while ~isempty(indexs(flags)) temp_index = indexs(flags); source = y(:,temp_index(1)); flags(temp_index(1)) = 0; temp_index = temp_index(2:end); temp_flag = []; for i = 1: length(temp_index) corrs = corrcoef(source,y(:,temp_index(i)));%矩阵相关系数 corrs = corrs(1,2); if corrs >= threshold_corr temp_flag(end+1) = i; end end flags(temp_index(temp_flag)) = 0; sgc(:,end+1) = source+sum(y(:,temp_index(temp_flag)),2); g_h = sum(sgc,2); g_h_e = sum((x-g_h).^2);什么意思
时间: 2023-06-15 19:07:57 浏览: 92
这段代码是一个基于相关性的信号子图聚类算法,用于从输入信号y中提取相关性较强的子信号群,使得每个子信号群可以代表原始信号的一部分。具体来说,该算法的输入为一个矩阵y,其中每列代表一个信号,输出为一个矩阵sgc,其中每列代表一个子信号群。该算法的主要步骤如下:
1. 初始化标志向量flags,表示每个信号是否已经被加入到子信号群中。
2. 循环直到所有信号都被加入到子信号群中:
a. 找到未被加入到子信号群中的信号中的第一个信号source。
b. 计算source与其他未被加入到子信号群中的信号的相关系数corrs,并将与source相关系数大于等于阈值threshold_corr的其他信号的索引添加到temp_flag中。
c. 将temp_flag中的所有信号的标志位设置为0。
d. 将source和temp_flag中的所有信号加权相加得到一个新的子信号群,并将其添加到sgc中。
e. 计算sgc中所有子信号群的加权和g_h,并计算输入信号x与g_h之间的欧氏距离g_h_e。
相关问题
while ~isempty(indexs(flags)) temp_index = indexs(flags); source = y(:,temp_index(1)); flags(temp_index(1)) = 0; temp_index = temp_index(2:end); temp_flag = []; for i = 1: length(temp_index) corrs = corrcoef(source,y(:,temp_index(i))) corrs = corrs(1,2); if corrs >= threshold_corr temp_flag(end+1) = i; 这怎么改成修正余弦相似度
要将这段代码改成修正余弦相似度,需要进行以下修改:
1. 将 `corrcoef` 函数修改为计算修正余弦相似度的函数。修正余弦相似度可以通过以下公式计算:
$$
r_{xy} = \frac{\sum\limits_{i=1}^n (x_i - \bar{x}) (y_i - \bar{y})}{\sqrt{\sum\limits_{i=1}^n (x_i - \bar{x})^2} \sqrt{\sum\limits_{i=1}^n (y_i - \bar{y})^2} + \epsilon}
$$
其中,$x$ 和 $y$ 是要比较的两个向量,$n$ 是向量的长度,$\bar{x}$ 和 $\bar{y}$ 是两个向量的均值,$\epsilon$ 是一个很小的数,防止分母为零。
2. 将 `corrs` 的计算方法改为修正余弦相似度的计算方法,即:
```
corrs = sum((source - mean(source)).*(y(:,temp_index(i)) - mean(y(:,temp_index(i)))))/(sqrt(sum((source - mean(source)).^2))*sqrt(sum((y(:,temp_index(i)) - mean(y(:,temp_index(i)))).^2)) + eps;
```
其中,`source` 是要比较的向量,`y(:,temp_index(i))` 是另一个向量,`eps` 是一个很小的数,防止分母为零。
修改后的代码如下:
```
while ~isempty(indexs(flags))
temp_index = indexs(flags);
source = y(:,temp_index(1));
flags(temp_index(1)) = 0;
temp_index = temp_index(2:end);
temp_flag = [];
for i = 1: length(temp_index)
corrs = sum((source - mean(source)).*(y(:,temp_index(i)) - mean(y(:,temp_index(i)))))/(sqrt(sum((source - mean(source)).^2))*sqrt(sum((y(:,temp_index(i)) - mean(y(:,temp_index(i)))).^2)) + eps;
if corrs >= threshold_corr
temp_flag(end+1) = i;
end
end
flags(temp_index(temp_flag)) = 0;
end
```
indexs = linspace(1,d,d);%生成等差数列。语法为:linspace(x1, x2, n),其中x1是起始值,x2是终止值,n是生成的数字个数 flags = logical(indexs);%逻辑函数 x_e = sum((x-mean(x)).^2);%mean 矩阵均值 方差 sgc = []; g_h = 0; g_h_e = 0; while ~isempty(indexs(flags)) temp_index = indexs(flags); source = y(:,temp_index(1)); flags(temp_index(1)) = 0; temp_index = temp_index(2:end); temp_flag = []; for i = 1: length(temp_index) corrs = corrcoef(source,y(:,temp_index(i)));%矩阵相关系数 corrs = corrs(1,2); if corrs >= threshold_corr temp_flag(end+1) = i; end end flags(temp_index(temp_flag)) = 0; sgc(:,end+1) = source+sum(y(:,temp_index(temp_flag)),2); g_h = sum(sgc,2); g_h_e = sum((x-g_h).^2); if g_h_e / x_e < threshold_nmse break end将这块的相似条件改为余弦相似度
可以将代码中的corrcoef函数替换为pdist2函数,使用余弦相似度计算矩阵之间的相似度。具体代码如下:
indexs = linspace(1,d,d);
flags = logical(indexs);
x_e = sum((x-mean(x)).^2);
sgc = [];
g_h = 0;
g_h_e = 0;
while ~isempty(indexs(flags))
temp_index = indexs(flags);
source = y(:,temp_index(1));
flags(temp_index(1)) = 0;
temp_index = temp_index(2:end);
temp_flag = [];
for i = 1: length(temp_index)
corrs = pdist2(source', y(:,temp_index(i))', 'cosine');
if corrs <= threshold_cosine
temp_flag(end+1) = i;
end
end
flags(temp_index(temp_flag)) = 0;
sgc(:,end+1) = source+sum(y(:,temp_index(temp_flag)),2);
g_h = sum(sgc,2);
g_h_e = sum((x-g_h).^2);
if g_h_e / x_e < threshold_nmse
break
end
end
这样代码中的相似条件就被改为了余弦相似度。
阅读全文
相关推荐
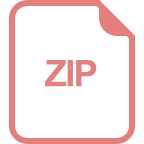
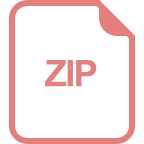
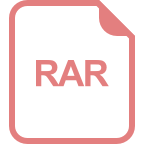










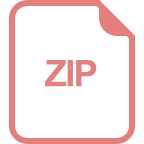
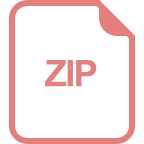
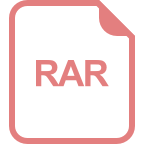
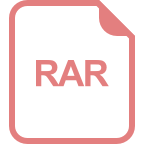