t=time() pycolmap.match_exhaustive(database_path) t=time() - t timings['RANSAC'].append(t) print(f'RANSAC in {t:.4f} sec')这段代码在做什么
时间: 2024-01-15 18:02:30 浏览: 24
这段代码仅仅是在计算使用`pycolmap.match_exhaustive()`函数进行图像特征匹配的时间,并将这个时间记录到`timings`字典中的`'RANSAC'`键对应的值中。具体来说,这段代码的作用是:
1. 获取当前时间戳。
2. 使用`pycolmap.match_exhaustive()`函数进行图像特征匹配。
3. 计算匹配所花费的时间,并将这个时间记录到`timings`字典中的`'RANSAC'`键对应的值中。
4. 打印输出匹配所花费的时间。
需要注意的是,这段代码中并没有使用RANSAC算法,它使用的仅仅是暴力匹配算法。因此,`'RANSAC'`这个键名并不太准确,应该改为`'Brute-Force Matching'`或者`'Exhaustive Matching'`比较合适。
相关问题
pycolmap.match_exhaustive()
`pycolmap.match_exhaustive()`是一个函数,它可以在COLMAP中进行图像特征匹配。这个函数使用暴力匹配算法来计算两幅图像之间的所有可能的匹配,因此它可能会非常耗时。在使用这个函数之前,你需要先使用`pycolmap.extract_features()`函数来提取图像特征。这个函数的语法如下:
```
matches = pycolmap.match_exhaustive(db_path, query_image_id, num_matches, config_path)
```
其中,`db_path`是COLMAP数据库的路径,`query_image_id`是要匹配的图像的ID,`num_matches`是要返回的匹配数目,`config_path`是COLMAP配置文件的路径。这个函数返回一个Numpy数组,其中每一行表示一组匹配,包括两个特征的索引和它们之间的距离。
t=time() # 使用COLMAP执行RANSAC算法进行三维重建 pycolmap.match_exhaustive(database_path) t=time() - t timings['RANSAC'].append(t) print(f'RANSAC in {t:.4f} sec')这段代码用了ransac算法吗
这段代码中并没有使用RANSAC算法,它只是使用了`pycolmap.match_exhaustive()`函数来进行暴力匹配。如果要使用RANSAC算法进行三维重建,则需要使用`pycolmap.triangulate()`函数和`pycolmap.bundle_adjustment()`函数来进行三角化和优化,其中`pycolmap.triangulate()`函数会使用RANSAC算法进行匹配筛选和三角化,而`pycolmap.bundle_adjustment()`函数则会使用非线性优化算法来优化三维点和相机位姿。具体的操作流程可以参考COLMAP官方文档中的教程。
相关推荐
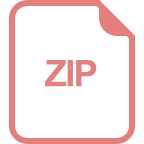
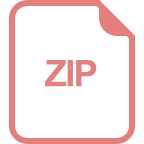
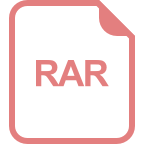












