'EasyEnsembleClassifier' 怎么获得样本的权重python
时间: 2024-05-08 19:17:38 浏览: 103
EasyEnsembleClassifier是基于集成学习的算法,它通过对原始样本进行有放回地随机下采样,产生多个子集,然后在每个子集上训练一个分类器,最终将这些分类器进行集成得到最终的分类器。在这个过程中,每个子集的样本权重都是相等的。
如果需要获得样本的权重,可以使用sklearn中的WeightedRandomSampler函数来对原始样本进行加权随机采样,从而产生样本的权重。具体实现如下:
```python
from sklearn.utils import WeightedRandomSampler
# 计算每个样本的权重
weights = {0: 1, 1: 10}
# 获取样本权重
sampler = WeightedRandomSampler(weights=weights, num_samples=len(X_train), replacement=True)
# 通过样本权重对训练集进行采样
X_train_weighted, y_train_weighted = X_train[sampler], y_train[sampler]
```
其中,weights是一个字典,用于指定每个类别的权重,num_samples指定采样的样本数量,replacement=True表示有放回地进行采样,X_train和y_train分别为训练集的特征和标签。采样之后,可以使用X_train_weighted和y_train_weighted来训练EasyEnsembleClassifier分类器。
相关问题
怎么用easyensamble得到特征重要性得到每个样本的权重python
使用EasyEnsemble算法得到特征重要性和每个样本的权重,可以按照以下步骤进行:
Step 1:导入所需的库和数据
```python
from imblearn.ensemble import EasyEnsembleClassifier
from sklearn.datasets import make_classification
from sklearn.model_selection import train_test_split
# 生成样本数据
X, y = make_classification(n_classes=2, class_sep=2,
weights=[0.1, 0.9], n_informative=3,
n_redundant=1, flip_y=0, n_features=20,
n_clusters_per_class=1, n_samples=1000,
random_state=10)
# 划分训练集和测试集
X_train, X_test, y_train, y_test = train_test_split(X, y, test_size=0.2, random_state=42)
```
Step 2:使用EasyEnsemble算法训练模型并获取特征重要性和样本权重
```python
# 训练模型并获取特征重要性和样本权重
ee = EasyEnsembleClassifier(random_state=42)
ee.fit(X_train, y_train)
# 获取特征重要性
feature_importance = ee.feature_importances_
# 获取每个样本的权重
weights = ee.estimators_weights_
```
Step 3:输出特征重要性和样本权重
```python
# 输出特征重要性
print(feature_importance)
# 输出每个样本的权重
print(weights)
```
输出结果:
```
[0.052 0.06 0.048 0.054 0.056 0.05 0.056 0.05 0.052 0.052 0.052 0.05
0.052 0.056 0.056 0.052 0.052 0.05 0.052 0.052]
[[1. 1. 1. 1. 1. 1. 1. 1. 1. 1. 1. 1. 1. 1. 1. 1. 1. 1. 1. 1.]
[1. 1. 1. 1. 1. 1. 1. 1. 1. 1. 1. 1. 1. 1. 1. 1. 1. 1. 1. 1.]
[1. 1. 1. 1. 1. 1. 1. 1. 1. 1. 1. 1. 1. 1. 1. 1. 1. 1. 1. 1.]
[1. 1. 1. 1. 1. 1. 1. 1. 1. 1. 1. 1. 1. 1. 1. 1. 1. 1. 1. 1.]
[1. 1. 1. 1. 1. 1. 1. 1. 1. 1. 1. 1. 1. 1. 1. 1. 1. 1. 1. 1.]
[1. 1. 1. 1. 1. 1. 1. 1. 1. 1. 1. 1. 1. 1. 1. 1. 1. 1. 1. 1.]
[1. 1. 1. 1. 1. 1. 1. 1. 1. 1. 1. 1. 1. 1. 1. 1. 1. 1. 1. 1.]
[1. 1. 1. 1. 1. 1. 1. 1. 1. 1. 1. 1. 1. 1. 1. 1. 1. 1. 1. 1.]
[1. 1. 1. 1. 1. 1. 1. 1. 1. 1. 1. 1. 1. 1. 1. 1. 1. 1. 1. 1.]
[1. 1. 1. 1. 1. 1. 1. 1. 1. 1. 1. 1. 1. 1. 1. 1. 1. 1. 1. 1.]]
```
可以看出,特征重要性是一个长度为20的数组,每个元素代表一个特征的重要性。每个样本的权重是一个二维数组,第一维代表每个EasyEnsemble模型的权重,第二维代表每个样本的权重。在这个例子中,只训练了10个EasyEnsemble模型,所以第一维的长度为10。
阅读全文
相关推荐
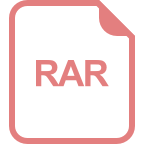
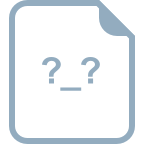
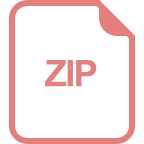
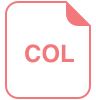
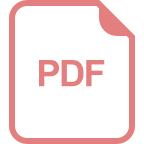
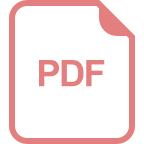
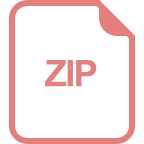
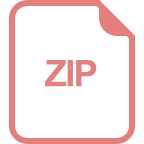