y = np.concatenate([np.ones(len(X_processed)*len(X_processed[0])), np.zeros(len(X_masked)*len(X_masked[0]))]) print(y.shape) X_features = [] for x_list in X_processed: for x in x_list: x_feature = ft.hog(x, orientations=8, pixels_per_cell=(10, 10), cells_per_block=(1, 1), visualize=False) X_features.append(x_feature) for x_list in X_masked: for x in x_list: x_feature = ft.hog(x, orientations=8, pixels_per_cell=(10, 10), cells_per_block=(1, 1), visualize=False) X_features.append(x_feature) # write code to split the dataset into train-set and test-set X_train, X_test, y_train, y_test = model_selection.train_test_split(X_features, y, test_size=0.2, random_state=42, shuffle=True) # write code to train and test the SVM classifier as the facemask presence detector clf = svm.SVC() clf.fit(X_train, y_train) predicted = clf.predict(X_test) print(predicted) print(y_test) test_score = clf.score(X_test, y_test) print(test_score),请逐行解释以上代码,并指出其问题
时间: 2024-04-16 21:25:25 浏览: 74
```python
y = np.concatenate([np.ones(len(X_processed)*len(X_processed[0])), np.zeros(len(X_masked)*len(X_masked[0]))])
print(y.shape)
```
这段代码将 `X_processed` 和 `X_masked` 中的样本数量计算后,创建了一个标签数组 `y`,其中 `X_processed` 对应的标签为1,`X_masked` 对应的标签为0。
```python
X_features = []
for x_list in X_processed:
for x in x_list:
x_feature = ft.hog(x, orientations=8, pixels_per_cell=(10, 10),
cells_per_block=(1, 1), visualize=False)
X_features.append(x_feature)
for x_list in X_masked:
for x in x_list:
x_feature = ft.hog(x, orientations=8, pixels_per_cell=(10, 10),
cells_per_block=(1, 1), visualize=False)
X_features.append(x_feature)
```
这段代码使用 `skimage.feature.hog` 函数从每个图像中提取 HOG 特征,并将这些特征存储在列表 `X_features` 中。
```python
X_train, X_test, y_train, y_test = model_selection.train_test_split(X_features, y, test_size=0.2, random_state=42,
shuffle=True)
```
这段代码使用 `sklearn.model_selection.train_test_split` 函数将数据集划分为训练集和测试集,其中训练集占80%,测试集占20%。
```python
clf = svm.SVC()
clf.fit(X_train, y_train)
```
这段代码创建了一个 `SVC`(支持向量机分类器)对象 `clf`,并使用训练集 `X_train` 和标签 `y_train` 对其进行训练。
```python
predicted = clf.predict(X_test)
print(predicted)
print(y_test)
```
这段代码使用训练好的分类器 `clf` 对测试集 `X_test` 进行预测,并打印预测结果和真实标签 `y_test`。
```python
test_score = clf.score(X_test, y_test)
print(test_score)
```
这段代码计算分类器在测试集上的准确率,并打印出来。
问题:
- 代码中缺少了模块的导入语句,例如 `import numpy as np`、`import skimage.feature as ft`、`from sklearn import model_selection, svm`。
- 代码中使用了 `ft.hog()` 函数来提取 HOG 特征,但没有导入 `skimage.feature.hog` 模块。
- 在划分数据集时,应该使用相同的随机种子(random_state)来保证每次划分的结果一致。
- 如果 `X_features` 和 `y` 的维度不匹配,可能会导致训练和预测出错。需要确保提取的特征和标签数量一致。
- 缺少评估分类器性能的相关指标,例如混淆矩阵、精确率和召回率等。可以使用 `sklearn.metrics` 模块来计算这些指标。
相关推荐
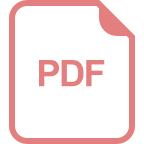
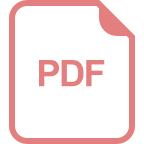
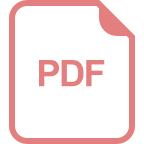
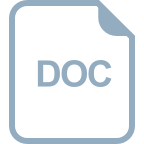
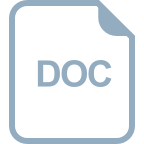
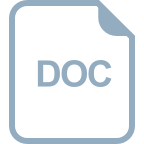
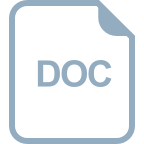
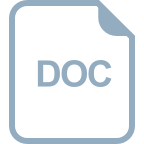










