def build_model(max_features, maxlen): """Build LSTM model""" model = Sequential() model.add(Embedding(max_features, 128, input_length=maxlen)) model.add(LSTM(128)) model.add(Dropout(0.5)) model.add(Dense(1)) model.add(Activation('sigmoid')) # model.add(tf.keras.layers.BatchNormalization()) model.compile(loss='binary_crossentropy', optimizer='rmsprop') return model这个模型使用model.saveLayer ModuleWrapper was created by passing non-serializable argument values in `__init__()`, and therefore the layer must override `get_config()` in order to be serializable. Please implement `get_config()`.
时间: 2024-03-06 19:51:17 浏览: 118
这个错误是由于模型中的某个层不可序列化导致的。通常情况下,这是由于使用了自定义层或函数而导致的。要解决这个问题,你需要在自定义层类中实现`get_config()`方法,该方法返回一个字典,包含层的配置信息。例如:
```python
class MyLayer(Layer):
def __init__(self, **kwargs):
super(MyLayer, self).__init__(**kwargs)
def build(self, input_shape):
# build the layer
def call(self, inputs):
# implement the layer's forward pass
def get_config(self):
config = super(MyLayer, self).get_config()
# add layer-specific configuration
return config
```
如果你使用的是自定义函数,可以使用`@tf.function`装饰器将其转换为可序列化的图函数。例如:
```python
@tf.function
def my_custom_function(inputs):
# implement the function's logic
return outputs
```
通过这种方式,你可以确保模型中的所有层和函数都是可序列化的,从而能够成功保存模型。
阅读全文
相关推荐
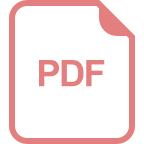
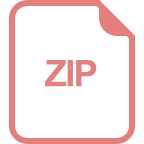
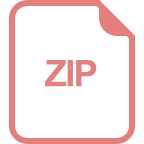








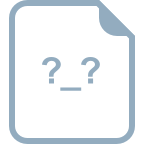
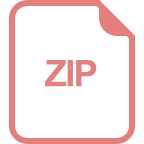
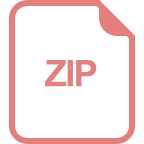
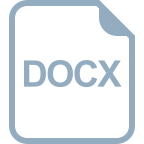
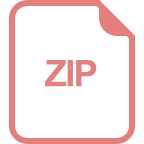
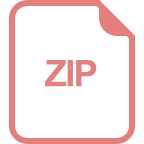