x = tf.placeholder('float', shape=[None, image_size])
时间: 2023-05-11 18:01:58 浏览: 46
这是一个 TensorFlow 中的占位符,用于在运行时传递输入数据。其中,'float' 表示数据类型为浮点数,shape=[None, image_size] 表示输入数据的形状为一个二维数组,第一维可以是任意长度,第二维长度为 image_size。
相关问题
input_image = tf.placeholder("float", [None, 80, 100, 4]) # 游戏像素 action = tf.placeholder("float", [None, output]) # 操作用TensorFlow2代码改写
在TensorFlow 2中,可以使用`tf.keras.Input`创建输入占位符,例如:
```
import tensorflow as tf
# 创建输入占位符
input_image = tf.keras.Input(shape=(80, 100, 4), dtype=tf.float32)
action = tf.keras.Input(shape=(output,), dtype=tf.float32)
```
这里使用`tf.keras.Input`函数来创建输入占位符,其中`shape`参数指定输入的形状,`dtype`参数指定输入的数据类型。注意,这里的输入占位符不再是`tf.placeholder`类型,而是`tf.Tensor`类型。
在使用这些占位符进行计算时,可以直接将它们传递给`tf.keras.Model`的`__call__`方法,例如:
```
import tensorflow as tf
# 创建输入占位符
input_image = tf.keras.Input(shape=(80, 100, 4), dtype=tf.float32)
action = tf.keras.Input(shape=(output,), dtype=tf.float32)
# 构建模型
x = tf.keras.layers.Conv2D(filters=32, kernel_size=3)(input_image)
x = tf.keras.layers.MaxPooling2D()(x)
x = tf.keras.layers.Flatten()(x)
x = tf.keras.layers.Dense(units=64, activation='relu')(x)
output = tf.keras.layers.Dense(units=output, activation='softmax')(x)
model = tf.keras.Model(inputs=[input_image, action], outputs=output)
# 使用输入占位符进行计算
input_image_data = tf.ones((32, 80, 100, 4))
action_data = tf.ones((32, output))
output_data = model([input_image_data, action_data])
```
这里使用`Model`类构建一个简单的神经网络模型,并将输入和输出占位符作为构造函数的参数传递给模型。然后,可以使用`model`对象调用模型进行计算,传递输入数据即可。
tf.nn.conv2d
tf.nn.conv2d is a function in TensorFlow that performs a 2D convolution operation on a given input tensor and a set of filters. It is typically used in deep learning models for image processing and computer vision tasks.
The function takes several arguments, including the input tensor, the filter tensor, the strides for the convolution operation, and the padding scheme. The output of the convolution operation is a new tensor that represents the result of applying the filters to the input tensor.
Here is an example usage of tf.nn.conv2d:
```
import tensorflow as tf
# Define input and filter tensors
input_tensor = tf.placeholder(tf.float32, shape=[None, 28, 28, 3])
filter_tensor = tf.Variable(tf.random_normal([5, 5, 3, 32]))
# Perform a 2D convolution operation with strides of 1 and padding of 'SAME'
conv = tf.nn.conv2d(input_tensor, filter_tensor, strides=[1, 1, 1, 1], padding='SAME')
# Run the convolution operation within a TensorFlow session
with tf.Session() as sess:
sess.run(tf.global_variables_initializer())
# Define a sample input tensor
input_data = np.random.rand(1, 28, 28, 3)
# Run the convolution operation on the input tensor
conv_result = sess.run(conv, feed_dict={input_tensor: input_data})
```
In this example, we define an input tensor with a shape of (None, 28, 28, 3), which represents a batch of 28x28 RGB images. We also define a filter tensor with a shape of (5, 5, 3, 32), which represents 32 5x5 filters that will be applied to the input tensor.
We then call tf.nn.conv2d with the input and filter tensors, specifying a stride of 1 and a padding scheme of 'SAME'. This means that the output tensor will have the same spatial dimensions as the input tensor, and that the edges of the input tensor will be zero-padded to ensure that the filters can be applied to all pixels.
Finally, we run the convolution operation within a TensorFlow session, providing a sample input tensor to test the operation. The resulting conv_result tensor will have a shape of (1, 28, 28, 32), representing a batch of 28x28 feature maps for each of the 32 filters applied to the input tensor.
相关推荐
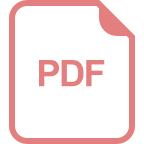
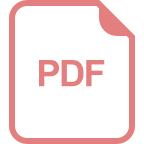












