theta = np.zeros(10)
时间: 2023-12-03 19:02:36 浏览: 118
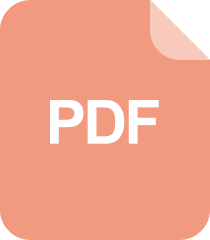
1183710113-许健-Lab3-Report1
`theta = np.zeros(10)` 是一个 Python 代码行,它创建了一个长度为 10 的一维数组 `theta`,并将其所有元素初始化为 0。
这个代码行使用了 Python 中的 NumPy 库,NumPy 是一个重要的 Python 库,它提供了高效的多维数组和矩阵运算功能。在这个代码行中,`np` 是 NumPy 库的别名,它是通过 `import numpy as np` 语句进行定义的。
`np.zeros(10)` 表示创建一个长度为 10 的一维数组,其中所有元素都是 0。这个函数的用法非常灵活,可以创建不同维度和不同元素类型的数组。例如,`np.zeros((3, 4))` 表示创建一个 3 行 4 列的二维数组,其中所有元素都是 0。
在在线学习中,我们通常需要定义一个参数向量 `theta` 来表示模型的参数,然后使用不断的更新来优化这个参数向量。在开始的时候,我们通常会将这个参数向量初始化为一些默认值,例如全零向量。在这个代码行中,我们使用了 `np.zeros(10)` 来创建一个长度为 10 的全零向量,作为模型参数的初始值。
阅读全文
相关推荐
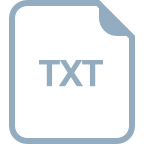















