使用轮廓系数评估st-dbscan聚类的Python代码
时间: 2024-05-10 12:14:24 浏览: 120
以下是使用轮廓系数评估ST-DBSCAN聚类的Python代码:
```
from sklearn.metrics import silhouette_score
from stdbscan import STDBSCAN
# load data
data = ...
# initialize ST-DBSCAN
stdbscan = STDBSCAN(eps1=..., eps2=..., t=...)
# fit the model and obtain cluster labels
labels = stdbscan.fit_predict(data)
# evaluate clustering using silhouette score
score = silhouette_score(data, labels)
print('Silhouette Score:', score)
```
在此代码中,需要将数据加载到`data`变量中,并根据数据集的特征进行 ST-DBSCAN 参数的初始化。然后,使用`fit_predict()`方法获取聚类标签,最后使用`silhouette_score()`函数计算轮廓系数评分。
相关问题
计算Silhouette系数评估st-dbscan聚类效果
Silhouette系数是一种常用的聚类效果评估指标,可以用来评估st-dbscan聚类的效果。具体计算步骤如下:
1. 对于每个样本i,计算它与同簇内其他样本的平均距离ai,以及它与最近簇内其他样本的平均距离bi。
2. 定义样本i的Silhouette系数为:(bi-ai)/max(ai,bi)。
3. 对于所有样本的Silhouette系数求平均值,即为聚类的整体Silhouette系数。
其中,Silhouette系数的取值范围在[-1,1]之间,越接近1表示聚类效果越好,越接近-1表示聚类效果越差,0表示聚类效果一般。
下面是一个使用Python计算st-dbscan聚类效果的Silhouette系数的示例代码:
``` python
from sklearn.metrics import silhouette_score
from sklearn.cluster import DBSCAN
import numpy as np
# 假设已经对数据进行了st-dbscan聚类,并得到了每个样本的簇标签labels和距离矩阵distances
# 将簇标签转换为整数类型
labels = [int(label) for label in labels]
# 计算Silhouette系数
silhouette_avg = silhouette_score(distances, labels)
print("st-dbscan聚类效果的Silhouette系数为:", silhouette_avg)
```
其中,distances是样本之间的距离矩阵,labels是每个样本的簇标签。需要注意的是,距离矩阵和簇标签需要与使用st-dbscan聚类算法时的输入保持一致。
st-dbscan聚类算法用代码实现
下面是st-dbscan聚类算法的Python代码实现:
```python
import numpy as np
from scipy.spatial.distance import pdist, squareform
def stdbscan(data, eps, min_pts):
"""
ST-DBSCAN algorithm implementation.
Parameters:
data (ndarray): Input data.
eps (float): The maximum distance between two samples for them to be considered as in the same neighborhood.
min_pts (int): The number of samples (or total weight) in a neighborhood for a point to be considered as a core point.
Returns:
labels (ndarray): Cluster labels for each point. -1 for noise points.
"""
# Compute pairwise distance matrix
dist_mat = squareform(pdist(data))
# Initialize variables
num_pts = data.shape[0]
visited = np.zeros(num_pts, dtype=bool)
labels = np.zeros(num_pts, dtype=int)
cluster_id = 0
# Iterate over all points
for i in range(num_pts):
if not visited[i]:
visited[i] = True
# Get neighbors within eps distance
neighbors = np.where(dist_mat[i] <= eps)[0]
# Check if there are enough neighbors
if len(neighbors) < min_pts:
labels[i] = -1 # Noise point
else:
cluster_id += 1
labels[i] = cluster_id
# Expand cluster
j = 0
while j < len(neighbors):
neighbor = neighbors[j]
if not visited[neighbor]:
visited[neighbor] = True
# Get neighbors within eps distance
new_neighbors = np.where(dist_mat[neighbor] <= eps)[0]
# Check if there are enough neighbors
if len(new_neighbors) >= min_pts:
neighbors = np.concatenate((neighbors, new_neighbors))
# Assign to cluster
if labels[neighbor] == 0:
labels[neighbor] = cluster_id
j += 1
return labels
```
其中,`data`是输入数据,`eps`是最大距离阈值(即点与点之间的距离超过该值则不再属于同一簇),`min_pts`是最小密度阈值(即点周围的其它点个数不足该值时不再属于核心点)。函数返回每个点的聚类标签,-1表示噪声点。
阅读全文
相关推荐
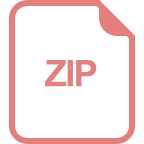
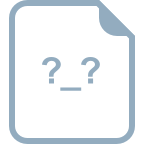
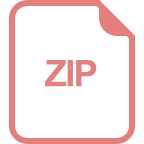













