from tensorflow.keras.layers import Input from tensorflow.keras.models import Model from tcn import TCN # 定义输入数据的shape input_shape = (None, 10) # 定义模型的输入层 inputs = Input(shape=input_shape) # 定义TCN网络的中间层,可以设置多个卷积层和池化层 tcn_layer = TCN(nb_filters=4, kernel_size=3, dilations=[1, 2, 4, 8, 16])(inputs) # 定义模型,将输入层和TCN网络的中间层连接起来 model = Model(inputs=inputs, outputs=tcn_layer) # 使用predict函数获取特征,将数据分成多个batch进行处理 batch_size = 32 num_samples = x_data.shape[0] features = [] for i in range(0, num_samples, batch_size): batch = x_data[i:i + batch_size] feature_batch = model.predict(batch) features.append(feature_batch) # 将多个batch的特征拼接成一个大的特征矩阵 features = np.concatenate(features, axis=0)这段代码样本数量不能整除以批次怎么办
时间: 2024-03-23 20:40:29 浏览: 17
如果样本数量不能整除以批次,最后一个batch的样本数量可能会小于batch_size。在这种情况下,你可以在循环的最后一次迭代中,将batch_size设置为剩余的样本数量,并将其传递给predict函数。如下所示:
```
batch_size = 32
num_samples = x_data.shape[0]
features = []
for i in range(0, num_samples, batch_size):
batch = x_data[i:i + batch_size]
if i + batch_size > num_samples:
batch_size = num_samples - i
feature_batch = model.predict(batch, batch_size=batch_size)
features.append(feature_batch)
features = np.concatenate(features, axis=0)
```
这样,最后一个batch的样本数量会被动态地设置。
相关问题
input_2 = keras.Input(shape=(160,)) x = keras.layers.LayerNormalization()(input_2) x = keras.layers.Reshape((160, 1))(x) x = Conv1D(filters=16, kernel_size=12, strides=4, padding='causal')(x) x = tf.keras.layers.Dropout(0.4)(x) x = tcnBlock(x, 12, 3, 1) x = tcnBlock(x, 6, 3, 2) x = tcnBlock(x, 4, 3, 4) x = GlobalAveragePooling1D()(x) x = keras.layers.LayerNormalization()(x) output_2 = keras.layers.Dense(1, activation='sigmoid')(x) model2 = keras.Model(inputs=input_2, outputs=output_2) model2.summary()这段代码正确吗
这段代码是有问题的。
在这段代码中,定义了一个包含多个TCN块(`tcnBlock`)的一维卷积神经网络模型。但是,这个模型的输出层是一个单个神经元,输出一个标量值。这意味着,这个模型是用来处理二分类问题的,需要对每个输入样本输出一个二元分类结果。
然而,在这个模型中,输入层的形状是`(160,)`,即一维的长度为160的向量,这个形状是不正确的。因为在模型中使用了一维卷积层,需要将输入转化为三维张量,其中第一维表示时间步长,第二维表示输入特征的数量,第三维表示通道数。所以需要将输入张量的形状修改为`(160, 1)`。
此外,在这个模型中,`tcnBlock`的参数数量也需要进行修改。在`tcnBlock`的定义中,第一个参数`incoming`表示输入张量,应该是一个三维张量。但是在这个模型中,输入张量的通道数是1,因此需要修改`tcnBlock`的定义,将其输入张量的通道数设置为1。
最后,在这个模型中,`keras.layers.LayerNormalization`和`keras.layers.Reshape`等层的导入方式有问题,应该使用`from tensorflow.keras.layers import LayerNormalization, Reshape`的方式导入。
下面是修正后的代码:
```
from tensorflow.keras.layers import Input, LayerNormalization, Reshape, Conv1D, GlobalAveragePooling1D, Dropout, Dense
from tensorflow.keras import regularizers
def tcnBlock(incoming, filters, kernel_size, dilation_rate):
net = incoming
identity = incoming
net = LayerNormalization()(net)
net = keras.layers.LeakyReLU(alpha=0.2)(net)
net = Dropout(0.3)(net)
net = Conv1D(filters, kernel_size, padding='causal', dilation_rate=dilation_rate, kernel_regularizer=regularizers.l2(1e-3))(net)
if identity.shape[-1] == filters:
shortcut = identity
else:
shortcut = Conv1D(filters, kernel_size, padding='same')(identity)
net = keras.layers.add([net, shortcut])
return net
input_2 = Input(shape=(160, 1))
x = LayerNormalization()(input_2)
x = Conv1D(filters=16, kernel_size=12, strides=4, padding='causal')(x)
x = Dropout(0.4)(x)
x = tcnBlock(x, 16, 3, 1)
x = tcnBlock(x, 8, 3, 2)
x = tcnBlock(x, 4, 3, 4)
x = GlobalAveragePooling1D()(x)
x = LayerNormalization()(x)
output_2 = Dense(1, activation='sigmoid')(x)
model2 = keras.Model(inputs=input_2, outputs=output_2)
model2.summary()
```
input_2 = keras.Input(shape=(176,)) x = keras.layers.Reshape((-1, 176, 1))(input_2) x = keras.layers.LayerNormalization()(x) x = Conv1D(filters=16, kernel_size=12, strides=4, padding='causal')(x) x = tf.keras.layers.Dropout(0.4)(x) x = tcnBlock(x, 16, 3, 1) x = tcnBlock(x, 8, 3, 2) x = tcnBlock(x, 4, 3, 4) x = GlobalAveragePooling1D()(x) x = keras.layers.LayerNormalization()(x) output_2 = keras.layers.Dense(1, activation='sigmoid')(x) model2 = keras.Model(inputs=input_2, outputs=output_2) model2.summary()结合上边的自定义,这段代码有什么问题
这段代码的结构看起来没有问题,但是需要注意以下几点:
1. 没有导入 `Conv1D` 和 `GlobalAveragePooling1D`,需要在代码开头加上 `from tensorflow.keras.layers import Conv1D, GlobalAveragePooling1D`。
2. 在第 3 行中,`Reshape` 层的参数应该是 `(176, 1)` 而不是 `(-1, 176, 1)`,因为 `-1` 表示这个维度的大小由其他维度推断出来,但在这里不能推断出来。
3. 在第 6 行中,`tf.keras.layers.Dropout` 应该改为 `keras.layers.Dropout`,因为前面已经使用了 `keras` 的命名空间。
4. 在第 7 行中,`tcnBlock` 是自定义的函数,需要检查该函数的实现是否正确并且已经导入。
5. 在第 13 行中,`keras.layers.LayerNormalization` 应该传入 `axis=-1`,以便在最后一个轴上进行归一化。
总体来说,这段代码可能是一个用于进行时间序列分类或回归的模型,使用了 TCN (Temporal Convolutional Networks) 的结构,通过 `Conv1D` 层捕捉时间序列中的局部模式,通过 `tcnBlock` 层进行多层次的时间特征提取,最终通过全局平均池化和 `Dense` 层进行分类或回归。
相关推荐
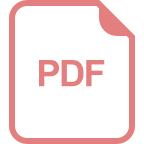
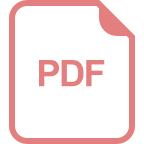
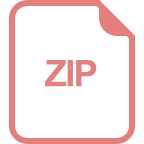













