用python写一个有监督学习中的判定树算法
时间: 2024-03-07 21:47:48 浏览: 26
好的,以下是一个使用Python实现的ID3决策树算法(基于信息增益)。假设我们有一个二元分类问题,数据集如下所示:
| 属性1 | 属性2 | 类别 |
|-------|-------|------|
| 1 | 1 | 1 |
| 1 | 0 | 1 |
| 0 | 1 | 0 |
| 0 | 0 | 0 |
首先,我们需要写一个函数来计算信息熵:
```python
import math
def calc_entropy(data):
n = len(data)
if n == 0:
return 0
count = {}
for d in data:
label = d[-1]
if label not in count:
count[label] = 0
count[label] += 1
entropy = 0
for c in count.values():
p = c / n
entropy -= p * math.log2(p)
return entropy
```
然后,我们需要写一个函数来计算信息增益:
```python
def calc_gain(data, feature_idx):
n = len(data)
if n == 0:
return 0
entropy_before = calc_entropy(data)
count = {}
for d in data:
feature_value = d[feature_idx]
label = d[-1]
if feature_value not in count:
count[feature_value] = {}
if label not in count[feature_value]:
count[feature_value][label] = 0
count[feature_value][label] += 1
entropy_after = 0
for feature_value, label_count in count.items():
p = sum(label_count.values()) / n
entropy = calc_entropy(label_count.values())
entropy_after += p * entropy
return entropy_before - entropy_after
```
接下来,我们可以编写一个递归函数来构建决策树:
```python
def build_tree(data, feature_list):
# 如果数据集为空,则返回空节点
if len(data) == 0:
return None
# 如果数据集的所有样本都属于同一类别,则返回叶子节点
labels = set(d[-1] for d in data)
if len(labels) == 1:
return labels.pop()
# 如果特征列表为空,则返回叶子节点,该节点的类别为数据集中样本数最多的类别
if len(feature_list) == 0:
label_counts = {}
for d in data:
label = d[-1]
if label not in label_counts:
label_counts[label] = 0
label_counts[label] += 1
return max(label_counts, key=label_counts.get)
# 选择信息增益最大的特征
best_feature = max(feature_list, key=lambda x: calc_gain(data, x))
# 构建根节点
root = {'feature': best_feature, 'children': {}}
# 将数据集划分成若干个子集,并递归构建子树
for feature_value in set(d[best_feature] for d in data):
sub_data = [d for d in data if d[best_feature] == feature_value]
sub_tree = build_tree(sub_data, [f for f in feature_list if f != best_feature])
root['children'][feature_value] = sub_tree
return root
```
最后,我们可以使用构建好的决策树来进行预测:
```python
def predict(tree, sample):
# 如果当前节点是叶子节点,则返回该节点的类别
if type(tree) != dict:
return tree
# 否则,根据样本的特征值找到下一个节点,并递归预测
feature_value = sample[tree['feature']]
if feature_value not in tree['children']:
return None
sub_tree = tree['children'][feature_value]
return predict(sub_tree, sample)
```
现在,我们可以使用上述的函数来构建决策树并进行预测:
```python
data = [
[1, 1, 1],
[1, 0, 1],
[0, 1, 0],
[0, 0, 0]
]
feature_list = [0, 1] # 特征列表
tree = build_tree(data, feature_list) # 构建决策树
sample = [1, 0] # 待预测样本
print(predict(tree, sample)) # 输出预测结果
```
输出结果为1,即属于第一类。
相关推荐
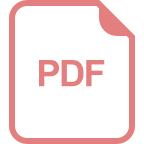
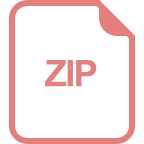
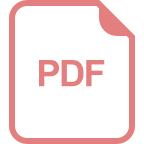















