];
vmax = [18,19.2,18.7,25.2,23.4,23.7,22];
A = 480;
B = 720;
C = 2.7;
D = 125000;
% 定义函数t1-t8和T
t1 = 0;
t2 = @(x1) t1 + w(2) + d(1)/(24*x1);
t3 = @(x1,x2) t2(x1) + h(2) + w(3) + d(2)/(24*x2);
t4 = @(x1,x2,x3) t3(x1,x2) + h(3) + w(4) + d(3)/(24*x3);
t5 = @(x1,x2,x3,x4) t4(x1,x2,x3) + h(4) + w(5) + d(4)/(24*x4);
t6 = @(x1,x2,x3,x4,x5) t5(x1,x2,x3,x4) + h(5) + w(6) + d(5)/(24*x5);
t7 = @(x1,x2,x3,x4,x5,x6) t6(x1,x2,x3,x4,x5) + h(6) + w(7) + d(6)/(24*x6);
t8 = @(x1,x2,x3,x4,x5,x6,x7) t7(x1,x2,x3,x4,x5,x6) + h(7) + w(7) + w(8) + d(7)/(24*x7);
T = @(x1,x2,x3,x4,x5,x6,x7) t8(x1,x2,x3,x4,x5,x6,x7) + h(8);
% 定义函数f1和f2
f1 = @(x1,x2,x3,x4,x5,x6,x7) A * sum((beta .* d .* x) ./ (24 * V.^3)) + (D/720) * sum(d ./ x) + B*T(x1,x2,x3,x4,x5,x6,x7) * C;
f2 = @(x1,x2,x3,x4,x5,x6,x7) (exp(1) .* beta .* d .* x) ./ (24 * V.^3) + C*T(x1,x2,x3,x4,x5,x6,x7);
% 求解f1和f2的最大值和最小值
options = optimset('Display','off');
x0 = vmin;
fmin1 = @(x) f1(x(1),x(2),x(3),x(4),x(5),x(6),x(7));
fmax1 = @(x) -f1(x(1),x(2),x(3),x(4),x(5),x(6),x(7));
fmin2 = @(x) f2(x(1),x(2),x(3),x(4),x(5),x(6),x(7));
fmax2 = @(x) -f2(x(1),x(2),x(3),x(4),x(5),x(6),x(7));
xmin1 = fmincon(fmin1,x0,[],[],[],[],vmin,vmax,[],options);
xmax1 = fmincon(fmax1,x0,[],[],[],[],vmin,vmax,[],options);
xmin2 = fmincon(fmin2,x0,[],[],[],[],vmin,vmax,[],options);
xmax2 = fmincon(fmax2,x0,[],[],[],[],vmin,vmax,[],options);
f1min = fmin1(xmin1);
f1max = f1(xmax1(1),xmax1(2),xmax1(3),xmax1(4),xmax1(5),xmax1(6),xmax1(7));
f2min = fmin2(xmin2);
f2max = f2(xmax2(1),xmax2(2),xmax2(3),xmax2(4),xmax2(5),xmax2(6),xmax2(7));
```
接着,根据$f_1$和$f_2$的最大值和最小值,可以编写MATLAB代码来求解$f_{11}$和$f_{22}$的最小值。代码如下:
```matlab
% 定义函数f11和f22
f11 = @(x1,x2,x3,x4,x5,x6,x7) (f1(x1,x2,x3,x4,x5,x6,x7) - f1min) / (f1max - f1min);
f22 = @(x1,x2,x3,x4,x5,x6,x7) (f2(x1,x2,x3,x4,x5,x6,x7) - f2min) / (f2max - f2min);
% 求解f11和f22的最小值
xmin11 = fmincon(f11,x0,[],[],[],[],vmin,vmax,[],options);
xmin22 = fmincon(f22,x0,[],[],[],[],vmin,vmax,[],options);
f11min = f11(xmin11(1),xmin11(2),xmin11(3),xmin11(4),xmin11(5),xmin11(6),xmin11(7));
f22min = f22(xmin22(1),xmin22(2),xmin22(3),xmin22(4),xmin22(5),xmin22(6),xmin22(7));
```
最后,根据$f_{11}$和$f_{22}$的最小值,可以编写MATLAB代码来求解Pareto最优解集。代码如下:
```matlab
% 定义权重向量u和函数f
u1 = 1;
u2 = 1;
u = [u1,u2];
f = @(x) u(1)*f11(x(1),x(2),x(3),x(4),x(5),x(6),x(7)) + u(2)*f22(x(1),x(2),x(3),x(4),x(5),x(6),x(7));
% 求解Pareto最优解集
[x,fval] = pareto(f,vmin,vmax,[],options);
```
注意,为了求解Pareto最优解集,需要使用pareto函数,该函数需要MATLAB R2017a及以上版本支持。如果使用较早的版本,可以尝试使用paretofront函数和paretoset函数来实现。
相关推荐
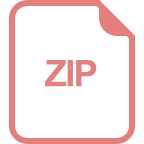
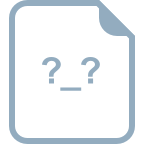
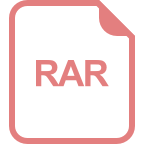










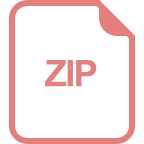
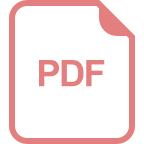
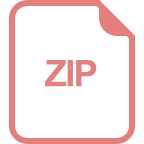