scheduler = torch.optim.lr_scheduler.ReduceLROnPlateau(optimizer, mode='min', factor=0.1, patience=200)这行代码啥意思
时间: 2024-06-06 07:05:09 浏览: 218
这行代码定义了一个学习率调度程序,将根据模型在验证集上的表现来动态地调整学习率。
具体地说,`torch.optim.lr_scheduler.ReduceLROnPlateau` 是 PyTorch 提供的一种学习率调度程序,它会监测一个指标(在这里是验证集上的损失值),如果这个指标在连续 `patience` 个 epoch 上都没有改善,就将当前学习率乘以 `factor`。这个过程会一直持续下去,直到学习率下降到 `min_lr` 或者达到了最大的调整次数 `max_iter`。
在这里,`mode='min'` 表示我们希望监测的指标越小越好(即验证集上的损失值越小越好),`factor=0.1` 表示每次调整时将学习率乘以 0.1,`patience=200` 表示如果连续 200 个 epoch 都没有改善,就进行一次调整。`optimizer` 是我们定义的优化器,这个调度程序将根据优化器的状态来调整学习率。
相关问题
scheduler = torch.optim.lr_scheduler.ReduceLROnPlateau
The `scheduler` variable is an instance of the `ReduceLROnPlateau` class from the PyTorch `optim.lr_scheduler` module. This class implements a learning rate scheduler that monitors a specified metric and reduces the learning rate if the metric does not improve for a certain number of epochs.
The `ReduceLROnPlateau` scheduler takes the following parameters:
- `optimizer`: The optimizer that is being used to train the model.
- `mode`: Specifies whether the metric being monitored should be minimized or maximized. Possible values are `'min'`, `'max'`, or `'auto'` (which infers the mode based on the metric name).
- `factor`: The factor by which the learning rate is reduced. For example, if `factor=0.1`, the learning rate will be reduced by a factor of 0.1 (i.e., the new learning rate will be 0.1 times the old learning rate).
- `patience`: The number of epochs to wait before reducing the learning rate if the metric does not improve.
- `verbose`: Specifies whether to print information about the learning rate changes.
- `threshold`: The threshold for measuring the new optimum, to only focus on significant changes.
- `threshold_mode`: Specifies whether the threshold is relative (`'rel'`) or absolute (`'abs'`).
The `scheduler.step()` method is called at the end of each epoch to update the learning rate based on the monitored metric.
详细分析一下python代码:import torch.optim as optim criterion = nn.CrossEntropyLoss() optimizer = optim.Adam(net.parameters(), lr=0.01, betas=(0.9, 0.999), eps=1e-08, weight_decay=0, amsgrad=False) scheduler = optim.lr_scheduler.ReduceLROnPlateau(optimizer, mode='min', factor=0.1, patience=10, verbose=True, min_lr=0) loss_hist, acc_hist = [], [] loss_hist_val, acc_hist_val = [], [] for epoch in range(140): running_loss = 0.0 correct = 0 for data in train_loader: batch, labels = data batch, labels = batch.to(device), labels.to(device) optimizer.zero_grad() outputs = net(batch) loss = criterion(outputs, labels) loss.backward() optimizer.step() # compute training statistics _, predicted = torch.max(outputs, 1) correct += (predicted == labels).sum().item() running_loss += loss.item() avg_loss = running_loss / len(train_set) avg_acc = correct / len(train_set) loss_hist.append(avg_loss) acc_hist.append(avg_acc) # validation statistics net.eval() with torch.no_grad(): loss_val = 0.0 correct_val = 0 for data in val_loader: batch, labels = data batch, labels = batch.to(device), labels.to(device) outputs = net(batch) loss = criterion(outputs, labels) _, predicted = torch.max(outputs, 1) correct_val += (predicted == labels).sum().item() loss_val += loss.item() avg_loss_val = loss_val / len(val_set) avg_acc_val = correct_val / len(val_set) loss_hist_val.append(avg_loss_val) acc_hist_val.append(avg_acc_val) net.train() scheduler.step(avg_loss_val) print('[epoch %d] loss: %.5f accuracy: %.4f val loss: %.5f val accuracy: %.4f' % (epoch + 1, avg_loss, avg_acc, avg_loss_val, avg_acc_val))
这段代码是一个基于PyTorch的神经网络训练过程。代码中使用了torch.optim模块中Adam优化器和ReduceLROnPlateau学习率调度器。其中,Adam优化器用于优化网络的参数,而ReduceLROnPlateau调度器用于自动调整学习率以提高训练效果。代码中使用nn.CrossEntropyLoss()作为损失函数,用于计算输出结果与标签之间的差距。
接下来的代码中使用了两个循环,一个是对训练数据集的循环,另一个是对验证数据集的循环。在训练数据集循环中,首先将数据集分成一个个batch,然后将batch和对应的标签传入网络进行前向传播,计算损失值,然后进行反向传播和参数更新。在这个过程中,记录了每个batch的正确预测个数和损失值,最后计算平均损失和准确率,并将其保存在loss_hist和acc_hist列表中。
在验证数据集循环中,同样将数据集分成一个个batch,然后将batch和对应的标签传入网络进行前向传播,计算损失值,并计算正确预测个数。最后将每个batch的平均损失和准确率记录在loss_hist_val和acc_hist_val列表中。
在每个epoch结束后,调用scheduler.step(avg_loss_val)方法来更新学习率,并打印出当前epoch的训练和验证结果。其中,avg_loss和avg_acc记录了该epoch的训练结果,avg_loss_val和avg_acc_val记录了该epoch的验证结果。
阅读全文
相关推荐
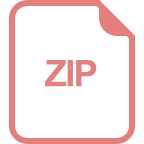
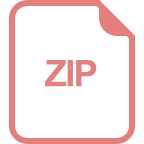



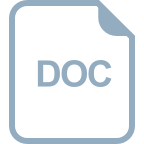









