train_data = single(permute(reshape(train.data',32,32,3,[]),[2 1 3 4]));什么意思
时间: 2024-05-25 20:16:38 浏览: 106
这行代码将原始的训练数据进行了一些变换,具体解释如下:
1. `train.data'`:将原始训练数据的转置,变成每一行对应一张图片的像素值;
2. `reshape(...,32,32,3,[])`:将上一步得到的向量重新变形成一个四维数组,每个元素对应一张图片,维度为`(32,32,3,图片数量)`,其中`(32,32,3)`表示每张图片的长宽和通道数,`[]`表示最后一维由其他维度自动计算得出;
3. `permute(...,[2 1 3 4])`:将上一步得到的四维数组进行维度置换,将第1维和第2维交换,变成`(32,32,3,图片数量)`的形式。
4. `single(...)`:将上一步得到的数组转换成单精度浮点数类型,以便后续计算。
相关问题
train_data = single(permute(reshape(train.data',32,32,3,[]),[2 1 3 4]));
This line of code is reshaping the input data for a neural network.
The `train.data` variable is assumed to be a 4D array with dimensions `32 x 32 x 3 x N`, where `N` is the number of training examples.
The `reshape` function is used to reorder the dimensions of this array. It first transposes the array so that the third dimension becomes the first dimension, i.e. `train.data'` has dimensions `N x 3 x 32 x 32`. The `reshape` function then rearranges the dimensions so that the second dimension becomes the first dimension, the first dimension becomes the second dimension, and the third and fourth dimensions are combined into a single dimension.
The resulting array has dimensions `32 x 32 x 3 x N`, which is a more suitable format for feeding into a convolutional neural network (CNN), as it allows the network to treat each pixel in the image as a separate input channel.
Finally, the `single` function is used to convert the array to single-precision floating point values, which is a common data type used in CNNs.
Overall, this line of code is preparing the input data for a CNN by reshaping and converting the data to the appropriate format and data type.
data=xlsread('data_load'); % 按时间排序 load_data = sortrows(data, 1); % 生成训练集和测试集 train_ratio = 0.8; train_size = floor(train_ratio * size(load_data, 1)); train_data = load_data(1:train_size, 2:end); test_data = load_data(train_size+1:end, 2:end); % 数据归一化 train_data_norm = normalize(train_data); test_data_norm = normalize(test_data); % 准备训练数据 X_train = []; Y_train = []; n_steps = 3; % 每个时间步长包含的数据点数 for i = n_steps:size(train_data_norm, 1) X_train = [X_train; train_data_norm(i-n_steps+1:i, :)]; Y_train = [Y_train; train_data_norm(i, :)]; end % 调整训练数据的形状 X_train = permute(reshape(X_train', [], n_steps, size(X_train,1)), [3, 2, 1]); Y_train = permute(reshape(Y_train', [], n_steps, size(Y_train,1)), [3, 2, 1]); % 构建LSTM模型 input_size = size(train_data,2)-1; output_size = size(train_data,2)-1; num_hidden_units = 64; layers = [ ... sequenceInputLayer(input_size) lstmLayer(num_hidden_units,'OutputMode','last') fullyConnectedLayer(output_size) regressionLayer]; % 训练模型 opts = trainingOptions('adam', ... 'MaxEpochs',50, ... 'GradientThreshold',1, ... 'InitialLearnRate',0.01, ... 'LearnRateSchedule','piecewise', ... 'LearnRateDropFactor',0.1, ... 'LearnRateDropPeriod',30, ... 'Verbose',0, ... 'Plots','training-progress'); trained_net = trainNetwork(X_train, Y_train, layers, opts); % 准备测试数据 X_test = []; Y_test = []; for i = n_steps:size(test_data_norm, 1) X_test = [X_test; test_data_norm(i-n_steps+1:i, :)]; Y_test = [Y_test; test_data_norm(i, :)]; end % 调整测试数据的形状 X_test = reshape(X_test, [size(X_test,1), n_steps, size(test_data,2)-1]); Y_test = reshape(Y_test, [size(Y_test,1), size(test_data,2)-1]); % 进行预测 Y_pred = predict(trained_net, X_test); % 反归一化预测结果 Y_pred = Y_pred .* max(train_data) + min(train_data); Y_test = Y_test .* max(train_data) + min(train_data); % 绘制预测结果 figure plot(Y_test(:,1), 'b') hold on plot(Y_pred(:,1), 'r') legend('真实值', '预测值') title('负荷预测结果') xlabel('时间步长') ylabel('负荷值')
根据您提供的代码,出错的原因是您的输入数据的特征维度为 51,但是您的模型的输入层需要的特征维度为 2。这是因为您在将数据转换为训练数据时,使用了所有特征。您需要仅选择前两个特征作为输入,即时间和负荷值。您可以在以下代码中对 `train_data_norm` 进行修改,以仅选择前两个特征作为输入:
```
% 准备训练数据
X_train = [];
Y_train = [];
n_steps = 3; % 每个时间步长包含的数据点数
for i = n_steps:size(train_data_norm, 1)
X_train = [X_train; train_data_norm(i-n_steps+1:i, 1:2)]; % 只选择前两个特征作为输入
Y_train = [Y_train; train_data_norm(i, 1:2)];
end
```
这样可以确保您的输入数据的特征维度为 2,与您的模型的输入层所需的特征维度相匹配。
阅读全文
相关推荐
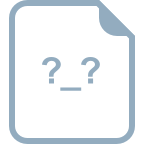












