用pytorch写一段四元数卷积
时间: 2023-09-11 18:04:50 浏览: 85
### 回答1:
下面是一段用 PyTorch 实现四元数卷积的代码示例:
```
import torch
import torch.nn as nn
import torch.nn.functional as F
class QuaternionConv2d(nn.Module):
def __init__(self, in_channels, out_channels, kernel_size, stride=1, padding=0, bias=True):
super(QuaternionConv2d, self).__init__()
self.in_channels = in_channels
self.out_channels = out_channels
self.kernel_size = kernel_size
self.stride = stride
self.padding = padding
self.bias = bias
# 四元数卷积的权值是复数,因此需要 4 倍输入通道数
self.weight = nn.Parameter(torch.Tensor(out_channels, in_channels * 4, kernel_size, kernel_size))
if bias:
self.bias = nn.Parameter(torch.Tensor(out_channels))
else:
self.register_parameter('bias', None)
self.reset_parameters()
def reset_parameters(self):
nn.init.kaiming_uniform_(self.weight, a=math.sqrt(5))
if self.bias is not None:
fan_in, _ = nn.init._calculate_fan_in_and_fan_out(self.weight)
bound = 1 / math.sqrt(fan_in)
nn.init.uniform_(self.bias, -bound, bound)
def forward(self, input):
# 将四元数展开为 4 个通道
input = input.reshape(input.size(0), self.in_channels, 4, input.size(2), input.size(3))
real, imag = input[:, :, 0], input[:, :, 1:]
real, imag = real.permute(0, 2, 1, 3, 4), imag.permute(0, 2, 1, 3, 4)
# 实数部分与虚数部分分别卷积
real_out = F.conv2d(real, self.weight[:, :self.in_channels, :, :], stride=self.stride, padding=self.padding)
imag_out = F.conv2d(imag, self.weight[:, self.in_channels:, :, :], stride=self.stride, padding=self.padding)
# 将实数部分与虚数部分合并为四元数
out = torch.stack([real_out, imag_out[
### 回答2:
四元数卷积是一种基于四元数数学运算的卷积操作。在使用PyTorch实现四元数卷积时,可以按照以下步骤进行:
1. 导入必要的模块
首先,我们需要导入PyTorch库,并确保已经安装了相关依赖库。
```python
import torch
import torch.nn as nn
import torch.nn.functional as F
```
2. 定义四元数卷积层
使用PyTorch的模块定义一个四元数卷积层。四元数卷积层的结构与普通卷积层类似,只是在计算卷积过程中使用了四元数的数学运算。
```python
class QuaternionConv2d(nn.Module):
def __init__(self, in_channels, out_channels, kernel_size, stride=1, padding=0, bias=True):
super(QuaternionConv2d, self).__init__()
self.conv_r = nn.Conv2d(in_channels, out_channels, kernel_size, stride=stride, padding=padding, bias=bias)
self.conv_i = nn.Conv2d(in_channels, out_channels, kernel_size, stride=stride, padding=padding, bias=bias)
self.conv_j = nn.Conv2d(in_channels, out_channels, kernel_size, stride=stride, padding=padding, bias=bias)
self.conv_k = nn.Conv2d(in_channels, out_channels, kernel_size, stride=stride, padding=padding, bias=bias)
def forward(self, x):
qr = self.conv_r(x)
qi = self.conv_i(x)
qj = self.conv_j(x)
qk = self.conv_k(x)
return qr, qi, qj, qk
```
3. 创建四元数卷积层实例
使用上述定义的四元数卷积层,创建一个四元数卷积层实例。
```python
quaternion_conv = QuaternionConv2d(in_channels=3, out_channels=8, kernel_size=3, stride=1, padding=1)
```
4. 进行卷积操作
将输入数据传递给四元数卷积层实例进行卷积运算。
```python
input = torch.randn(1, 3, 32, 32)
output_r, output_i, output_j, output_k = quaternion_conv(input)
```
以上就是使用PyTorch实现四元数卷积的简单示例。需要注意的是,四元数卷积在实际应用中可能会有更加复杂的结构和参数设置,以上示例仅为简单的展示。在实际应用中,可能需要根据具体的需求进行更多的调整和优化。
### 回答3:
四元数卷积是一种在神经网络中使用四元数进行卷积操作的技术。在使用pytorch编写四元数卷积时,可以按照以下步骤进行:
1. 首先,导入所需的pytorch库和其他依赖项。这包括torch、torch.nn和其他需要使用的库。
2. 定义一个继承自torch.nn.Module的FourQuaternionConvolution类,这个类将实现四元数卷积操作。
3. 在构造函数中,定义四元数卷积的输入和输出通道数,以及其他必要的参数。同时,创建四元数卷积操作所需的权重和偏置。
4. 在forward方法中,实现四元数卷积的具体操作。首先,将输入张量(四元数)分割为实部和虚部,然后使用torch.nn.functional中的conv2d函数对实部和虚部进行卷积操作。最后,将两个卷积结果按照四元数的形式重新组合起来作为输出。
5. 对于反向传播,pytorch会自动计算梯度并更新权重和偏置,无需手动实现。
6. 最后,可以使用创建的FourQuaternionConvolution类来定义自己的神经网络,并在训练过程中使用四元数卷积层。
总之,使用pytorch编写四元数卷积可以通过定义一个四元数卷积类,实现forward方法以及其他必要的操作。这样,就可以在神经网络中使用四元数的卷积操作进行训练和推理。
相关推荐
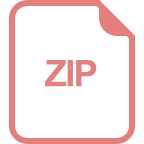
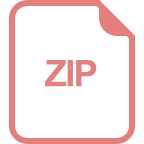



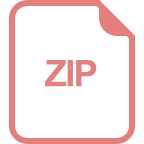
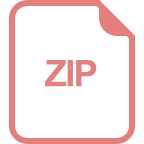
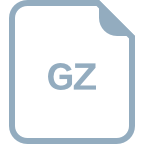
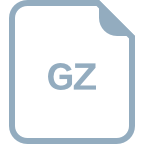
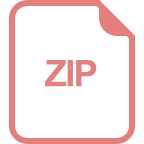
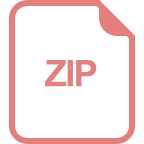