plt.plot(J_history) plt.xlabel("Iteration") plt.ylabel("$J(\Theta)$") plt.title("Cost function using Gradient Descent")
时间: 2024-01-03 22:05:10 浏览: 26
这段代码使用了 Python 中的 Matplotlib 库来绘制梯度下降算法的损失函数 J(Theta) 随迭代次数的变化曲线图。具体来说,plt.plot(J_history) 语句将 J_history 中保存的每次迭代的损失函数值绘制在图像上,plt.xlabel("Iteration") 和 plt.ylabel("$J(\\Theta)$") 分别设置了 X 轴和 Y 轴的标签,plt.title("Cost function using Gradient Descent") 则设置了图像的标题。注意到 $J(\Theta)$ 使用了 Latex 语法,通过转义符号 \\ 可以将 $ 字符正确地显示在图像上。
相关问题
plt.plot(J_history2) plt.xlabel("Iteration") plt.ylabel("$J(\Theta)$") plt.title("Cost function using Gradient Descent")
这段代码使用matplotlib库绘制了梯度下降算法迭代过程中代价函数的变化情况。具体来说,plt.plot(J_history2)表示将J_history2中的数据点连接起来,生成折线图;plt.xlabel("Iteration")和plt.ylabel("$J(\Theta)$")分别设置x轴和y轴的标签;plt.title("Cost function using Gradient Descent")设置图表的标题。
import matplotlib.pyplot as plt from scipy.stats import norm X = df.iloc[:, 4:] # 特征数据 X = scaler.fit_transform(X) y_1 = df[['U(Ⅳ)浓度']] # 目标变量1 y_2 = df[['U(Ⅵ)浓度']] # 目标变量2) # 添加偏置项 x0=1 到 X 中 X_b = np.c_[np.ones((X.shape[0], 1)), X] # 初始化学习率,迭代次数和初始参数 eta = 0.1 n_iterations = 1000 theta = np.random.randn(2, 1) # 定义似然函数计算梯度 def likelihood(theta, X, y): m = y.size h = X.dot(theta).flatten() mu = h sigma = 1 # 假设高斯噪声的标准差是1 p = norm(mu, sigma).pdf(y.flatten()) L = np.prod(p) return L # 定义损失函数计算梯度 def loss(theta, X, y): m = y.size h = X.dot(theta).flatten() mu = h sigma = 1 # 假设高斯噪声的标准差是1 p = norm(mu, sigma).pdf(y.flatten()) J = -np.sum(np.log(p)) return J/m # 批量梯度下降算法 for iteration in range(n_iterations): gradients = 2/X_b.shape[0] * X_b.T.dot(X_b.dot(theta) - y) theta = theta - eta * gradients if iteration % 100 == 0: print(f"Iteration {iteration}: theta={theta.flatten()}, likelihood={likelihood(theta, X_b, y)}, loss={loss(theta, X_b, y)}") # 绘制数据和回归直线 plt.plot(X, y, "b.") X_new = np.array([[0], [10]]) X_new_b = np.c_[np.ones((2, 1)), X_new] y_predict = X_new_b.dot(theta) plt.plot(X_new, y_predict, "r-", linewidth=2, label="Predictions") plt.xlabel("X") plt.ylabel("y") plt.legend() plt.show()
这段代码是一个简单的线性回归模型,使用了批量梯度下降法来拟合数据。具体来说,它首先进行了特征数据的标准化处理,然后定义了似然函数和损失函数来计算梯度,最后使用批量梯度下降法来更新参数并拟合数据。在训练过程中,它每迭代100次就会输出当前参数、似然函数和损失函数的值。最后,它将拟合出的回归直线和原始数据一起绘制在图上,以便进行可视化比较。
相关推荐
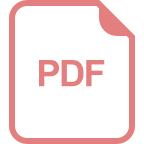











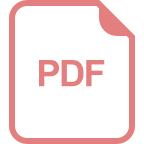
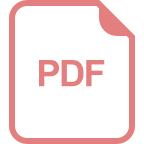