python中如何对excle中每一列按同样的高度进行插值,并绘制成图且输出
时间: 2024-03-21 12:37:45 浏览: 28
要对Excel中每一列进行插值并绘制成图,可以使用Python中的pandas和matplotlib库来实现。下面是一个简单的示例代码:
```python
import pandas as pd
import matplotlib.pyplot as plt
# 读取Excel文件
df = pd.read_excel('data.xlsx')
# 对每一列进行线性插值
df_interpolated = df.interpolate(method='linear', axis=0)
# 绘制每一列的图像
for col in df_interpolated.columns:
plt.plot(df_interpolated[col])
# 设置图像标题和坐标轴标签
plt.title('Data Interpolation and Plotting')
plt.xlabel('X Axis')
plt.ylabel('Y Axis')
# 显示图像
plt.show()
```
在这个示例中,我们首先使用pandas的read_excel函数读取Excel文件,然后使用interpolate函数对每一列进行线性插值。接着,我们使用matplotlib库的plot函数绘制每一列的图像。最后,我们设置图像的标题和坐标轴标签,并使用show函数显示图像。
需要注意的是,这个示例中的Excel文件必须包含数值数据,并且每一列的数据类型必须相同。如果需要对不同类型的数据进行插值和绘图,需要进行一些额外的处理。
相关问题
python中如何对excle中8列数据每一列按同样的高度进行插值,并绘制成图且输出
和上面的示例类似,对于8列数据,我们也可以使用pandas和matplotlib库来进行插值和绘图。下面是一个示例代码:
```python
import pandas as pd
import matplotlib.pyplot as plt
# 读取Excel文件
df = pd.read_excel('data.xlsx', usecols=[0, 1, 2, 3, 4, 5, 6, 7])
# 对每一列进行线性插值
df_interpolated = df.interpolate(method='linear', axis=0)
# 绘制每一列的图像
for col in df_interpolated.columns:
plt.plot(df_interpolated[col])
# 设置图像标题和坐标轴标签
plt.title('Data Interpolation and Plotting')
plt.xlabel('X Axis')
plt.ylabel('Y Axis')
# 显示图像
plt.show()
```
在这个示例中,我们使用了pandas的read_excel函数来读取Excel文件,并使用usecols参数指定了要读取的列。然后,我们使用interpolate函数对每一列进行线性插值,再使用matplotlib库的plot函数绘制每一列的图像。最后,我们设置图像的标题和坐标轴标签,并使用show函数显示图像。
需要注意的是,这个示例中的Excel文件必须包含8列数值数据,并且每一列的数据类型必须相同。如果需要对不同类型的数据进行插值和绘图,需要进行一些额外的处理。
在python语言中如何对excle表格里多列数据进行插值处理,并绘制成图、输出为excle
在Python中,可以使用 Pandas 库来读取 Excel 表格,使用 Scipy 库来进行插值处理,使用 Matplotlib 库来绘制图表,使用 Pandas 库来将数据输出到 Excel 表格中。
下面是一个简单的例子,演示如何对 Excel 表格中的多列数据进行插值处理,并绘制成图,并将插值后的数据输出到 Excel 表格中。
首先,我们需要安装 Pandas、Scipy 和 Matplotlib 库,可以使用以下命令进行安装:
```
pip install pandas scipy matplotlib
```
假设我们有一个 Excel 表格,文件名为 `data.xlsx`,包含两列数据 `x` 和 `y`,我们需要对这些数据进行插值处理并绘制成图,然后将插值后的数据输出到 Excel 表格 `output.xlsx` 中。
```python
import pandas as pd
import numpy as np
from scipy.interpolate import interp1d
import matplotlib.pyplot as plt
# 读取 Excel 表格
df = pd.read_excel('data.xlsx')
# 获取 x 和 y 列的数据
x = df['x'].values
y = df['y'].values
# 创建插值函数
f = interp1d(x, y, kind='cubic')
# 生成插值后的 x 和 y 数据
x_new = np.linspace(x.min(), x.max(), 1000)
y_new = f(x_new)
# 绘制图表
plt.plot(x, y, 'o', x_new, y_new, '-')
plt.legend(['data', 'cubic'], loc='best')
plt.xlabel('x')
plt.ylabel('y')
plt.title('Interpolation')
plt.show()
# 将插值后的数据输出到 Excel 表格
df_new = pd.DataFrame({'x': x_new, 'y': y_new})
df_new.to_excel('output.xlsx', index=False)
```
这个例子中,我们先使用 Pandas 库读取 Excel 表格 `data.xlsx`,然后获取 `x` 和 `y` 列的数据。接着,我们使用 Scipy 库中的 `interp1d` 函数创建了一个三次样条插值函数,并使用 `np.linspace` 函数生成了插值后的 `x` 数据。最后,我们使用 Matplotlib 库绘制了插值后的数据图表,使用 Pandas 库将插值后的数据输出到 Excel 表格 `output.xlsx` 中。
注意,在这个例子中,我们使用了三次样条插值函数,如果需要使用其他的插值方法,可以在 `interp1d` 函数的 `kind` 参数中指定插值方法。
相关推荐
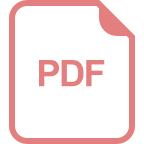
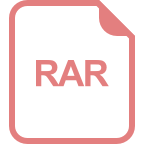








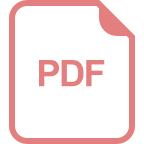
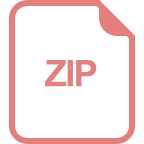
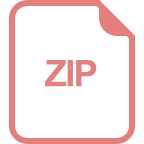
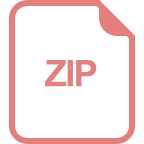