在pycharm里运行的神经网络代码
时间: 2024-04-06 12:33:27 浏览: 267
好的,我可以回答这个问题。在PyCharm中运行神经网络代码,通常需要以下步骤:
1. 安装必要的依赖库:例如TensorFlow、Keras、PyTorch等。
2. 导入所需的库和模块:例如numpy、pandas等用于数据处理的库,以及tensorflow、keras等用于神经网络的库。
3. 准备数据:包括数据预处理、数据划分等。
4. 构建模型:选择合适的神经网络模型,并进行模型的搭建和训练。
5. 进行模型测试:使用测试数据集对模型进行测试,并评估模型的性能。
6. 进行模型优化:根据测试结果对模型进行优化,例如调整超参数等。
以上是一般的步骤,具体需要根据实际情况进行调整。在PyCharm中运行代码,可以使用快捷键Shift+F10或者点击运行按钮来执行代码。
相关问题
利用pycharm根据卷积神经网络识别手写字母运行一个代码
PyCharm是一个强大的Python集成开发环境,用于构建基于卷积神经网络(Convolutional Neural Network, CNN)的手写字母识别项目,你可以按照以下步骤来编写并运行代码:
1. **安装必要的库**:
- 首先,你需要安装TensorFlow或PyTorch等深度学习框架,以及像Keras这样的高级API,因为它们提供了预定义的CNN模型和便利的功能。
```
pip install tensorflow keras
```
2. **数据预处理**:
导入MNIST数据集,这是常用于手写数字识别的标准数据集,包含了训练和测试样本。
```python
from tensorflow.keras.datasets import mnist
(train_data, train_labels), (test_data, test_labels) = mnist.load_data()
```
3. **图像预处理**:
对像素值归一化,并将图像转换为适合输入CNN的维度。
```python
train_data, test_data = train_data / 255.0, test_data / 255.0
train_data = train_data.reshape(-1, 28, 28, 1)
test_data = test_data.reshape(-1, 28, 28, 1)
```
4. **构建CNN模型**:
使用Keras创建一个简单的CNN模型,包括卷积层、池化层和全连接层。
```python
model = tf.keras.Sequential([
tf.keras.layers.Conv2D(32, (3, 3), activation='relu', input_shape=(28, 28, 1)),
tf.keras.layers.MaxPooling2D((2, 2)),
tf.keras.layers.Flatten(),
tf.keras.layers.Dense(128, activation='relu'),
tf.keras.layers.Dense(10, activation='softmax') # 输出层,10代表0-9十个数字
])
```
5. **编译模型**:
设置损失函数、优化器和评估指标。
```python
model.compile(optimizer='adam',
loss=tf.keras.losses.SparseCategoricalCrossentropy(from_logits=True),
metrics=['accuracy'])
```
6. **训练模型**:
将训练数据拟合到模型上。
```python
model.fit(train_data, train_labels, epochs=10, validation_split=0.1)
```
7. **评估模型**:
测试模型在测试集上的性能。
```python
test_loss, test_acc = model.evaluate(test_data, test_labels, verbose=2)
print(f'Test accuracy: {test_acc}')
```
8. **预测**:
最后,你可以用模型对新的手写字母图片进行分类。
```python
predictions = model.predict(new_image.reshape(1, 28, 28, 1))
predicted_label = np.argmax(predictions)
print(f"Predicted label: {predicted_label}")
```
记得在实际操作中替换`new_image`为你想要识别的实际手写字体图片。
pycharm 实现bp神经网络
PyCharm 是一款强大的 Python IDE,它提供了很多方便的功能和工具,使得我们能够更加轻松地实现神经网络的开发。
实现一个基本的 BP 神经网络需要以下步骤:
1. 导入相关的库,如 numpy、matplotlib 等。
2. 定义神经网络的结构,包括输入层、隐藏层和输出层的神经元数量。
3. 初始化权重和偏置,一般采用随机值初始化。
4. 定义激活函数,如 sigmoid、ReLU 等。
5. 定义损失函数,如均方误差(MSE)等。
6. 进行前向传播计算,计算输出值。
7. 计算损失函数值。
8. 进行反向传播计算,更新权重和偏置。
9. 循环以上步骤直到收敛。
在 PyCharm 中实现 BP 神经网络可以按照以下步骤:
1. 创建一个 Python 项目,并将需要的库导入到项目中。
2. 在项目中创建一个 Python 文件,并编写 BP 神经网络的代码。可以使用 PyCharm 提供的代码提示和自动补全功能来提高编写效率。
3. 运行代码并查看结果。可以使用 PyCharm 提供的调试功能来帮助调试代码。
阅读全文
相关推荐
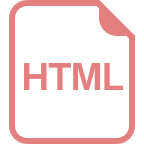







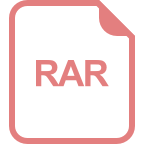
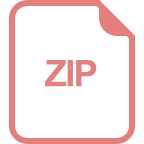





