代码解释:x_train = train_set[:, 0:2]
时间: 2024-03-27 15:40:10 浏览: 13
这段代码假设train_set是一个二维数组,其中每一行表示一个数据样本,第一列和第二列分别表示该样本的两个特征。例如,train_set可以长这样:
```
train_set = [[1, 2, 3],
[4, 5, 6],
[7, 8, 9]]
```
在这个例子中,train_set有三个样本,每个样本有三个特征。
接下来,代码中的`[:, 0:2]`表示对train_set数组进行切片操作。`:`表示对所有行进行操作,`0:2`表示对第0列和第1列进行操作,即选取train_set数组中的前两列作为特征。因此,x_train将会是一个二维数组,其中每一行表示一个样本,只包含train_set中的前两个特征。例如:
```
x_train = [[1, 2],
[4, 5],
[7, 8]]
```
这样做的目的是将数据集中的特征提取出来,以便在训练模型时使用。通常情况下,我们将数据集分成特征和标签两个部分,特征被用来训练模型,标签被用来评估模型的性能。
相关问题
代码解释:x_train = train_set[:, 0:2] y_train = train_set[:, 2]
这段代码是将数据集train_set中的特征和标签分开存储。假设train_set是一个二维数组,其中每一行表示一个数据样本,第一列和第二列分别表示该样本的两个特征,第三列表示该样本的标签。例如,train_set可以长这样:
```
train_set = [[1, 2, 0],
[4, 5, 1],
[7, 8, 0]]
```
在这个例子中,train_set有三个样本,每个样本有两个特征和一个标签。
接下来,代码中的`[:, 0:2]`表示对train_set数组进行切片操作,选取所有行和前两列(即特征),将它们存储在x_train中。因此,x_train将会是一个二维数组,其中每一行表示一个样本,只包含train_set中的前两个特征。例如:
```
x_train = [[1, 2],
[4, 5],
[7, 8]]
```
然后,代码中的`[:, 2]`表示对train_set数组进行切片操作,选取所有行和第三列(即标签),将它们存储在y_train中。因此,y_train将会是一个一维数组,其中每个元素表示一个样本的标签。例如:
```
y_train = [0, 1, 0]
```
这样做的目的是将数据集分成特征和标签两个部分,特征被用来训练模型,标签被用来评估模型的性能。
帮我为下面的代码加上注释:class SimpleDeepForest: def __init__(self, n_layers): self.n_layers = n_layers self.forest_layers = [] def fit(self, X, y): X_train = X for _ in range(self.n_layers): clf = RandomForestClassifier() clf.fit(X_train, y) self.forest_layers.append(clf) X_train = np.concatenate((X_train, clf.predict_proba(X_train)), axis=1) return self def predict(self, X): X_test = X for i in range(self.n_layers): X_test = np.concatenate((X_test, self.forest_layers[i].predict_proba(X_test)), axis=1) return self.forest_layers[-1].predict(X_test[:, :-2]) # 1. 提取序列特征(如:GC-content、序列长度等) def extract_features(fasta_file): features = [] for record in SeqIO.parse(fasta_file, "fasta"): seq = record.seq gc_content = (seq.count("G") + seq.count("C")) / len(seq) seq_len = len(seq) features.append([gc_content, seq_len]) return np.array(features) # 2. 读取相互作用数据并创建数据集 def create_dataset(rna_features, protein_features, label_file): labels = pd.read_csv(label_file, index_col=0) X = [] y = [] for i in range(labels.shape[0]): for j in range(labels.shape[1]): X.append(np.concatenate([rna_features[i], protein_features[j]])) y.append(labels.iloc[i, j]) return np.array(X), np.array(y) # 3. 调用SimpleDeepForest分类器 def optimize_deepforest(X, y): X_train, X_test, y_train, y_test = train_test_split(X, y, test_size=0.2) model = SimpleDeepForest(n_layers=3) model.fit(X_train, y_train) y_pred = model.predict(X_test) print(classification_report(y_test, y_pred)) # 4. 主函数 def main(): rna_fasta = "RNA.fasta" protein_fasta = "pro.fasta" label_file = "label.csv" rna_features = extract_features(rna_fasta) protein_features = extract_features(protein_fasta) X, y = create_dataset(rna_features, protein_features, label_file) optimize_deepforest(X, y) if __name__ == "__main__": main()
# Define a class named 'SimpleDeepForest'
class SimpleDeepForest:
# Initialize the class with 'n_layers' parameter
def __init__(self, n_layers):
self.n_layers = n_layers
self.forest_layers = []
# Define a method named 'fit' to fit the dataset into the classifier
def fit(self, X, y):
X_train = X
# Use the forest classifier to fit the dataset for 'n_layers' times
for _ in range(self.n_layers):
clf = RandomForestClassifier()
clf.fit(X_train, y)
# Append the classifier to the list of forest layers
self.forest_layers.append(clf)
# Concatenate the training data with the predicted probability of the last layer
X_train = np.concatenate((X_train, clf.predict_proba(X_train)), axis=1)
# Return the classifier
return self
# Define a method named 'predict' to make predictions on the test set
def predict(self, X):
X_test = X
# Concatenate the test data with the predicted probability of each layer
for i in range(self.n_layers):
X_test = np.concatenate((X_test, self.forest_layers[i].predict_proba(X_test)), axis=1)
# Return the predictions of the last layer
return self.forest_layers[-1].predict(X_test[:, :-2])
# Define a function named 'extract_features' to extract sequence features
def extract_features(fasta_file):
features = []
# Parse the fasta file to extract sequence features
for record in SeqIO.parse(fasta_file, "fasta"):
seq = record.seq
gc_content = (seq.count("G") + seq.count("C")) / len(seq)
seq_len = len(seq)
features.append([gc_content, seq_len])
# Return the array of features
return np.array(features)
# Define a function named 'create_dataset' to create the dataset
def create_dataset(rna_features, protein_features, label_file):
labels = pd.read_csv(label_file, index_col=0)
X = []
y = []
# Create the dataset by concatenating the RNA and protein features
for i in range(labels.shape[0]):
for j in range(labels.shape[1]):
X.append(np.concatenate([rna_features[i], protein_features[j]]))
y.append(labels.iloc[i, j])
# Return the array of features and the array of labels
return np.array(X), np.array(y)
# Define a function named 'optimize_deepforest' to optimize the deep forest classifier
def optimize_deepforest(X, y):
# Split the dataset into training set and testing set
X_train, X_test, y_train, y_test = train_test_split(X, y, test_size=0.2)
# Create an instance of the SimpleDeepForest classifier with 3 layers
model = SimpleDeepForest(n_layers=3)
# Fit the training set into the classifier
model.fit(X_train, y_train)
# Make predictions on the testing set
y_pred = model.predict(X_test)
# Print the classification report
print(classification_report(y_test, y_pred))
# Define the main function to run the program
def main():
rna_fasta = "RNA.fasta"
protein_fasta = "pro.fasta"
label_file = "label.csv"
# Extract the RNA and protein features
rna_features = extract_features(rna_fasta)
protein_features = extract_features(protein_fasta)
# Create the dataset
X, y = create_dataset(rna_features, protein_features, label_file)
# Optimize the DeepForest classifier
optimize_deepforest(X, y)
# Check if the program is being run as the main program
if __name__ == "__main__":
main()
相关推荐
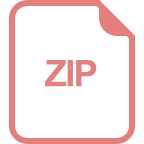
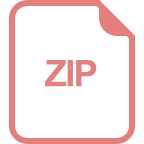













