cv.createTrackbar('radius', 'image', 50, min(int((n - 1) / 2), int((m - 1) / 2)), circleChange)什么意思
时间: 2024-06-03 10:12:56 浏览: 11
这段代码是在OpenCV中创建一个滑动条,用于调整圆的半径大小。具体解释如下:
- `cv.createTrackbar()` 是创建一个滑动条的函数。
- `'radius'` 是滑动条的名称。
- `'image'` 是滑动条所在的窗口名称。
- `50` 是滑动条的初始值。
- `min(int((n - 1) / 2), int((m - 1) / 2))` 是滑动条的最大值,其中 `n` 和 `m` 是图像的宽度和高度, `(n-1)/2` 和 `(m-1)/2` 分别是图像中心点的横纵坐标,因此 `min(int((n - 1) / 2), int((m - 1) / 2))` 取的是图像中心点到边缘的最短距离,作为滑动条的最大值,确保圆的半径不会超出图像边界。
- `circleChange` 是回调函数的名称,用于在滑动条值发生改变时执行相应的操作。
总的来说,这段代码的作用是在图像上创建一个滑动条,让用户可以通过调整滑动条的值来改变圆的半径大小。
相关问题
def En(image): x, y,_ = image.shape # 获取图片大小 radius = np.random.randint(10, int(min(x, y)), 1) # pos_x = np.random.randint(0, (min(x, y) - radius), 1) # 获取人脸光照区域的中心点坐标 pos_y = np.random.randint(0, (min(x, y) - radius), 1) # 获取人脸光照区域的中心坐标 pos_x = int(pos_x[0]) pos_y = int(pos_y[0]) radius = int(radius[0]) strength = 100 for j in range(pos_y - radius, pos_y + radius): for i in range(pos_x-radius, pos_x+radius): distance = math.pow((pos_x - i), 2) + math.pow((pos_y - j), 2) distance = np.sqrt(distance) if distance < radius: result = 1 - distance / radius result = result*strength # print(result) image[i, j, 0] = min((image[i, j, 0] + result),255) image[i, j, 1] = min((image[i, j, 1] + result),255) image[i, j, 2] = min((image[i, j, 2] + result),255) image = image.astype(np.uint8) return image对此函数进行文件夹内部图像处理
您可以使用以下代码对文件夹内的所有图像进行处理:
```python
import os
import cv2
import numpy as np
import math
def En(image):
x, y,_ = image.shape
radius = np.random.randint(10, int(min(x, y)), 1)
pos_x = np.random.randint(0, (min(x, y) - radius), 1)
pos_y = np.random.randint(0, (min(x, y) - radius), 1)
pos_x = int(pos_x[0])
pos_y = int(pos_y[0])
radius = int(radius[0])
strength = 100
for j in range(pos_y - radius, pos_y + radius):
for i in range(pos_x-radius, pos_x+radius):
distance = math.pow((pos_x - i), 2) + math.pow((pos_y - j), 2)
distance = np.sqrt(distance)
if distance < radius:
result = 1 - distance / radius
result = result*strength
image[i, j, 0] = min((image[i, j, 0] + result),255)
image[i, j, 1] = min((image[i, j, 1] + result),255)
image[i, j, 2] = min((image[i, j, 2] + result),255)
image = image.astype(np.uint8)
return image
img_folder_path = "YOUR_FOLDER_PATH" # 修改为你的文件夹路径
for filename in os.listdir(img_folder_path):
if filename.endswith(".jpg") or filename.endswith(".png"): # 处理jpg和png格式的图像
img_path = os.path.join(img_folder_path, filename)
img = cv2.imread(img_path)
img_en = En(img)
cv2.imwrite(img_path, img_en)
```
该代码会遍历指定文件夹内的所有图像,对每一张图像都进行处理并保存。您只需要将 `YOUR_FOLDER_PATH` 修改为您的文件夹路径即可。
def TEST(): global col global squ ret, frame = image.read() color_lower = np.array([int(Hmin.value),int(Smin.value),int(Vmin.value)]) color_upper = np.array([int(Hmax.value), int(Smax.value), int(Vmax.value)]) color1_lower = np.array([int(H1min.value),int(S1min.value),int(V1min.value)]) color1_upper = np.array([int(H1max.value), int(S1max.value), int(V1max.value)]) #frame = cv2.resize(frame, (400, 400)) frame_ = cv2.GaussianBlur(frame,(5,5),0) hsv = cv2.cvtColor(frame,cv2.COLOR_BGR2HSV) mask = cv2.inRange(hsv,color_lower,color_upper) mask = cv2.erode(mask,None,iterations=2) mask = cv2.dilate(mask,None,iterations=2) mask = cv2.GaussianBlur(mask,(3,3),0) mask1 = cv2.inRange(hsv,color1_lower,color1_upper) mask1 = cv2.erode(mask1,None,iterations=2) mask1 = cv2.dilate(mask1,None,iterations=2) mask1 = cv2.GaussianBlur(mask1,(3,3),0) cnts = cv2.findContours(mask.copy(),cv2.RETR_EXTERNAL,cv2.CHAIN_APPROX_SIMPLE)[-2] cnts1 = cv2.findContours(mask1.copy(),cv2.RETR_EXTERNAL,cv2.CHAIN_APPROX_SIMPLE)[-2] if len(cnts) > 0: # 找到最大的轮廓 cnt = max(cnts, key=cv2.contourArea) (color_x,color_y),color_radius = cv2.minEnclosingCircle(cnt) if color_radius > 10: squ=1 else: squ=0 if len(cnts1) > 0: # 找到最大的轮廓 cnt1 = max(cnts1, key=cv2.contourArea) (color1_x,color1_y),color1_radius = cv2.minEnclosingCircle(cnt1) if color1_radius > 10: col=1 else: col=0
这段代码是一个函数`TEST()`,它的作用是读取图像并进行一系列的处理。首先,它从图像中读取帧。然后,根据一些阈值(`Hmin.value`,`Smin.value`,`Vmin.value`等)定义颜色范围。接下来,它对帧进行高斯模糊处理,并将帧从BGR颜色空间转换为HSV颜色空间。然后,它使用颜色范围创建掩码,并对掩码进行腐蚀和膨胀处理,以去除噪声并平滑掩码。接着,它使用`cv2.findContours()`函数找到掩码中的轮廓,并选择最大的轮廓。最后,它计算最小包围圆的圆心和半径,并根据半径大小将`col`和`squ`变量设置为1或0。
请问你还有其他问题吗?
相关推荐
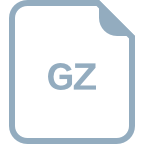
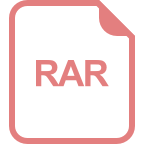
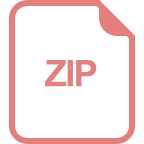












