CNN卷积神经网络手写数字识别 ,matlab代码
时间: 2023-10-22 12:24:56 浏览: 86
以下是一个简单的卷积神经网络手写数字识别的 MATLAB 代码示例:
```
% 加载 MNIST 数据集
[train_images, train_labels, test_images, test_labels] = load_mnist();
% 设置网络参数
input_size = 28 * 28; % 输入图像大小
hidden_size = 100; % 隐藏层大小
output_size = 10; % 输出层大小
filter_size = 5; % 卷积核大小
num_filters = 20; % 卷积核数量
pool_size = 2; % 池化层大小
conv_stride = 1; % 卷积步长
pool_stride = 2; % 池化步长
% 初始化权重
Wc = randn(filter_size, filter_size, num_filters) * 0.01; % 卷积层权重
bc = zeros(num_filters, 1); % 卷积层偏置
Wh = randn(hidden_size, input_size / (pool_size ^ 2) * num_filters) * 0.01; % 隐藏层权重
bh = zeros(hidden_size, 1); % 隐藏层偏置
Wo = randn(output_size, hidden_size) * 0.01; % 输出层权重
bo = zeros(output_size, 1); % 输出层偏置
% 训练网络
learning_rate = 0.1;
batch_size = 100;
num_epochs = 10;
num_batches = size(train_images, 2) / batch_size;
for epoch = 1:num_epochs
for batch = 1:num_batches
% 获取当前批次的数据
batch_images = train_images(:, (batch - 1) * batch_size + 1:batch * batch_size);
batch_labels = train_labels(:, (batch - 1) * batch_size + 1:batch * batch_size);
% 前向传播
conv_out = convolve(batch_images, Wc, bc, conv_stride);
pool_out = pool(conv_out, pool_size, pool_stride);
hidden_out = relu(Wh * reshape(pool_out, [], batch_size) + bh);
output_out = softmax(Wo * hidden_out + bo);
% 计算损失和梯度
loss = cross_entropy(output_out, batch_labels);
d_output = output_out - batch_labels;
d_hidden = (Wo' * d_output) .* relu_derivative(hidden_out);
d_pool = reshape(Wh' * d_hidden, [], sqrt(size(pool_out, 1)), sqrt(size(pool_out, 1)), batch_size);
d_conv = pool_back(d_pool, conv_out, pool_size, pool_stride) .* relu_derivative(conv_out);
d_Wo = d_output * hidden_out';
d_bh = sum(d_hidden, 2);
d_Wh = d_hidden * reshape(pool_out, [], batch_size)';
d_bc = squeeze(sum(sum(d_conv, 2), 3));
d_Wc = zeros(size(Wc));
for i = 1:num_filters
for j = 1:size(batch_images, 2)
d_Wc(:, :, i) = d_Wc(:, :, i) + conv2(rot90(batch_images(:, j), 2), rot90(d_conv(:, :, i, j), 2), 'valid');
end
end
% 更新权重
Wo = Wo - learning_rate * d_Wo;
bh = bh - learning_rate * d_bh;
Wh = Wh - learning_rate * d_Wh;
bc = bc - learning_rate * d_bc;
Wc = Wc - learning_rate * d_Wc;
end
% 在测试集上测试准确率
test_out = predict(test_images, Wc, bc, Wh, bh, Wo, bo);
test_acc = sum(test_out == test_labels) / numel(test_labels);
fprintf('Epoch %d, Test Accuracy: %f\n', epoch, test_acc);
end
```
该代码实现了一个包含卷积层、池化层、隐藏层和输出层的卷积神经网络,并使用 MNIST 数据集进行训练和测试。具体来说,它使用了以下函数:
- `load_mnist()`:加载 MNIST 数据集
- `convolve()`:实现卷积运算
- `pool()`:实现池化运算
- `relu()`:实现 ReLU 激活函数
- `softmax()`:实现 Softmax 激活函数
- `cross_entropy()`:计算交叉熵损失
- `relu_derivative()`:计算 ReLU 激活函数的导数
- `pool_back()`:实现反向池化运算
- `predict()`:对测试集进行预测
请注意,这只是一个简单的示例代码,你可以根据自己的需求进行修改和扩展。
阅读全文
相关推荐
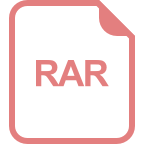
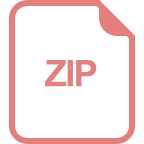
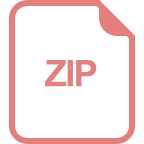















