我有一个dataframe,有两个columns,code和count,我需要by code去统计每个code对应的count栏位的数值加总,生成一个新dataframe
时间: 2024-03-10 16:44:26 浏览: 157
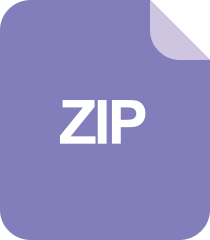
1、python exe打包资源,直接运行 2、对多个Excel文件中的某个sheet进行合并 3、python\excl
好的,你可以使用`groupby()`和`sum()`方法来实现这个需求。以下是示例代码:
```python
import pandas as pd
# 假设你的原始数据存储在df变量中
# 创建一个新的DataFrame,按code分组并计算count列的总和
new_df = pd.DataFrame(df.groupby('code')['count'].sum())
# 输出新的DataFrame
print(new_df)
```
这段代码会按照`code`列进行分组,并计算每个组中`count`列的总和,最终生成一个新的DataFrame。你可以将代码中的`df`替换为你实际使用的DataFrame对象。
阅读全文
相关推荐
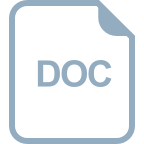
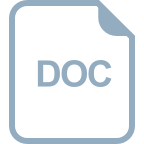
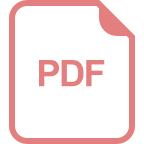
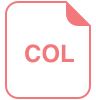
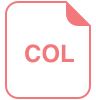
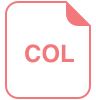
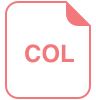










