confusion matrix python
时间: 2023-04-28 22:01:46 浏览: 81
混淆矩阵是一种用于评估分类模型性能的工具,通常用于机器学习和统计学中。在Python中,可以使用scikit-learn库中的confusion_matrix函数来生成混淆矩阵。混淆矩阵是一个二维数组,其中行表示实际类别,列表示预测类别。对角线上的元素表示正确分类的样本数,而非对角线上的元素表示错误分类的样本数。通过分析混淆矩阵,可以计算出各种分类指标,如准确率、召回率、F1分数等。
相关问题
plot_confusion_matrix python代码
以下是一个简单的 Python 代码,用于绘制混淆矩阵:
```python
import numpy as np
import matplotlib.pyplot as plt
from sklearn.metrics import confusion_matrix
def plot_confusion_matrix(y_true, y_pred, classes,
normalize=False,
title=None,
cmap=plt.cm.Blues):
"""
This function prints and plots the confusion matrix.
Normalization can be applied by setting `normalize=True`.
"""
cm = confusion_matrix(y_true, y_pred)
if normalize:
cm = cm.astype('float') / cm.sum(axis=1)[:, np.newaxis]
print("Normalized confusion matrix")
else:
print('Confusion matrix, without normalization')
print(cm)
plt.imshow(cm, interpolation='nearest', cmap=cmap)
plt.title(title)
plt.colorbar()
tick_marks = np.arange(len(classes))
plt.xticks(tick_marks, classes, rotation=45)
plt.yticks(tick_marks, classes)
fmt = '.2f' if normalize else 'd'
thresh = cm.max() / 2.
for i, j in np.ndindex(cm.shape):
plt.text(j, i, format(cm[i, j], fmt),
horizontalalignment="center",
color="white" if cm[i, j] > thresh else "black")
plt.tight_layout()
plt.ylabel('True label')
plt.xlabel('Predicted label')
plt.show()
```
这个函数使用了 scikit-learn 库中的 confusion_matrix 函数,用于计算混淆矩阵。它还使用了 matplotlib 库来绘制混淆矩阵的图形。您可以将真实标签和预测标签作为参数传递给该函数,以及一个类列表,用于标识每个类别。您还可以选择是否对混淆矩阵进行归一化,并为图形添加标题。
python绘制confusion matrix的代码
以下是一个使用Python和matplotlib库绘制混淆矩阵的示例代码:
```python
import numpy as np
import matplotlib.pyplot as plt
def plot_confusion_matrix(cm, classes, normalize=False, title='Confusion matrix', cmap=plt.cm.Blues):
"""
This function prints and plots the confusion matrix.
Normalization can be applied by setting `normalize=True`.
"""
if normalize:
cm = cm.astype('float') / cm.sum(axis=1)[:, np.newaxis]
print("Normalized confusion matrix")
else:
print('Confusion matrix, without normalization')
print(cm)
plt.imshow(cm, interpolation='nearest', cmap=cmap)
plt.title(title)
plt.colorbar()
tick_marks = np.arange(len(classes))
plt.xticks(tick_marks, classes, rotation=45)
plt.yticks(tick_marks, classes)
fmt = '.2f' if normalize else 'd'
thresh = cm.max() / 2.
for i, j in itertools.product(range(cm.shape[0]), range(cm.shape[1])):
plt.text(j, i, format(cm[i, j], fmt),
horizontalalignment="center",
color="white" if cm[i, j] > thresh else "black")
plt.tight_layout()
plt.ylabel('True label')
plt.xlabel('Predicted label')
# 示例数据
classes = ['cat', 'dog', 'fish']
cm = np.array([[10, 2, 1],
[3, 15, 2],
[0, 1, 8]])
# 绘制混淆矩阵
plt.figure()
plot_confusion_matrix(cm, classes)
plt.show()
```
上述代码将输出一个混淆矩阵图像,如下所示:

相关推荐
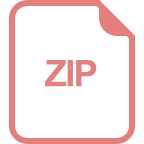
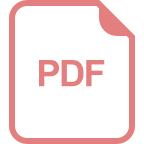
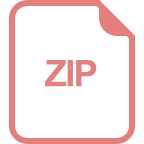












