autograd.Function实现relu
时间: 2024-05-12 08:15:42 浏览: 85
下面是使用`autograd.Function`实现ReLU函数的示例代码:
```python
import torch
class MyReLUFunction(torch.autograd.Function):
@staticmethod
def forward(ctx, input):
ctx.save_for_backward(input)
output = torch.clamp(input, min=0)
return output
@staticmethod
def backward(ctx, grad_output):
input, = ctx.saved_tensors
grad_input = grad_output.clone()
grad_input[input < 0] = 0
return grad_input
# Create a tensor and apply the custom ReLU function
x = torch.tensor([-2.0, -1.0, 0.0, 1.0, 2.0], requires_grad=True)
y = MyReLUFunction.apply(x)
# Compute gradients using autograd
y.sum().backward()
# Print the gradients
print(x.grad)
```
在这个示例中,我们定义了一个名为`MyReLUFunction`的新的`autograd.Function`。我们重载了`forward`和`backward`方法来实现ReLU函数的前向传播和反向传播。在前向传播中,我们使用`clamp`函数来实现ReLU操作。在反向传播中,我们需要计算输入`grad_input`相对于输出`grad_output`的梯度。我们先将`grad_output`克隆一份,然后将小于0的输入值的梯度设置为0。最后,我们返回`grad_input`。
在示例中,我们创建一个输入张量`x`,然后应用自定义ReLU函数`MyReLUFunction`。接着,我们使用`autograd`计算梯度,并打印出来。
阅读全文
相关推荐
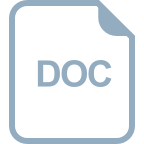
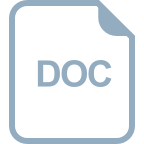
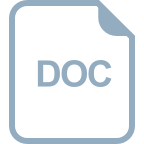




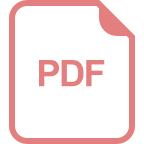
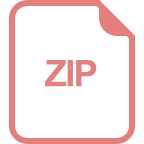
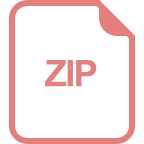
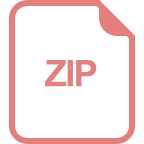
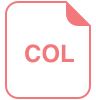
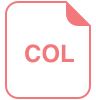
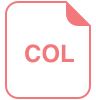
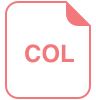



