paddle使用StackingEnsembleForecaster报错AttributeError: 'list' object has no attribute 'target'
时间: 2023-07-15 16:56:06 浏览: 68
这个错误通常是因为在使用StackingEnsembleForecaster时,传入的数据格式不正确导致的。StackingEnsembleForecaster要求传入的训练数据必须是一个Pandas DataFrame或Dict,其中包含训练数据和对应的标签。请检查一下你传入的数据格式是否正确,是否包括了标签信息。如果问题还存在,可以提供更详细的错误信息和代码,以便更好地帮助你解决问题。
相关问题
paddle报错AttributeError: 'list' object has no attribute 'get_target'
这个错误通常是因为您在使用 PaddlePaddle 的时候,将一个列表对象传入了需要一个单独的张量的函数中。这个函数在执行的时候,会尝试调用列表对象上不存在的方法,导致了 `AttributeError: 'list' object has no attribute 'get_target'` 的异常。
要解决这个问题,您需要检查您的代码,找到传入了列表对象的函数,并将其替换为单独的张量。如果您无法确定哪个函数出现了问题,您可以通过打印出相关变量的类型和形状来进行调试。
reg.fit(dataset_train_scaled, dataset_val_scaled)其中dataset_train_scaled, dataset_val_scaled为列表类型,paddle模型使用StackingEnsembleForecaster进行多路时序数据联合训练时报错AttributeError: 'list' object has no attribute 'target',怎么将上述列表修改为为符合StackingEnsembleForecaster模型的dict类型的列表
假设原先的 `dataset_train_scaled` 和 `dataset_val_scaled` 是一个由多个时间序列数据组成的列表,每个时间序列数据都有两列数据,一列是时间序列值,另一列是该时间序列对应的目标值,那么可以按照以下方式将其转换为符合StackingEnsembleForecaster模型要求的dict类型的列表:
```python
import pandas as pd
import numpy as np
# 假设原始数据是一个列表,每个元素都是一个pandas.DataFrame对象
dataset_train = [pd.DataFrame({'time': np.arange(10), 'value': np.random.rand(10), 'target': np.random.rand(10)}) for _ in range(5)]
dataset_val = [pd.DataFrame({'time': np.arange(10), 'value': np.random.rand(10), 'target': np.random.rand(10)}) for _ in range(5)]
# 将每个DataFrame对象转换为dict类型的对象
dataset_train_dict = [{'ts': df[['time', 'value']], 'target': df['target'], 'start': df['time'].iloc[0], 'freq': 'D'} for df in dataset_train]
dataset_val_dict = [{'ts': df[['time', 'value']], 'target': df['target'], 'start': df['time'].iloc[0], 'freq': 'D'} for df in dataset_val]
```
上面的代码将每个DataFrame对象转换为一个dict对象,并进行了适当的字段设置,包括时间序列数据、目标数据、起始时间和采样频率。这样就可以将其作为StackingEnsembleForecaster模型的输入数据了:
```python
from paddle.incubate.forecast import StackingEnsembleForecaster
reg = StackingEnsembleForecaster(estimators=[model1, model2, model3], output_dim=1)
reg.fit(dataset_train_dict, dataset_val_dict)
```
这样就可以使用StackingEnsembleForecaster模型进行多路时序数据联合训练了。注意,以上代码仅供参考,具体实现方式需要根据你的数据格式进行适当的调整。
阅读全文
相关推荐
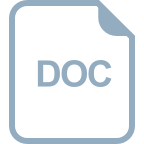
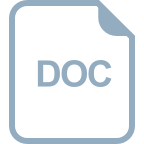
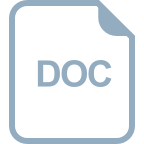





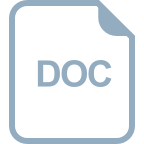
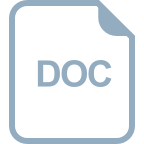
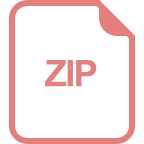
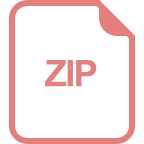
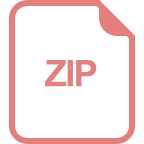
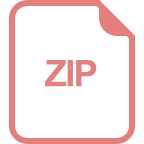
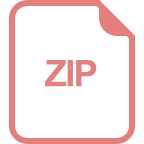