如何用resnorm求以上函数的残差平方和
时间: 2024-02-17 15:59:56 浏览: 110
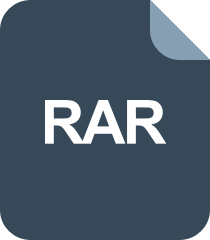
lsqcurvefitting.rar_curve fitting_lsqcurvefit函数_matlab lsqcurvef
可以使用 `polyfit` 函数的输出参数 `resnorm` 直接计算拟合函数的残差平方和,代码如下:
```matlab
% 拟合一次多项式
p1 = polyfit(x,y,1);
[yfit1, resnorm1] = polyval(p1,x,[],y);
SSresid1 = resnorm1;
% 拟合二次多项式
p2 = polyfit(x,y,2);
[yfit2, resnorm2] = polyval(p2,x,[],y);
SSresid2 = resnorm2;
% 拟合三次多项式
p3 = polyfit(x,y,3);
[yfit3, resnorm3] = polyval(p3,x,[],y);
SSresid3 = resnorm3;
```
其中,`resnorm1`、`resnorm2` 和 `resnorm3` 分别表示一次多项式、二次多项式和三次多项式的残差平方和。由于 `polyval` 函数有多个输出参数,我们可以通过指定第三个输入参数为 `[]`,并在第四个参数中传入原始数据 `y`,从而同时获取拟合函数的函数值和残差平方和。
阅读全文
相关推荐
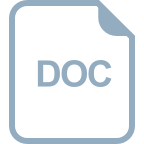
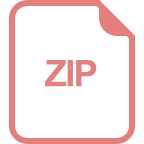
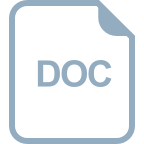
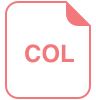
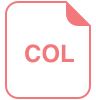












